Augmented Lagrangian is Enough for Optimal Offline RL with General Function Approximation and Partial Coverage
ICLR 2023(2023)
摘要
Offline reinforcement learning (RL), which refers to decision-making from a previously-collected dataset of interactions, has received significant attention over the past years. Much effort has focused on improving offline RL practicality by addressing the prevalent issue of partial data coverage through various forms of conservative policy learning. While the majority of algorithms do not have finite-sample guarantees, several provable conservative offline RL algorithms are designed and analyzed within the single-policy concentrability framework that handles partial coverage. Yet, in the nonlinear function approximation setting where confidence intervals are difficult to obtain, existing provable algorithms suffer from computational intractability, prohibitively strong assumptions, and suboptimal statistical rates. In this paper, we leverage the marginalized importance sampling (MIS) formulation of RL and present the first set of offline RL algorithms that are statistically optimal and practical under general function approximation and single-policy concentrability, bypassing the need for uncertainty quantification. We identify that the key for successfully solving the sample-based approximation of the MIS problem is ensuring that certain state occupancy validity constraints are nearly satisfied. We enforce these constraints by a novel application of the augmented Lagrangian method and prove the following result: with MIS formulation, augmented Lagrangian is enough for statistically optimal offline RL. In stark contrast to prior algorithms that induce additional conservatism through methods such as behavior regularization, our approach provably eliminates this need and reinterprets regularizers as "enforcers of state occupancy validity" than "promoters of conservatism."
更多查看译文
关键词
Offline RL,Pessimism,RL Theory
AI 理解论文
溯源树
样例
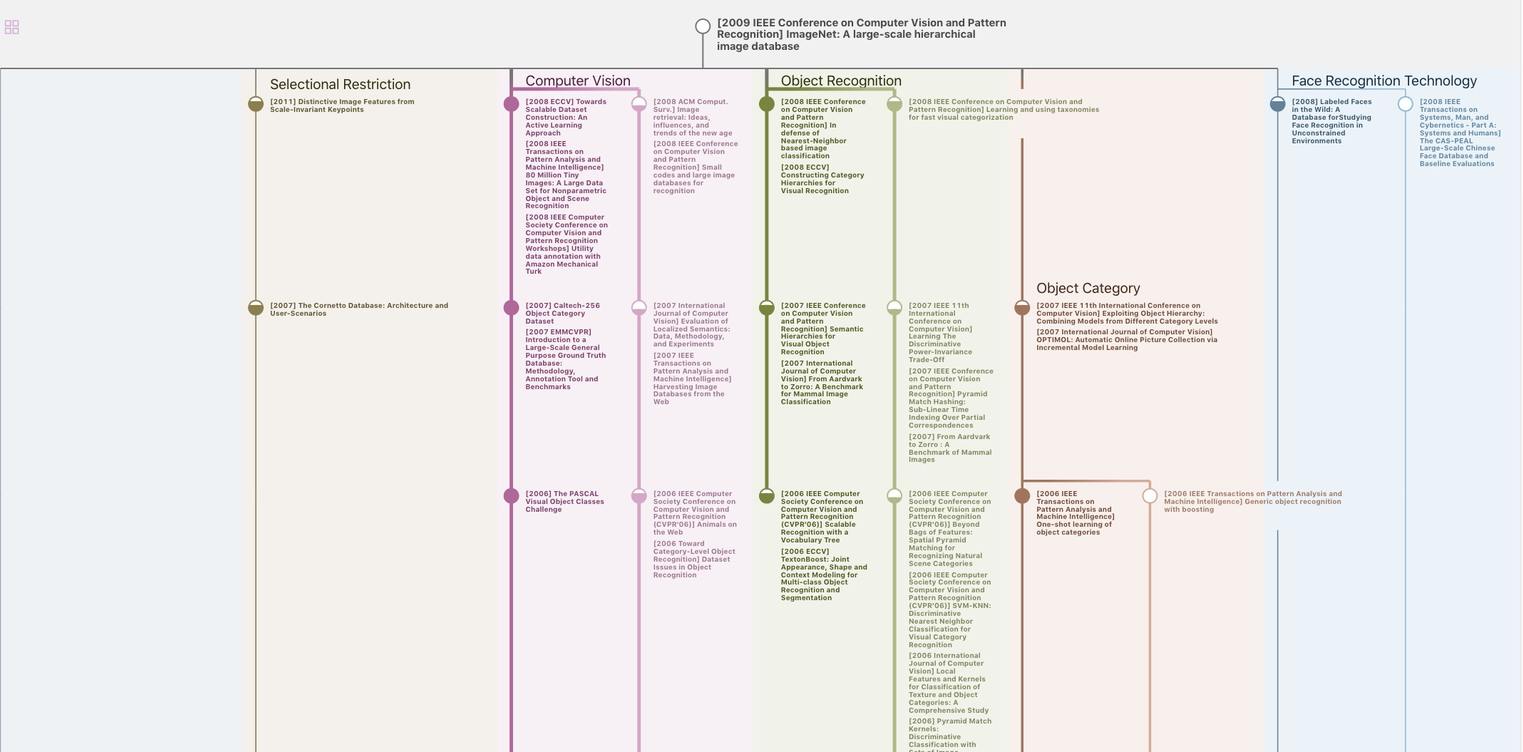
生成溯源树,研究论文发展脉络
Chat Paper
正在生成论文摘要