Learning About Progress From Experts
ICLR 2023(2023)
摘要
Many important tasks involve some notion of long-term progress in multiple phases: e.g. to clean a shelf it must be cleared of items, cleaning products applied, and then the items placed back on the shelf. In this work, we explore the use of expert demonstrations in long-horizon tasks to learn a monotonically increasing function that summarizes progress. This function can then be used to aid agent exploration in environments with sparse rewards. As a case study we consider the NetHack environment, which requires long-term progress at a variety of scales and is far from being solved by existing approaches. In this environment, we demonstrate that by learning a model of long-term progress from expert data containing only observations, we can achieve efficient exploration in challenging sparse tasks, well beyond what is possible with current state-of-the-art approaches. We will open-source the curated expert training data at publication time.
更多查看译文
关键词
learning from demonstrations,reinforcement learning,exploration,nethack
AI 理解论文
溯源树
样例
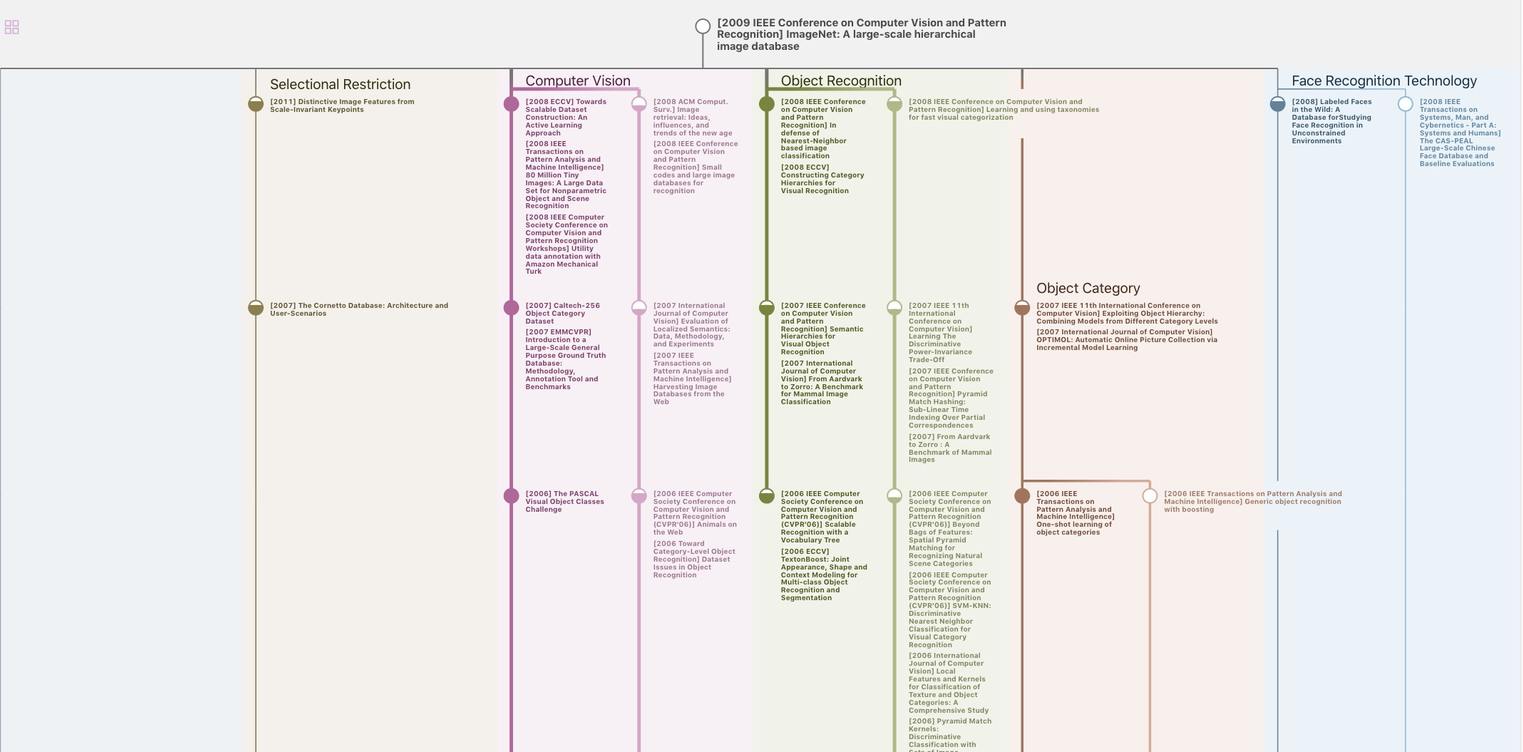
生成溯源树,研究论文发展脉络
Chat Paper
正在生成论文摘要