Associative Memory Augmented Asynchronous Spatiotemporal Representation Learning for Event-based Perception
ICLR 2023(2023)
摘要
We propose $\textit{EventFormer}$, a computationally efficient event-based representation learning framework for asynchronously processing event camera data. EventFormer treats sparse input events as a spatially unordered set and models their spatial interactions using self-attention mechanism. An associative memory-augmented recurrent module is used to correlate with the stored representation computed from past events. A memory addressing mechanism is proposed to store and retrieve the latent states only $\textit{where}$ these events occur and update them only $\textit{when}$ they occur. The representation learning shift from input space to the latent memory space resulting in reduced computation cost for processing each event. We show that EventFormer achieves 0.5$\%$ and 9$\%$ better accuracy with 30000$\times$ and 200$\times$ less computation compared to the state-of-the-art dense and event-based method, respectively, on event-based object recognition datasets.
更多查看译文
关键词
associative memory,memory augmented neural network,spatiotemporal representation,event-based camera,event-based perception,object recognition,attention,set processing
AI 理解论文
溯源树
样例
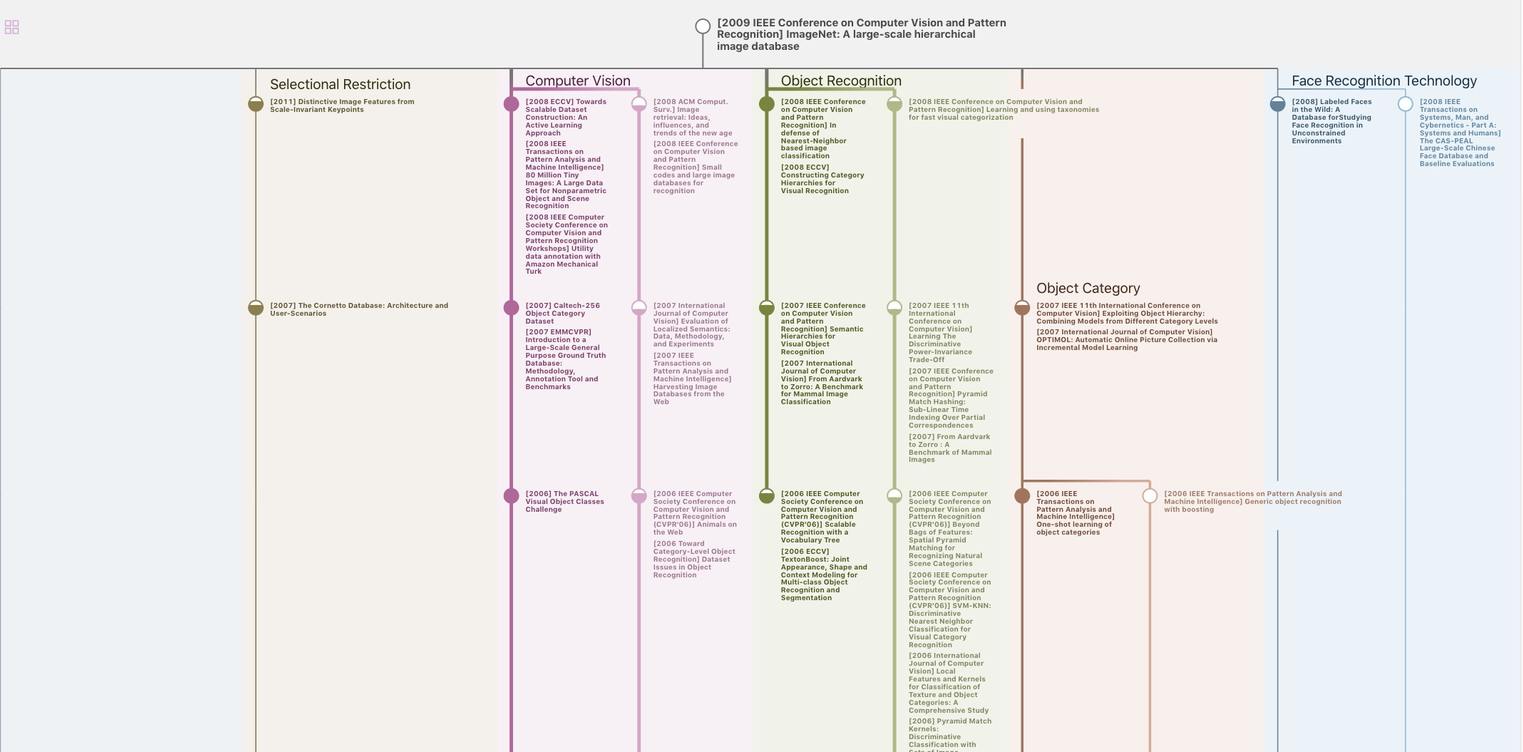
生成溯源树,研究论文发展脉络
Chat Paper
正在生成论文摘要