Mitigating Gradient Bias in Multi-objective Learning: A Provably Convergent Approach
ICLR 2023(2023)
摘要
Many machine learning problems today have multiple objective functions. They appear either in learning with multiple criteria where learning has to make a trade-off between multiple performance metrics such as fairness, safety and accuracy; or, in multi-task learning where multiple tasks are optimized jointly, sharing inductive bias between them. This problems are often tackled by the multi-objective optimization framework. However, existing stochastic multi-objective gradient methods and its variants (e.g., MGDA, PCGrad, CAGrad, etc.) all adopt a biased noisy gradient direction, which leads to degraded empirical performance.
To this end, we develop a stochastic multi-objective gradient correction (MoCo) method for multi-objective optimization. The unique feature of our method is that it can guarantee convergence without increasing the batch size even in the nonconvex setting. Simulations on multi-task supervised and reinforcement learning demonstrate the effectiveness of our method relative to the state-of-the-art methods.
更多查看译文
关键词
Multi-objective Optimization,Machine Learning
AI 理解论文
溯源树
样例
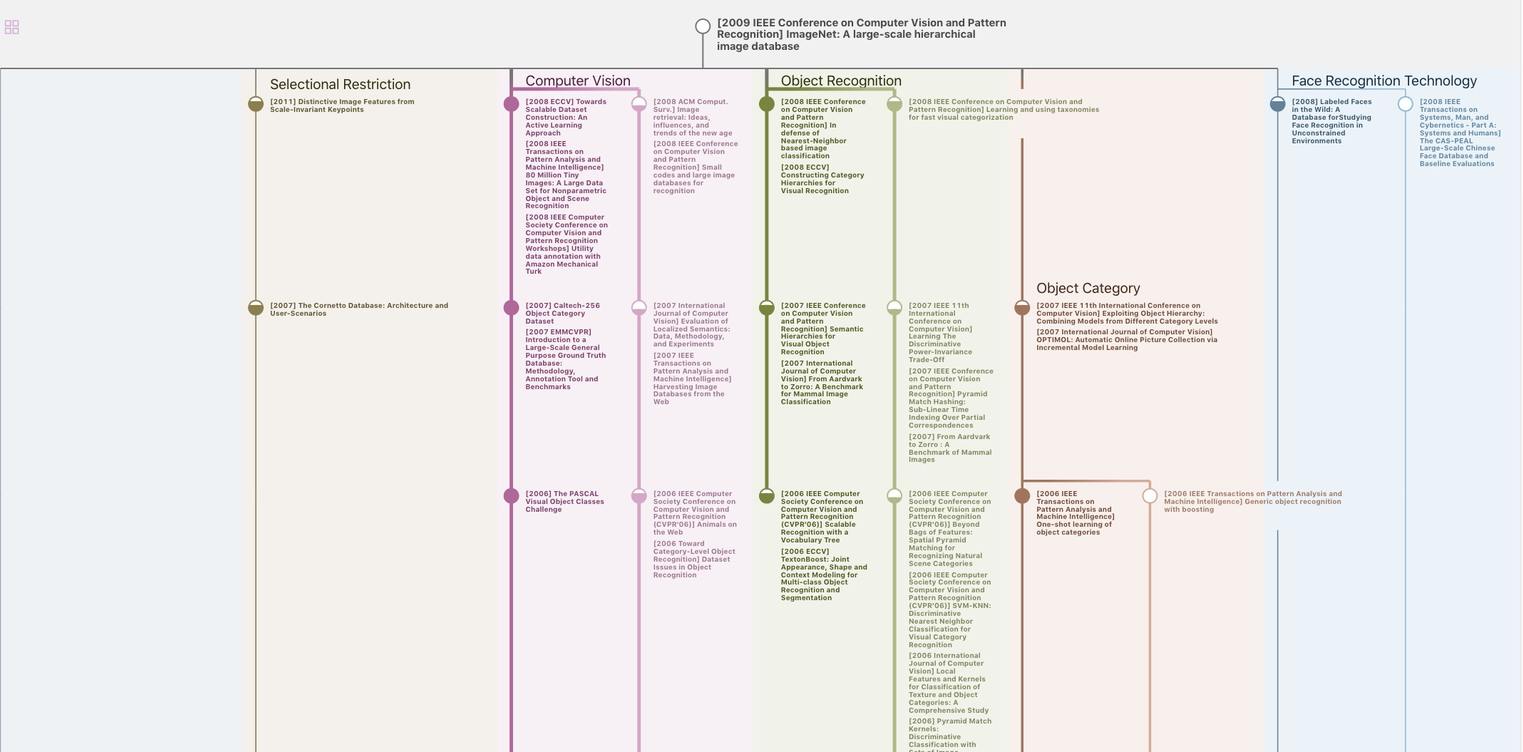
生成溯源树,研究论文发展脉络
Chat Paper
正在生成论文摘要