PASSerRank: Prediction of Allosteric Sites with Learning to Rank
ArXiv(2023)
摘要
Allostery plays a crucial role in regulating protein activity, making it a highly sought-after target in drug development. One of the major challenges in allosteric drug research is the identification of allosteric sites, which is a fundamental aspect of the field. In recent years, many computational models have been developed for accurate allosteric site prediction. However, most of these models focus on designing a general rule that can be applied to pockets of proteins from various families. In this study, we present a new approach to this task using the concept of Learning to Rank (LTR). Our LTR model ranks pockets of each protein based on their relevance as allosteric sites. The model outperforms other common machine learning models, with a higher F1 score and Matthews correlation coefficient. After training and validation on two datasets, the Allosteric Database (ASD) and CASBench, the LTR model was able to rank an allosteric pocket in the top 3 positions for 83.6% and 80.5% of test proteins, respectively. The trained model is available for free on the PASSer platform (https://passer.smu.edu) to aid in drug discovery research.
更多查看译文
AI 理解论文
溯源树
样例
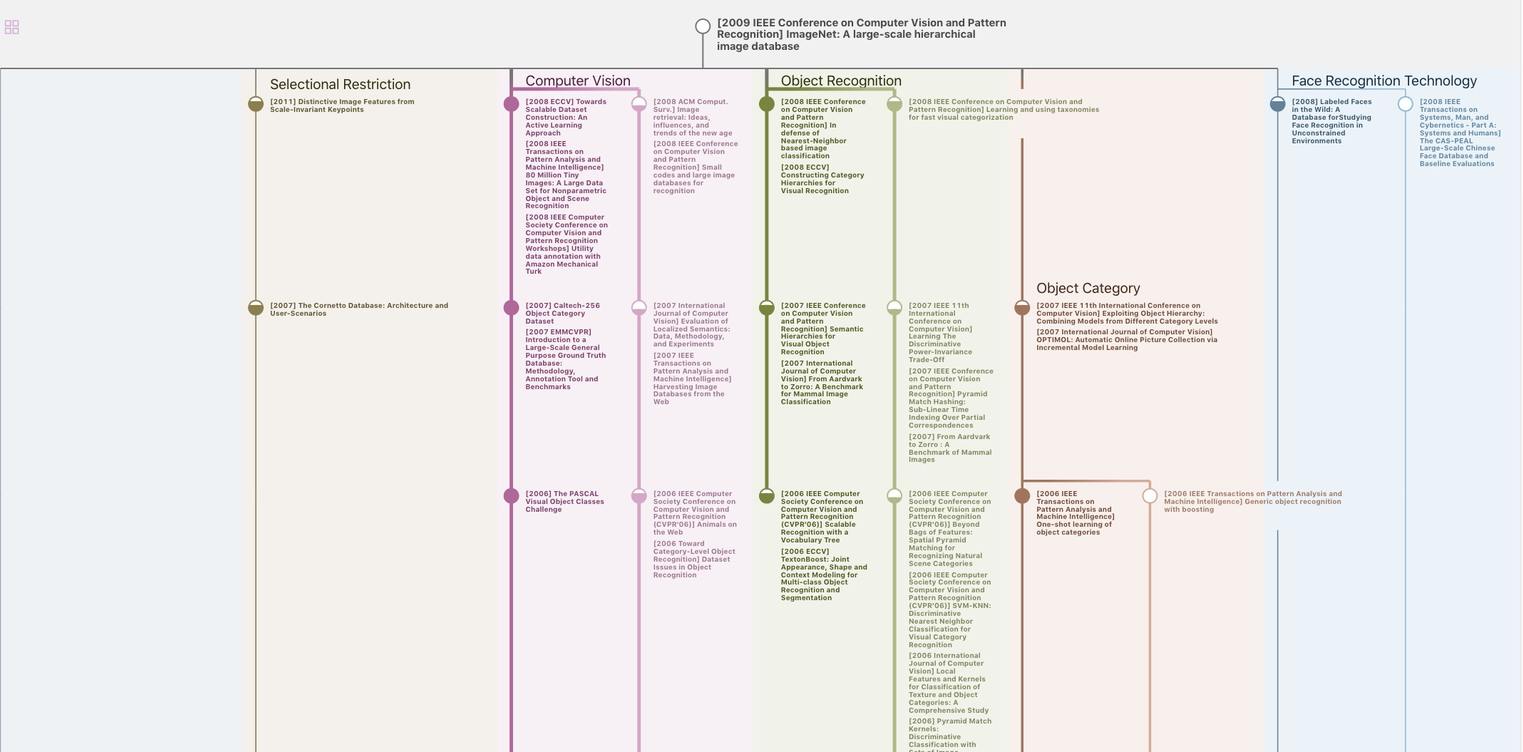
生成溯源树,研究论文发展脉络
Chat Paper
正在生成论文摘要