Quantum Graph Learning: Frontiers and Outlook
arXiv (Cornell University)(2023)
摘要
Quantum theory has shown its superiority in enhancing machine learning. However, facilitating quantum theory to enhance graph learning is in its infancy. This survey investigates the current advances in quantum graph learning (QGL) from three perspectives, i.e., underlying theories, methods, and prospects. We first look at QGL and discuss the mutualism of quantum theory and graph learning, the specificity of graph-structured data, and the bottleneck of graph learning, respectively. A new taxonomy of QGL is presented, i.e., quantum computing on graphs, quantum graph representation, and quantum circuits for graph neural networks. Pitfall traps are then highlighted and explained. This survey aims to provide a brief but insightful introduction to this emerging field, along with a detailed discussion of frontiers and outlook yet to be investigated.
更多查看译文
关键词
graph learning,quantum,frontiers
AI 理解论文
溯源树
样例
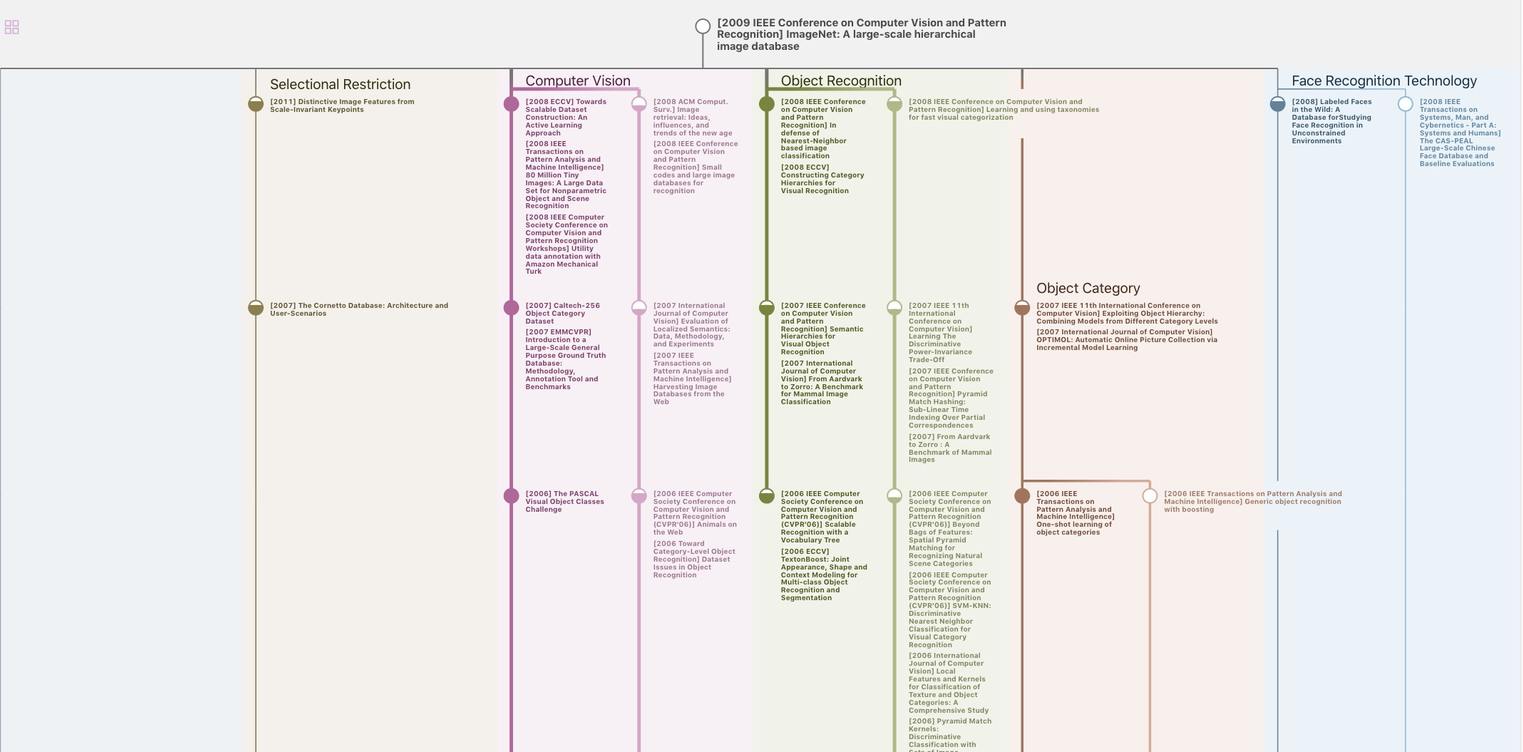
生成溯源树,研究论文发展脉络
Chat Paper
正在生成论文摘要