Causal Effect Estimation: Recent Advances, Challenges, and Opportunities
arxiv(2023)
摘要
Causal inference has numerous real-world applications in many domains, such as health care, marketing, political science, and online advertising. Treatment effect estimation, a fundamental problem in causal inference, has been extensively studied in statistics for decades. However, traditional treatment effect estimation methods may not well handle large-scale and high-dimensional heterogeneous data. In recent years, an emerging research direction has attracted increasing attention in the broad artificial intelligence field, which combines the advantages of traditional treatment effect estimation approaches (e.g., propensity score, matching, and reweighing) and advanced machine learning approaches (e.g., representation learning, adversarial learning, and graph neural networks). Although the advanced machine learning approaches have shown extraordinary performance in treatment effect estimation, it also comes with a lot of new topics and new research questions. In view of the latest research efforts in the causal inference field, we provide a comprehensive discussion of challenges and opportunities for the three core components of the treatment effect estimation task, i.e., treatment, covariates, and outcome. In addition, we showcase the promising research directions of this topic from multiple perspectives.
更多查看译文
关键词
estimation,effect,advances
AI 理解论文
溯源树
样例
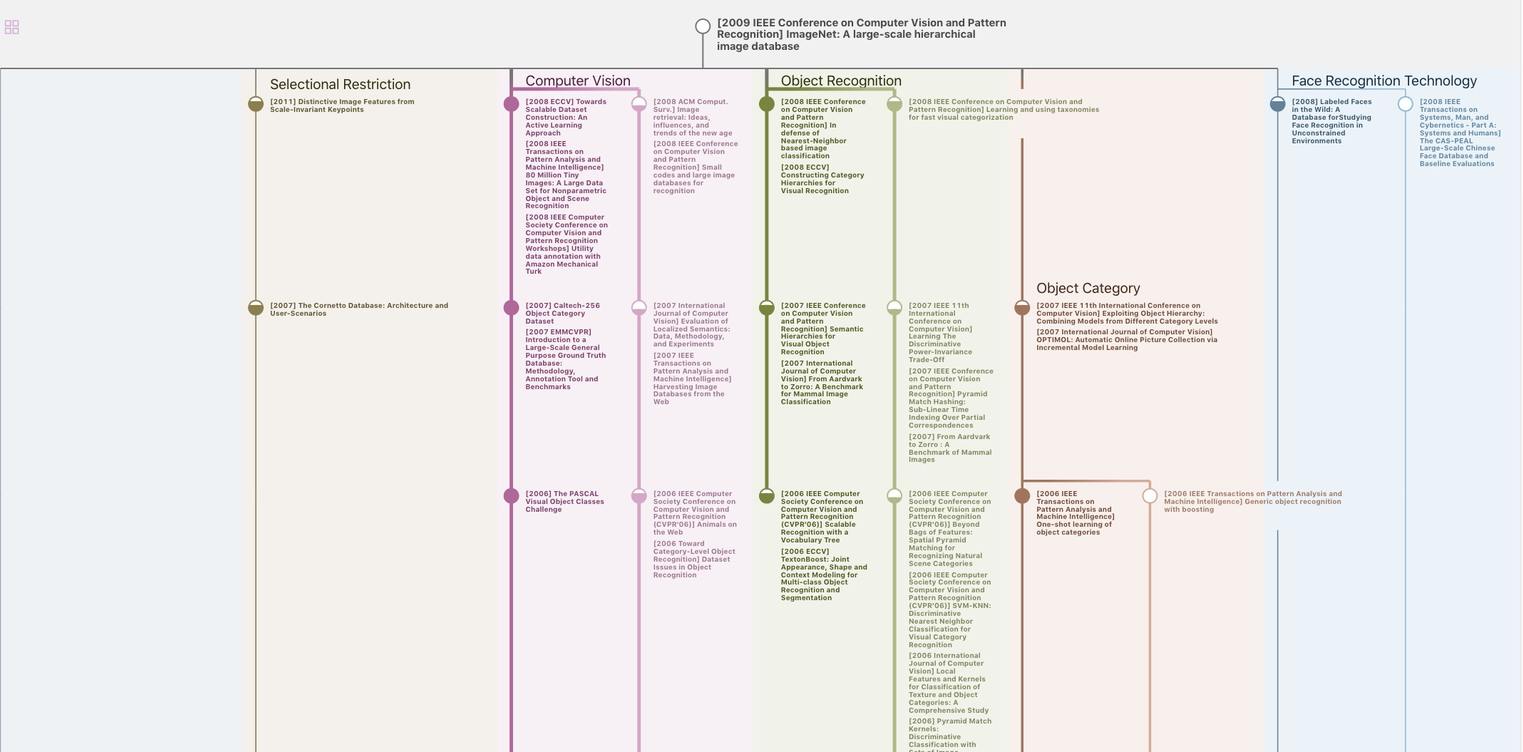
生成溯源树,研究论文发展脉络
Chat Paper
正在生成论文摘要