Average-Constrained Policy Optimization
arxiv(2023)
摘要
Reinforcement Learning (RL) with constraints is becoming an increasingly important problem for various applications. Often, the average criterion is more suitable. Yet, RL for average criterion-constrained MDPs remains a challenging problem. Algorithms designed for discounted constrained RL problems often do not perform well for the average CMDP setting. In this paper, we introduce a new (possibly the first) policy optimization algorithm for constrained MDPs with the average criterion. The Average-Constrained Policy Optimization (ACPO) algorithm is inspired by the famed PPO-type algorithms based on trust region methods. We develop basic sensitivity theory for average MDPs, and then use the corresponding bounds in the design of the algorithm. We provide theoretical guarantees on its performance, and through extensive experimental work in various challenging MuJoCo environments, show the superior performance of the algorithm when compared to other state-of-the-art algorithms adapted for the average CMDP setting.
更多查看译文
关键词
optimization,policy,average-constrained
AI 理解论文
溯源树
样例
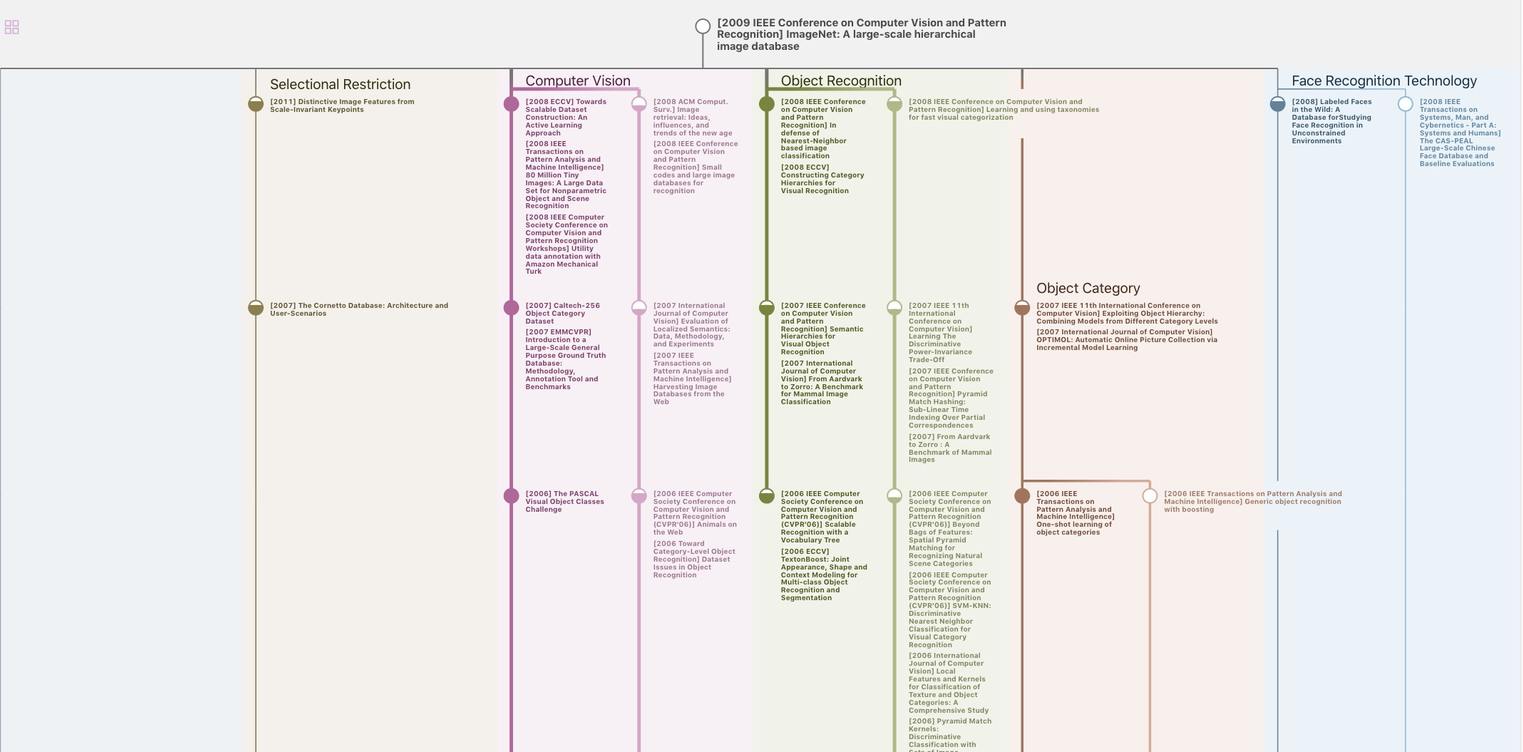
生成溯源树,研究论文发展脉络
Chat Paper
正在生成论文摘要