Learning Representations from Local to Global for Fine-grained Patient Similarity Measuring in Intensive Care Unit
2022 IEEE International Conference on Data Mining (ICDM)(2022)
摘要
Patient similarity measurement is an essential step in discovering clinically meaningful subgroups and building case retrieval systems. Most existing studies implement this procedure using similarity measurement algorithms on the multivariate clinical time-series (input space) or the low-dimensional patient representation (representation space) learned by a representation learning model. However, they either suffer from the adverse effects of irrelevant variables in the data or fail to assess the fine-grained similarity underneath the disease progress. In this paper, we propose a method to measure more fine-grained patient similarity in the state space, where each patient is represented by a series of state representations that reveal the dynamic health status. We discuss three desiderata, including stability, personality, and interpretability, for the state representations, and on this basis, develop a supervised predictive model that learns good state representations for identifying similar patients and predicting patient outcomes. Experimental results on the publicly available dataset MIMIC-III show that our method offers a promising direction for precisely identifying similar patients at the state trajectory level, as well as accurately predicting outcomes.
更多查看译文
关键词
patient similarity,representation learning,deep learning,healthcare
AI 理解论文
溯源树
样例
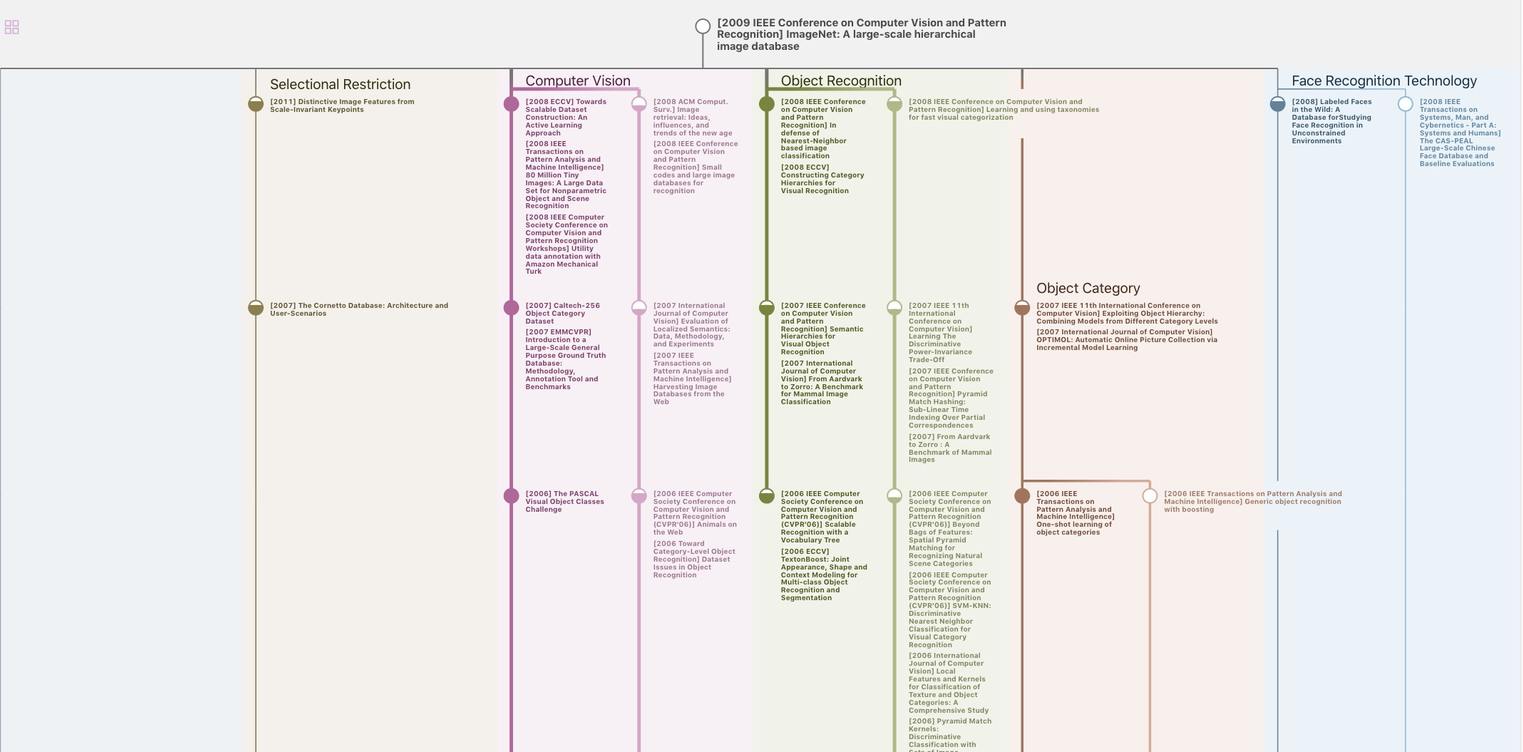
生成溯源树,研究论文发展脉络
Chat Paper
正在生成论文摘要