Efficient Multi-Task Reinforcement Learning via Selective Behavior Sharing
arxiv(2023)
摘要
The ability to leverage shared behaviors between tasks is critical for sample-efficient multi-task reinforcement learning (MTRL). While prior methods have primarily explored parameter and data sharing, direct behavior-sharing has been limited to task families requiring similar behaviors. Our goal is to extend the efficacy of behavior-sharing to more general task families that could require a mix of shareable and conflicting behaviors. Our key insight is an agent's behavior across tasks can be used for mutually beneficial exploration. To this end, we propose a simple MTRL framework for identifying shareable behaviors over tasks and incorporating them to guide exploration. We empirically demonstrate how behavior sharing improves sample efficiency and final performance on manipulation and navigation MTRL tasks and is even complementary to parameter sharing. Result videos are available at https://sites.google.com/view/qmp-mtrl.
更多查看译文
关键词
selective behavior
AI 理解论文
溯源树
样例
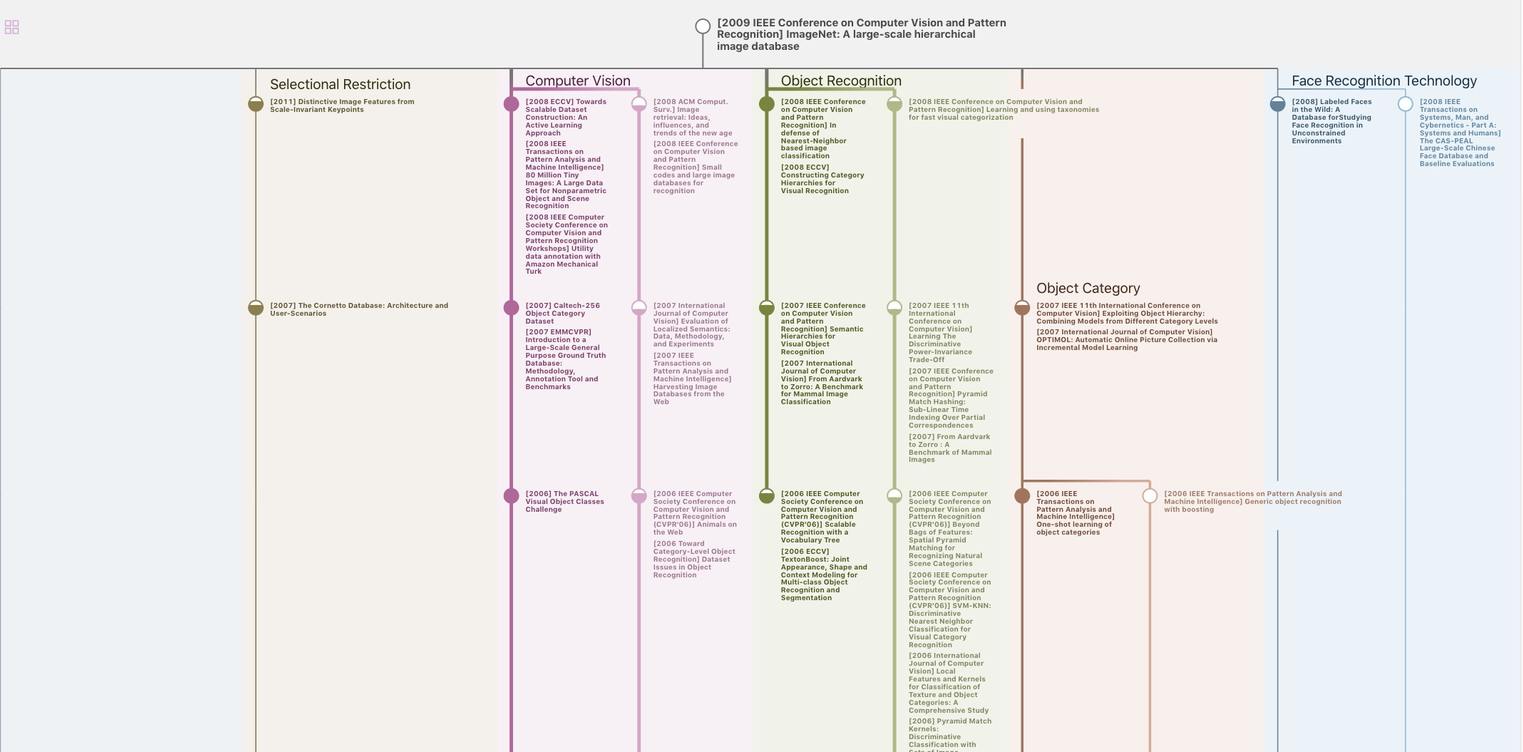
生成溯源树,研究论文发展脉络
Chat Paper
正在生成论文摘要