Time-warped Trials
arxiv(2023)
摘要
I outline a signal resampling strategy for aligning event times between time series trials in contexts where significant event times like onsets and offsets vary between trials. These variations prevent direct comparisons of trials in practical contexts as comparisons require equal-length time series (Salari et al., 2019). Algorithms like dynamic time warping help us quantify these variations locally but do not apply well to continuous transformations of time series signals without interpolating or downsampling to add or remove samples (Jamid, 2004; Eckner, 2014). I show that with consideration for padding and sampling frequency that sinc interpolation is sufficient to resample parts of trial intervals to produce equal-length time-locked trials that correlate to and strongly approximate their unwarped counterparts with minimal interpolation effects. Specifically I show that interpolation effects can be minimized by oversampling, selectively interpolating mis-aligned parts of trials with respect to mean mis-aligned event lengths, and interpolating mis-aligned events with sufficient zero-padding. Interpolated signals then have a bandlimit well below the Nyquist frequency and satisfy the Nyquist-Shannon sampling theorem ensuring perfect reconstructions, and I find that I can track and potentially counteract resampling effects on signal energy quantities.
更多查看译文
关键词
time-warped
AI 理解论文
溯源树
样例
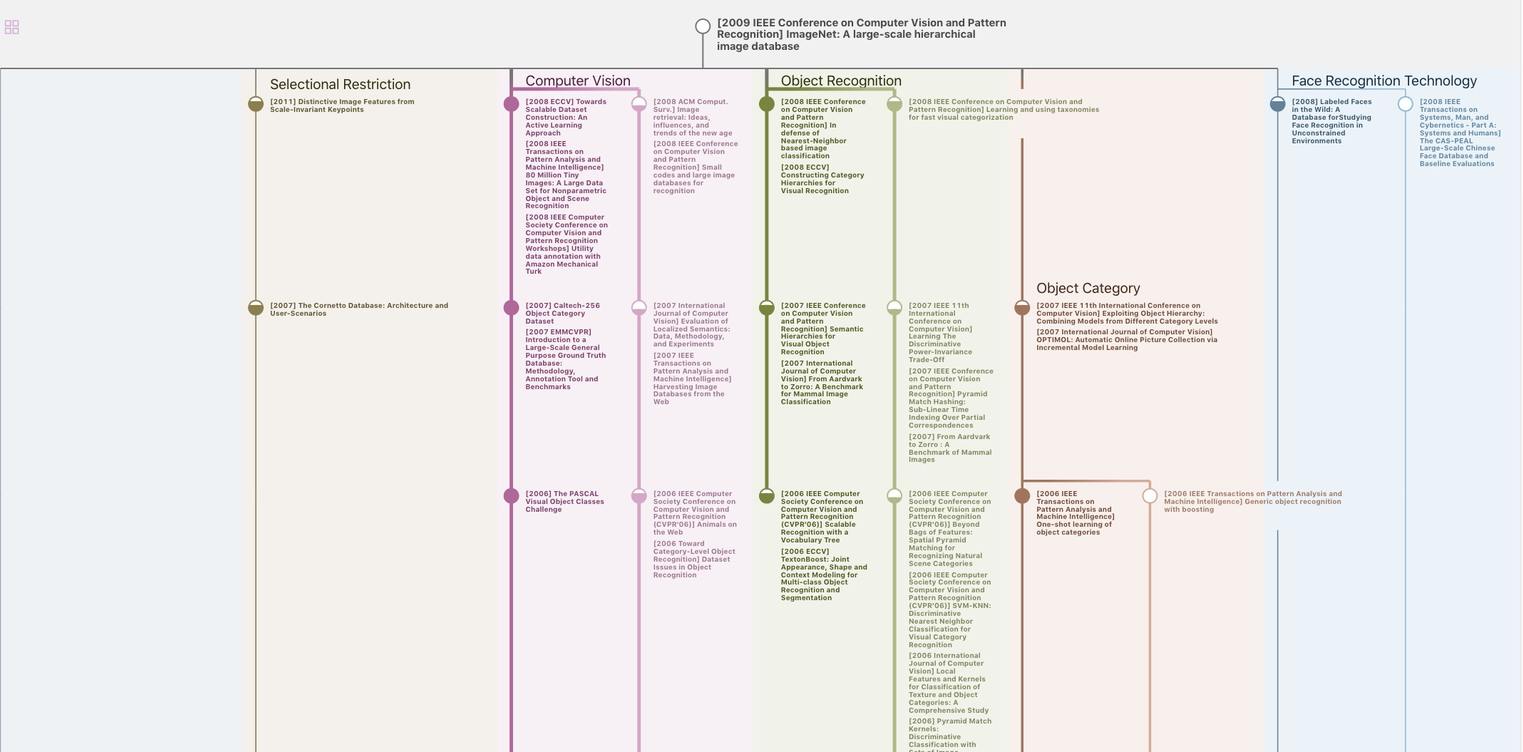
生成溯源树,研究论文发展脉络
Chat Paper
正在生成论文摘要