Free Lunch for Domain Adversarial Training: Environment Label Smoothing
ICLR 2023(2023)
摘要
A fundamental challenge for machine learning models is how to generalize learned models for out-of-distribution (OOD) data. Among various approaches, exploiting invariant features by Domain Adversarial Training (DAT) received widespread attention. Despite its success, we observe training instability from DAT, mostly due to over-confident domain discriminator and environment label noise. To address this issue, we proposed Environment Label Smoothing (ELS), which encourages the discriminator to output soft probability, which thus reduces the confidence of the discriminator and alleviates the impact of noisy environment labels. We demonstrate, both experimentally and theoretically, that ELS can improve training stability, local convergence, and robustness to noisy environment labels. By incorporating ELS with DAT methods, we are able to yield state-of-art results on a wide range of domain generalization/adaptation tasks, particularly when the environment labels are highly noisy.
更多查看译文
关键词
Out-of-Distribution Generalization,Domain adaptation/generalization,Domain adversarial training,environmnt label noise,non-asymptotic convergence
AI 理解论文
溯源树
样例
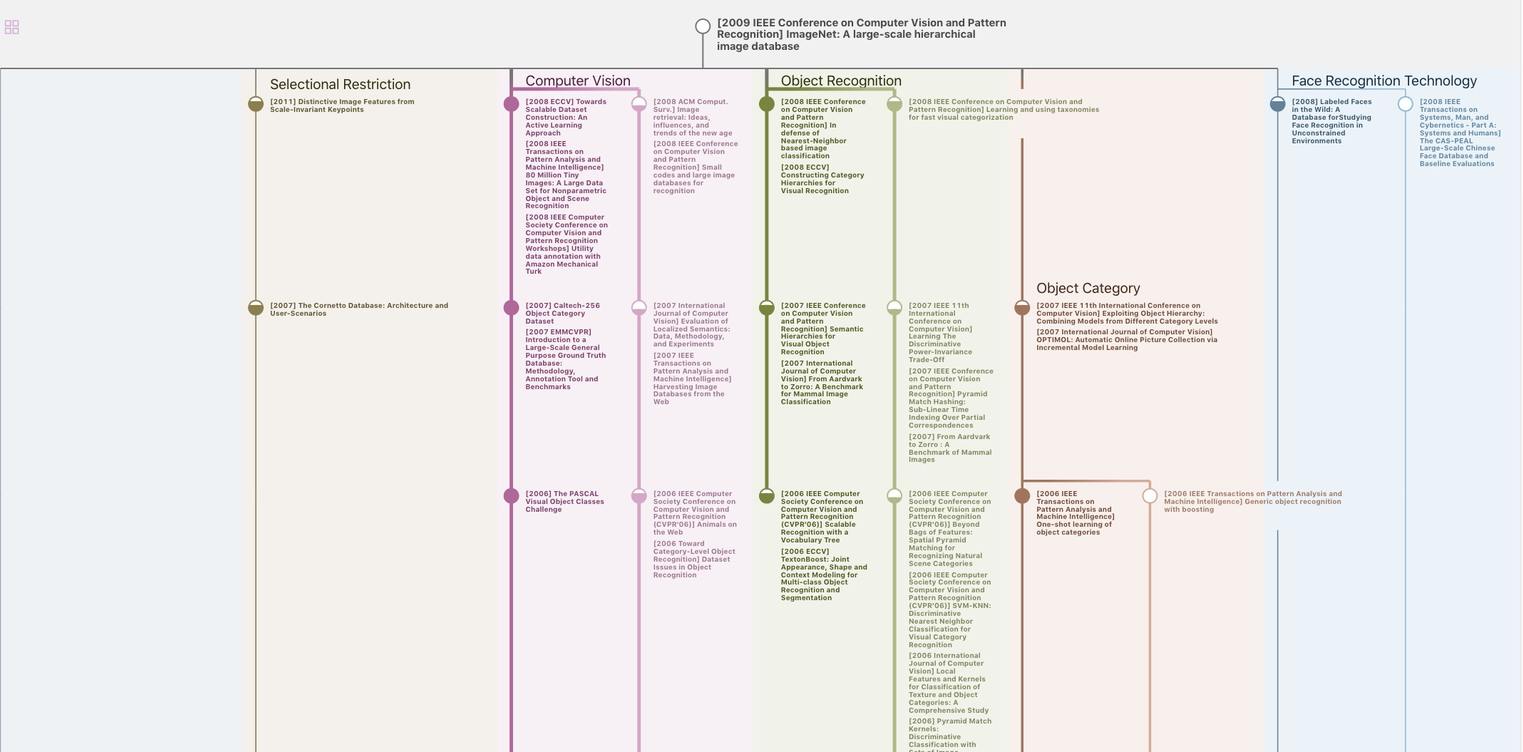
生成溯源树,研究论文发展脉络
Chat Paper
正在生成论文摘要