Deep Active Learning for Scientific Computing in the Wild
CoRR(2023)
摘要
Deep learning (DL) is revolutionizing the scientific computing community. To reduce the data gap caused by usually expensive simulations or experimentation, active learning has been identified as a promising solution for the scientific computing community. However, the deep active learning (DAL) literature is currently dominated by image classification problems and pool-based methods, which are not directly transferrable to scientific computing problems, dominated by regression problems with no pre-defined 'pool' of unlabeled data. Here for the first time, we investigate the robustness of DAL methods for scientific computing problems using ten state-of-the-art DAL methods and eight benchmark problems. We show that, to our surprise, the majority of the DAL methods are not robust even compared to random sampling when the ideal pool size is unknown. We further analyze the effectiveness and robustness of DAL methods and suggest that diversity is necessary for a robust DAL for scientific computing problems.
更多查看译文
关键词
scientific computing,wild,learning,deep
AI 理解论文
溯源树
样例
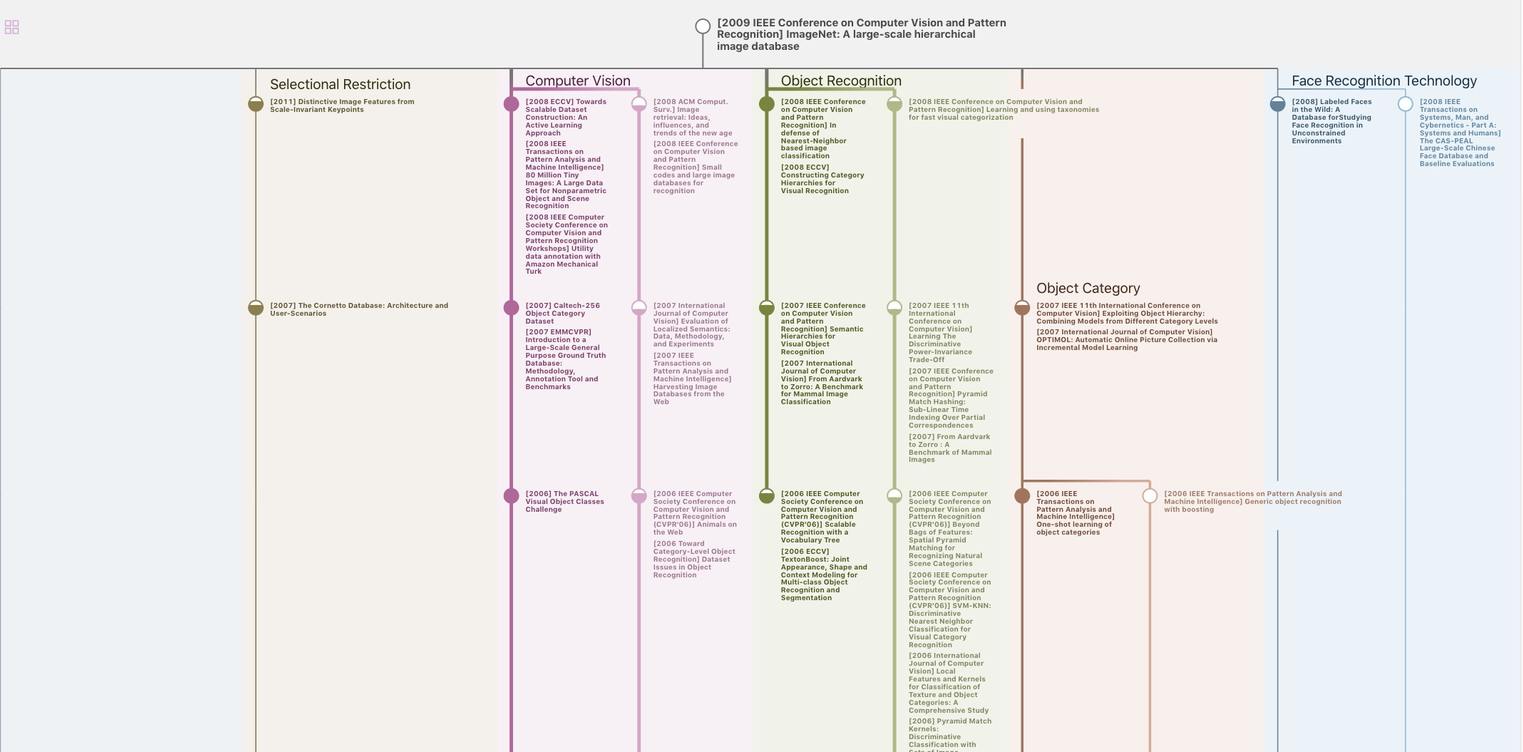
生成溯源树,研究论文发展脉络
Chat Paper
正在生成论文摘要