Gaussian Noise is Nearly Instance Optimal for Private Unbiased Mean Estimation
arxiv(2023)
摘要
We investigate unbiased high-dimensional mean estimators in differential privacy. We consider differentially private mechanisms whose expected output equals the mean of the input dataset, for every dataset drawn from a fixed convex domain $K$ in $\mathbb{R}^d$. In the setting of concentrated differential privacy, we show that, for every input such an unbiased mean estimator introduces approximately at least as much error as a mechanism that adds Gaussian noise with a carefully chosen covariance. This is true when the error is measured with respect to $\ell_p$ error for any $p \ge 2$. We extend this result to local differential privacy, and to approximate differential privacy, but for the latter the error lower bound holds either for a dataset or for a neighboring dataset. We also extend our results to mechanisms that take i.i.d.~samples from a distribution over $K$ and are unbiased with respect to the mean of the distribution.
更多查看译文
关键词
private unbiased mean,gaussian noise,estimation,instance optimal
AI 理解论文
溯源树
样例
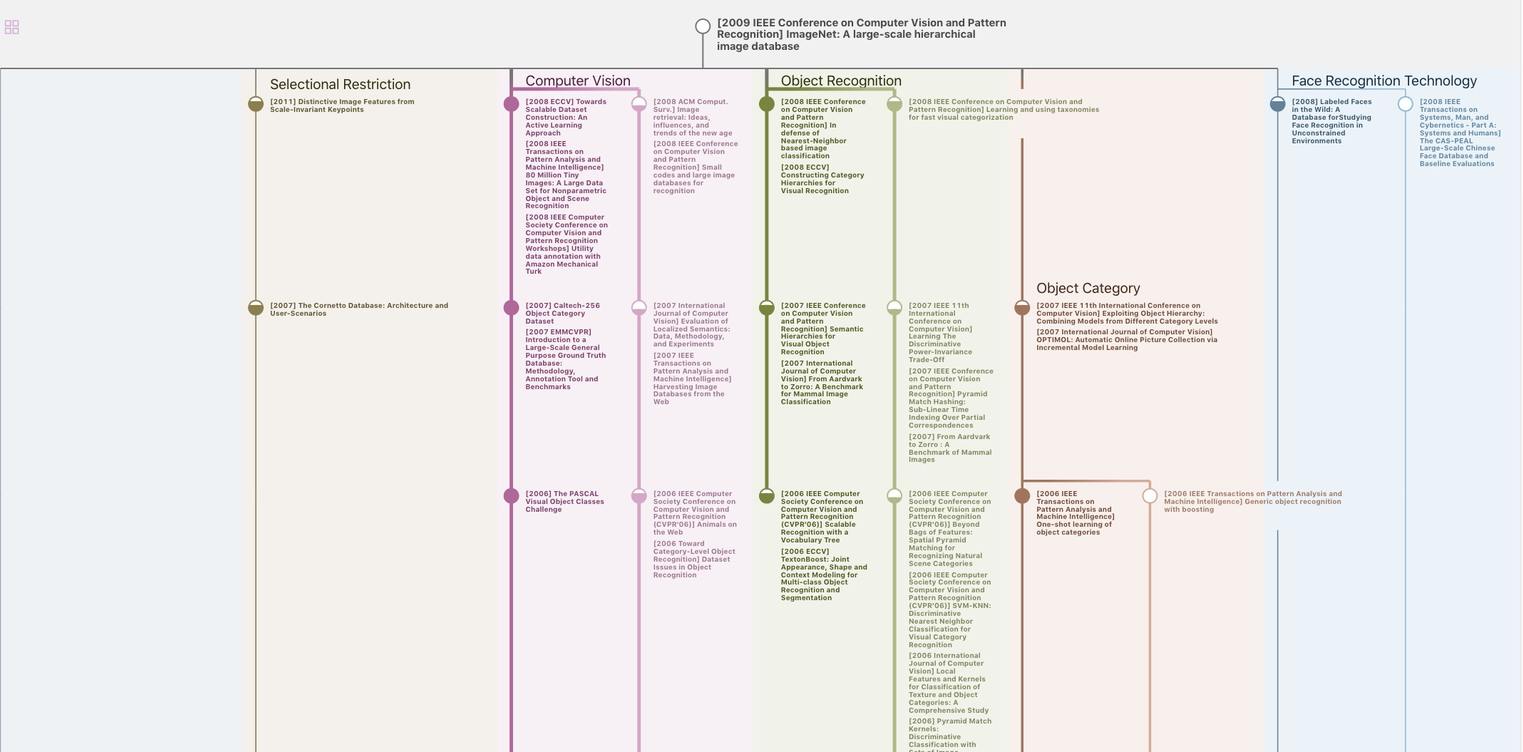
生成溯源树,研究论文发展脉络
Chat Paper
正在生成论文摘要