Low Complexity Adaptive Machine Learning Approaches for End-to-End Latency Prediction
CoRR(2023)
Abstract
Software Defined Networks have opened the door to statistical and AI-based techniques to improve efficiency of networking. Especially to ensure a certain Quality of Service (QoS) for specific applications by routing packets with awareness on content nature (VoIP, video, files, etc.) and its needs (latency, bandwidth, etc.) to use efficiently resources of a network. Monitoring and predicting various Key Performance Indicators (KPIs) at any level may handle such problems while preserving network bandwidth. The question addressed in this work is the design of efficient, low-cost adaptive algorithms for KPI estimation, monitoring and prediction. We focus on end-to-end latency prediction, for which we illustrate our approaches and results on data obtained from a public generator provided after the recent international challenge on GNN [12]. In this paper, we improve our previously proposed low-cost estimators [6] by adding the adaptive dimension, and show that the performances are minimally modified while gaining the ability to track varying networks.
MoreTranslated text
Key words
machine learning,prediction,adaptive,complexity,end-to-end
AI Read Science
Must-Reading Tree
Example
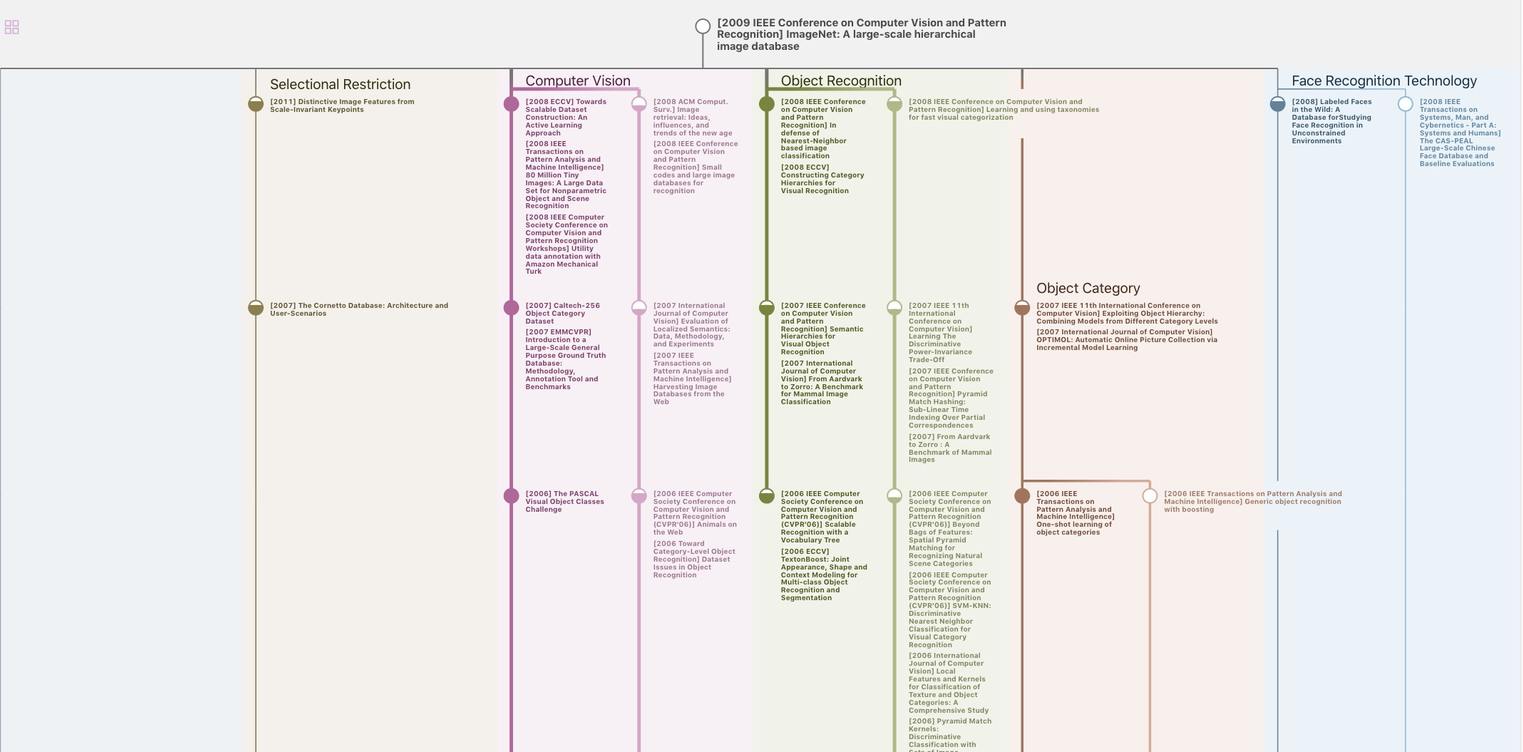
Generate MRT to find the research sequence of this paper
Chat Paper
Summary is being generated by the instructions you defined