Privacy Preserving Ultra-Short-term Wind Power Prediction Based on Secure Multi Party Computation
arxiv(2023)
摘要
Mining the spatial and temporal correlation of wind farm output data is beneficial for enhancing the precision of ultra-short-term wind power prediction. However, if the wind farms are owned by separate entities, they may be reluctant to share their data directly due to privacy concerns as well as business management regulation policies. Although cryptographic approaches have been designed to protect privacy in the process of data sharing, it is still a challenging problem to encrypt the original data while extracting the nonlinear relationship among multiple wind farms in the machine learning process. This paper presents pwXGBoost, a technique based on the machine learning tree model and secure multi-party computation (SMPC) that can successfully extract complicated relationships while preserving data privacy. A maximum mean discrepancy (MMD) based scheme is proposed to effectively choose adjacent candidate wind farms to participate in the collaborative model training, therefore improving the accuracy and reducing the burden of data acquisition. The proposed method was evaluated on real world data collected from a cluster of wind farms in Inner Mongolia, China, demonstrating that it is capable of achieving considerable efficiency and performance improvements while preserving privacy
更多查看译文
关键词
privacy preserving,secure,ultra-short-term
AI 理解论文
溯源树
样例
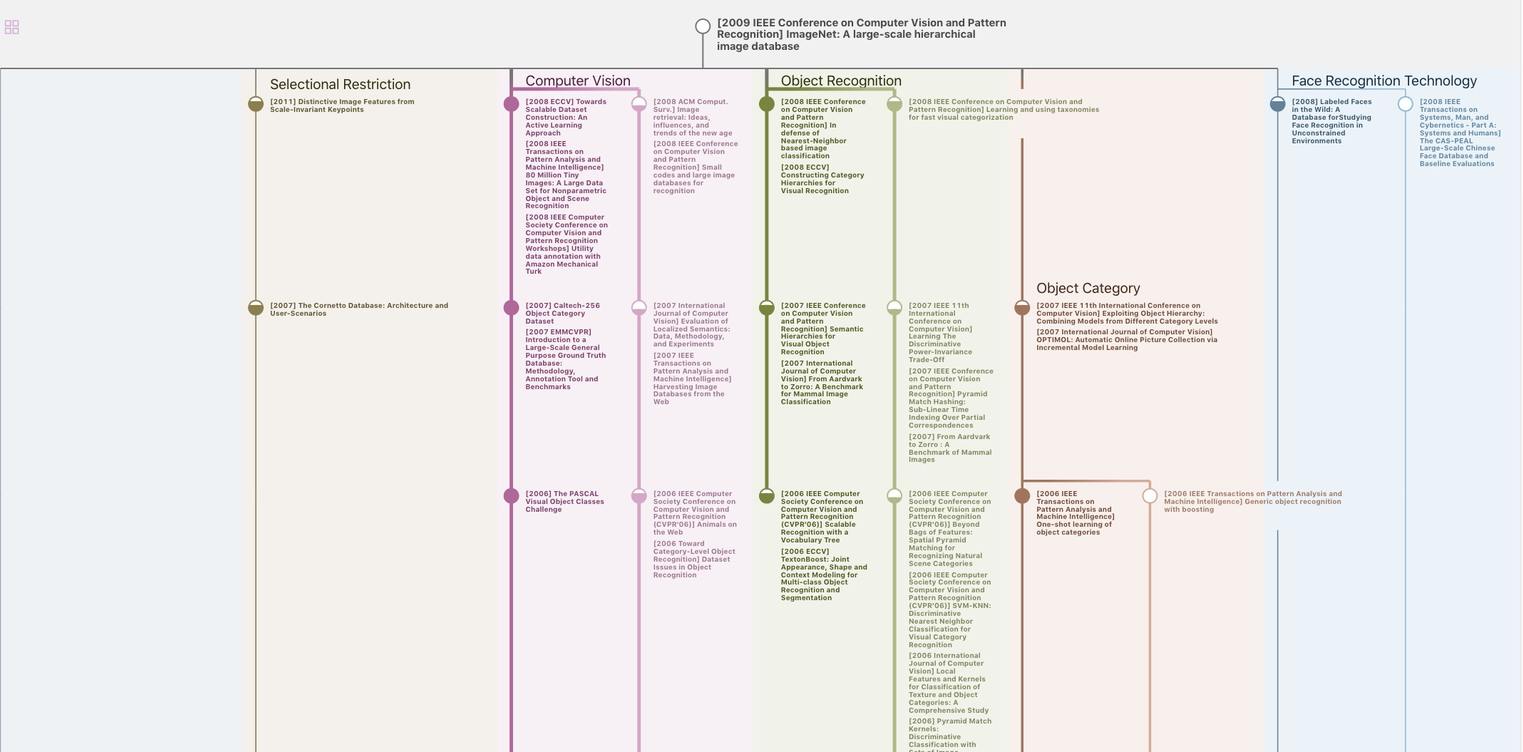
生成溯源树,研究论文发展脉络
Chat Paper
正在生成论文摘要