Optimizing DDPM Sampling with Shortcut Fine-Tuning
arXiv (Cornell University)(2023)
Abstract
In this study, we propose Shortcut Fine-Tuning (SFT), a new approach for addressing the challenge of fast sampling of pretrained Denoising Diffusion Probabilistic Models (DDPMs). SFT advocates for the fine-tuning of DDPM samplers through the direct minimization of Integral Probability Metrics (IPM), instead of learning the backward diffusion process. This enables samplers to discover an alternative and more efficient sampling shortcut, deviating from the backward diffusion process. We also propose a new algorithm that is similar to the policy gradient method for fine-tuning DDPMs by proving that under certain assumptions, the gradient descent of diffusion models is equivalent to the policy gradient approach. Through empirical evaluation, we demonstrate that our fine-tuning method can further enhance existing fast DDPM samplers, resulting in sample quality comparable to or even surpassing that of the full-step model across various datasets.
MoreTranslated text
Key words
ddpm,fine-tuning
AI Read Science
Must-Reading Tree
Example
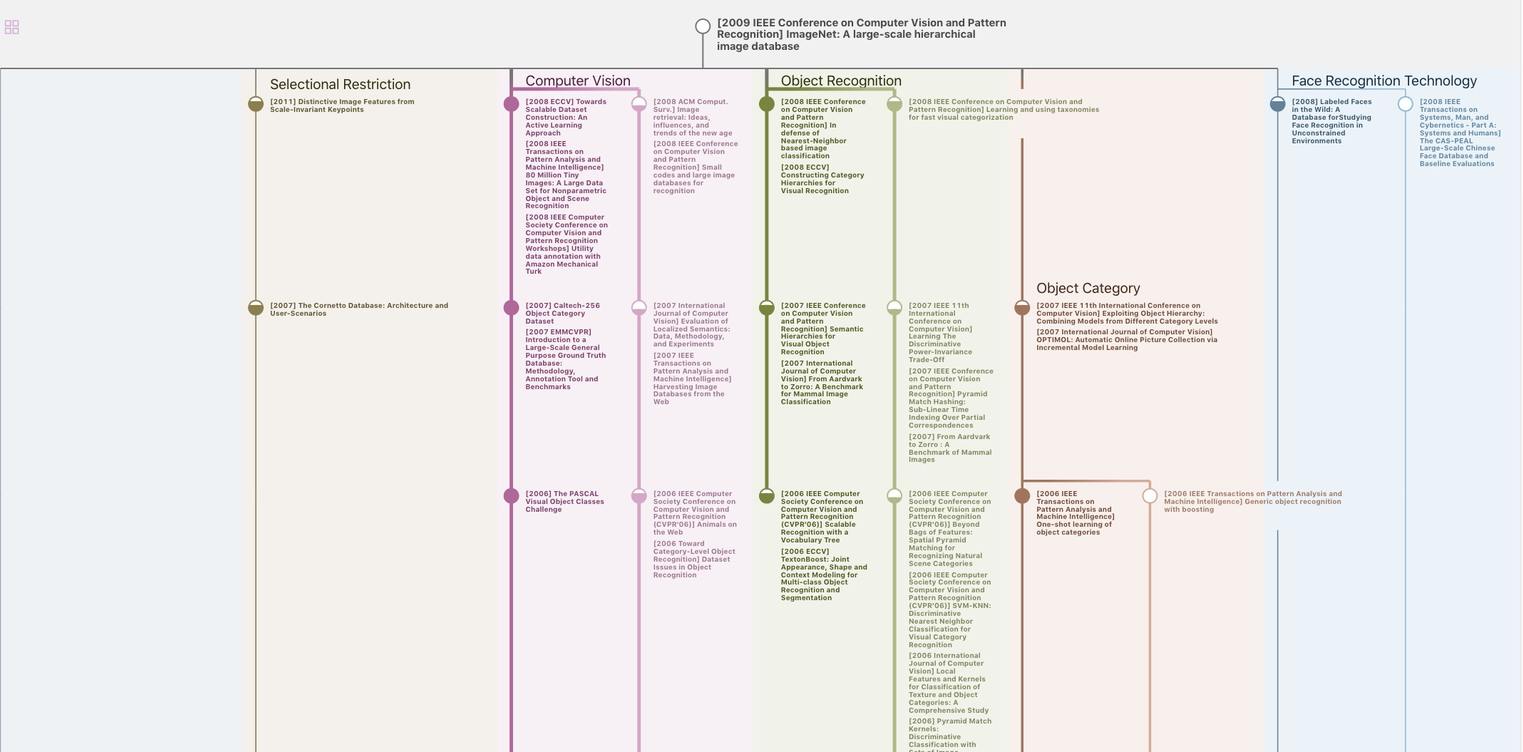
Generate MRT to find the research sequence of this paper
Chat Paper
Summary is being generated by the instructions you defined