Graph Neural Network Framework for Security Assessment Informed by Topological Measures
arXiv (Cornell University)(2023)
Abstract
In the power system, security assessment (SA) plays a pivotal role in determining the safe operation in a normal situation and some contingencies scenarios. Electrical variables as input variables of the model are mainly considered to indicate the power system operation as secure or insecure, according to the reliability criteria for contingency scenarios. In this approach, the features are in grid format data, where the relation between features and any knowledge of network topology is absent. Moreover, the traditional and common models, such as neural networks (NN), are not applicable if the input variables are in the graph format structure. Therefore, this paper examines the security analysis in the graph neural network (GNN) framework such that the GNN model incorporates the network connection and node's neighbors' influence for the assessment. Here the input features are separated graphs representing different network conditions in electrical and structural statuses. Topological characteristics defined by network centrality measures are added in the feature vector representing the structural properties of the network. The proposed model is simulated in the IEEE 118-Bus system for the voltage static security assessment (SSA). The performance indices validate the efficiency of the GNN-based model compared to the traditional NN model denoting that the information enclosed in graph data boosts the classifier performance since the GNN model benefits the neighbors' features. Moreover, outperforming of GNN-based model is determined when robustness and sensitivity analyzes are carried out. The proposed method is not limited to a specific task and can be extended for other security assessments with different critical variables, such as dynamic analysis and frequency criteria, respectively.
MoreTranslated text
Key words
security assessment,topological measures,neural network,graph
AI Read Science
Must-Reading Tree
Example
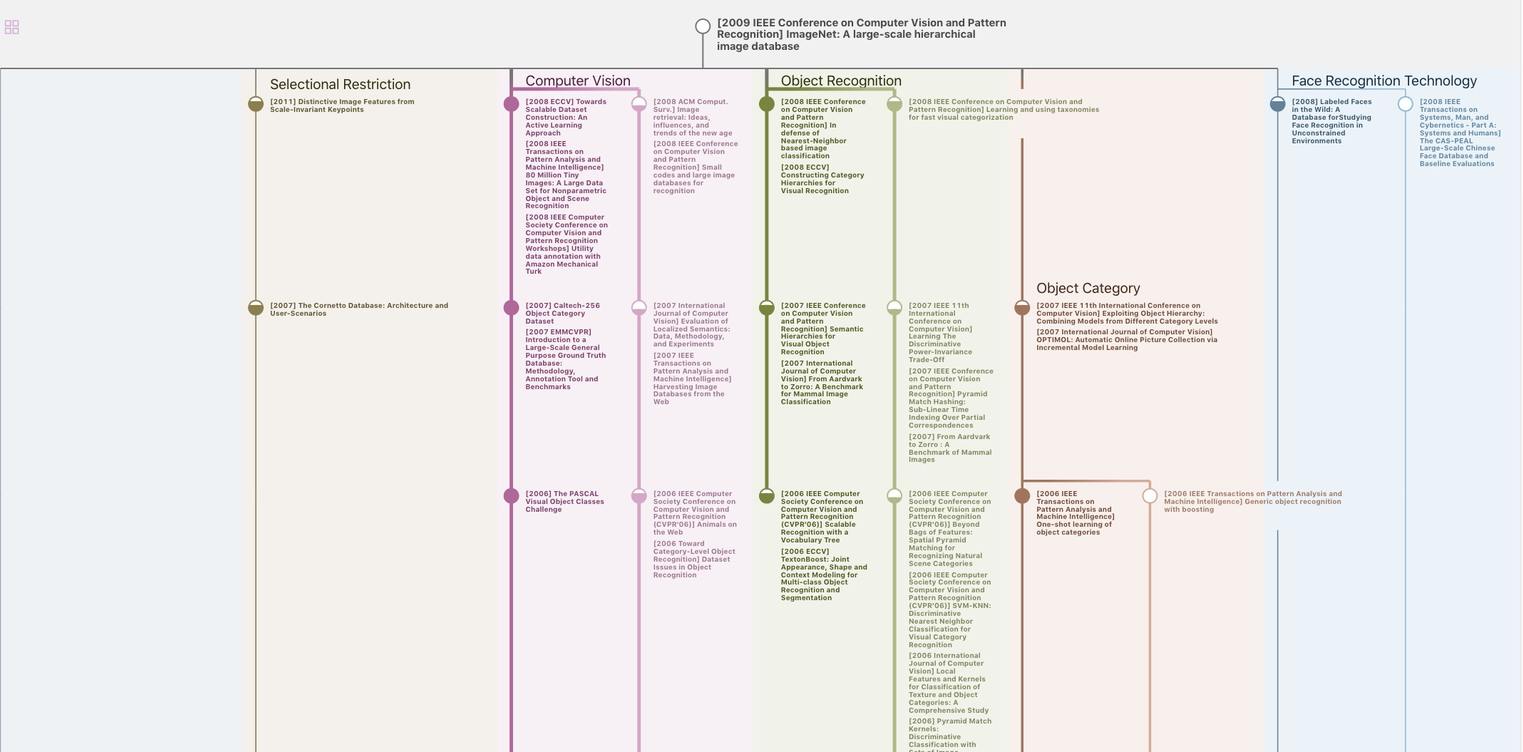
Generate MRT to find the research sequence of this paper
Chat Paper
Summary is being generated by the instructions you defined