FedEBA+: Towards Fair and Effective Federated Learning via Entropy-Based Model
arXiv (Cornell University)(2023)
摘要
Ensuring fairness is a crucial aspect of Federated Learning (FL), which
enables the model to perform consistently across all clients. However,
designing an FL algorithm that simultaneously improves global model performance
and promotes fairness remains a formidable challenge, as achieving the latter
often necessitates a trade-off with the former. To address this challenge, we
propose a new FL algorithm, FedEBA+, which enhances fairness while
simultaneously improving global model performance. FedEBA+ incorporates a fair
aggregation scheme that assigns higher weights to underperforming clients and
an alignment update method. In addition, we provide theoretical convergence
analysis and show the fairness of FedEBA+. Extensive experiments demonstrate
that FedEBA+ outperforms other SOTA fairness FL methods in terms of both
fairness and global model performance.
更多查看译文
关键词
effective federated learning,entropy-based
AI 理解论文
溯源树
样例
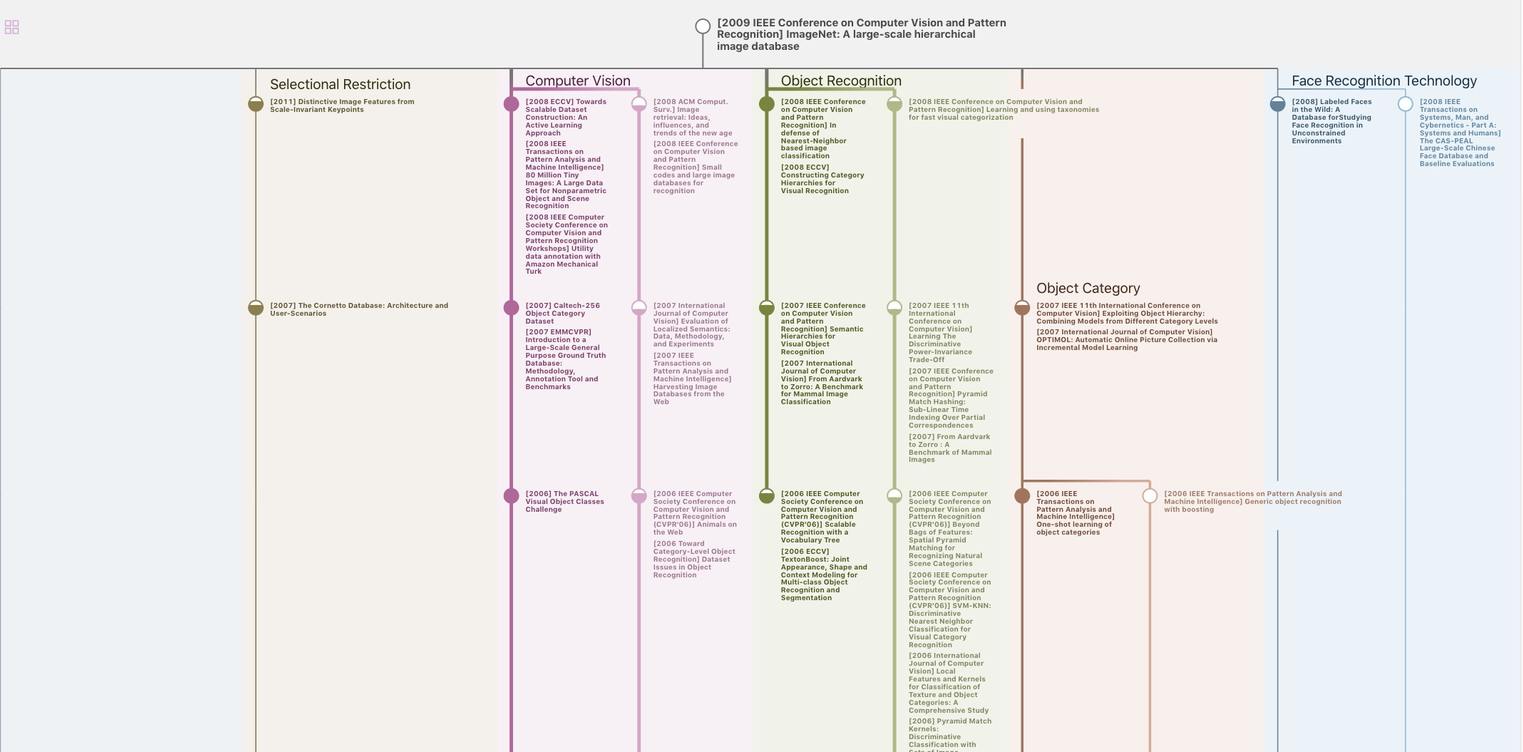
生成溯源树,研究论文发展脉络
Chat Paper
正在生成论文摘要