ZegOT: Zero-shot Segmentation Through Optimal Transport of Text Prompts
CoRR(2023)
Abstract
Recent success of large-scale Contrastive Language-Image Pre-training (CLIP) has led to great promise in zero-shot semantic segmentation by transferring image-text aligned knowledge to pixel-level classification. However, existing methods usually require an additional image encoder or retraining/tuning the CLIP module. Here, we present a cost-effective strategy using text-prompt learning that keeps the entire CLIP module frozen while fully leveraging its rich information. Specifically, we propose a novel Zero-shot segmentation with Optimal Transport (ZegOT) method that matches multiple text prompts with frozen image embeddings through optimal transport, which allows each text prompt to efficiently focus on specific semantic attributes. Additionally, we propose Deep Local Feature Alignment (DLFA) that deeply aligns the text prompts with intermediate local feature of the frozen image encoder layers, which significantly boosts the zero-shot segmentation performance. Through extensive experiments on benchmark datasets, we show that our method achieves the state-of-the-art (SOTA) performance with only x7 lighter parameters compared to previous SOTA approaches.
MoreTranslated text
Key words
text prompts,segmentation,zero-shot
AI Read Science
Must-Reading Tree
Example
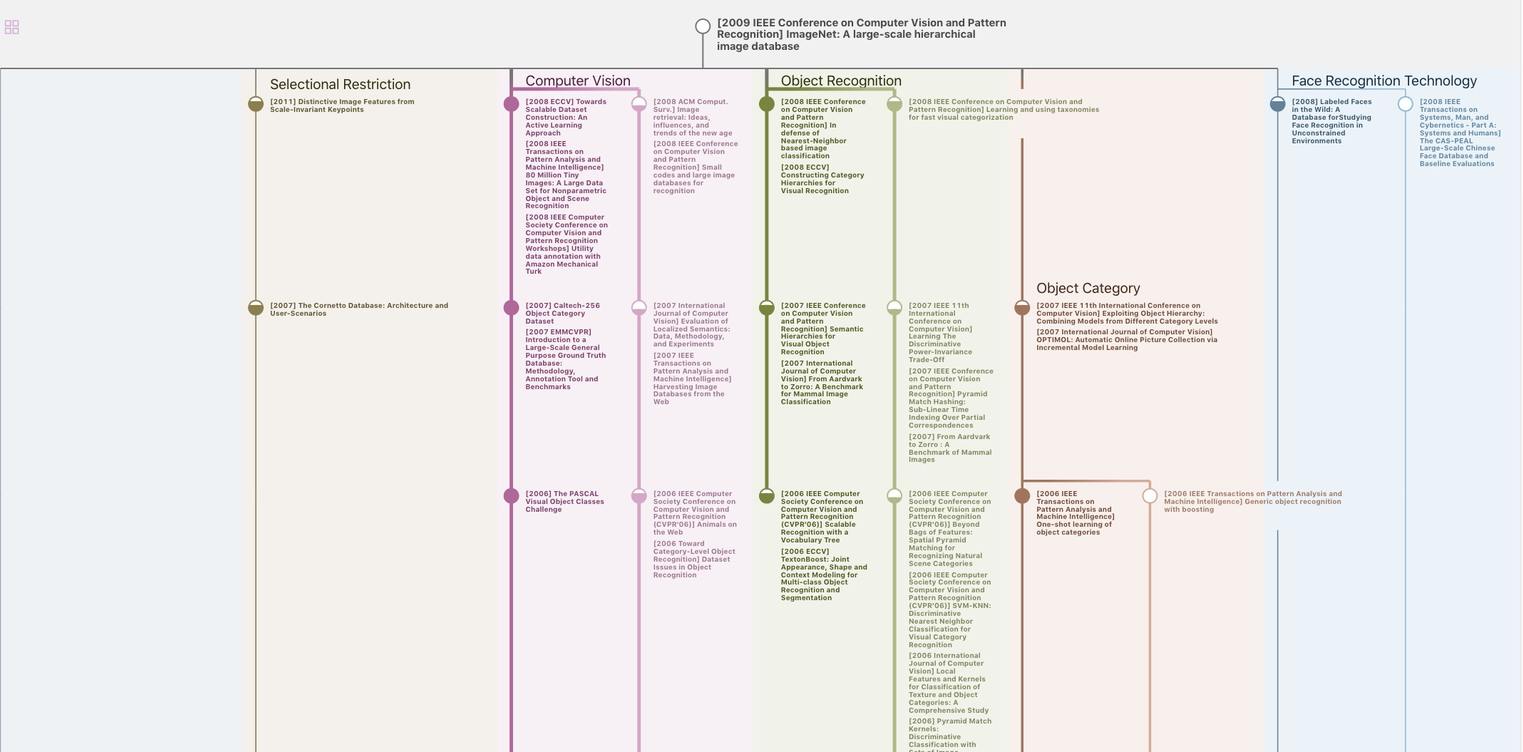
Generate MRT to find the research sequence of this paper
Chat Paper
Summary is being generated by the instructions you defined