Statistical whitening of neural populations with gain-modulating interneurons
CoRR(2023)
摘要
Statistical whitening transformations play a fundamental role in many computational systems, and may also play an important role in biological sensory systems. Individual neurons appear to rapidly and reversibly alter their input-output gains, approximately normalizing the variance of their responses. Populations of neurons appear to regulate their joint responses, reducing correlations between neural activities. It is natural to see whitening as the objective that guides these behaviors, but the mechanism for such joint changes is unknown, and direct adjustment of synaptic interactions would seem to be both too slow, and insufficiently reversible. Motivated by the extensive neuroscience literature on rapid gain modulation, we propose a recurrent network architecture in which joint whitening is achieved through modulation of gains within the circuit. Specifically, we derive an online statistical whitening algorithm that regulates the joint second-order statistics of a multi-dimensional input by adjusting the marginal variances of an overcomplete set of interneuron projections. The gains of these interneurons are adjusted individually, using only local signals, and feed back onto the primary neurons. The network converges to a state in which the responses of the primary neurons are whitened. We demonstrate through simulations that the behavior of the network is robust to poor conditioning or noise when the gains are sign-constrained, and can be generalized to achieve a form of local whitening in convolutional populations, such as those found throughout the visual or auditory system.
更多查看译文
AI 理解论文
溯源树
样例
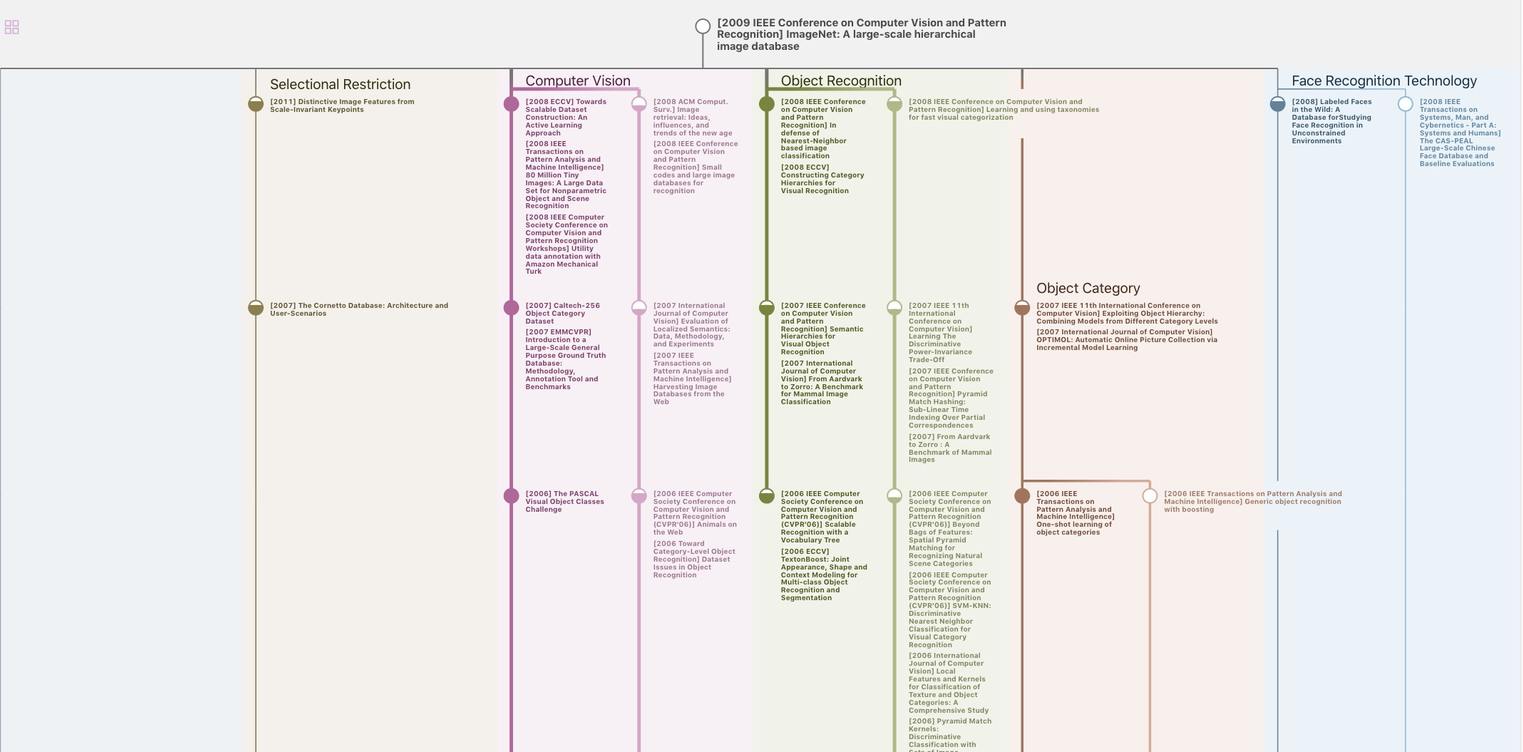
生成溯源树,研究论文发展脉络
Chat Paper
正在生成论文摘要