Machine learning assisted discovery of exotic criticality in a planar quasicrystal
arxiv(2023)
摘要
Our understanding of universality and phase transitions is deeply rooted in the notion of scaling. Indeed continuous phase transitions typically exhibit scale-invariant behavior facilitating the use of standard renormalization group (RG) techniques. Some critical systems, however, evade full scale invariance, in that observables reproduce themselves only under a set of discrete scale factors $\delta^n$. Such discrete scale invariance (DSI) presents a conceptual challenge as many of our theoretical tools fail to be directly applicable. Here, we report on a discovery of emergent degrees of freedom for the recently studied classical dimer model on the quasiperiodic Ammann-Beenker tiling. Using a machine learning assisted approach we establish that their statistics can be expressed in terms of emergent large-scale super-dimers. Moreover, the result reveals an emergent discrete scale invariance, where the same dimer problem is re-appearing at successive discrete coarse-grained levels, demonstrating proximity to an RG fixed point. Our findings not only provide a rare example of successfully applying RG to a strongly-correlated system on a two-dimensional quasicrystal, but, owing to the generality of the approach, delineate a new paradigm in analysis and a practical tool for discovering coarse-grained representations in quasiperiodic and other non-homogeneous systems.
更多查看译文
AI 理解论文
溯源树
样例
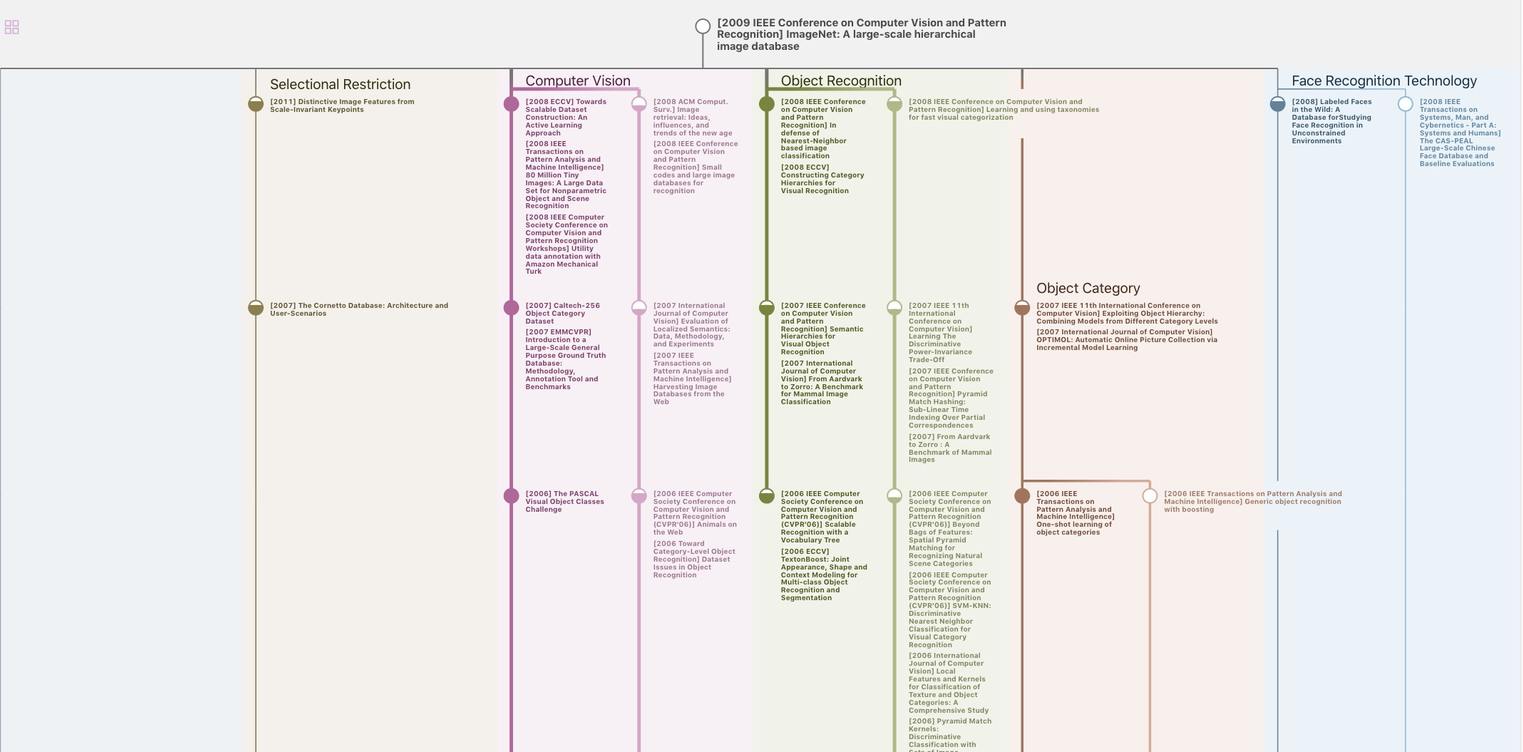
生成溯源树,研究论文发展脉络
Chat Paper
正在生成论文摘要