Energy-Efficient FPGA Implementation of Power-of-2 Weights-Based Convolutional Neural Networks With Low Bit-Precision Input Images
IEEE Transactions on Circuits and Systems II: Express Briefs(2023)
摘要
Convolutional neural networks (CNNs) have performed exceptionally well on a variety of image classification tasks but need significant amount of memory and computational resources. In this brief, we propose a power-of-2 weights based CNN inference engine, that takes images whose pixels are either binarized or quantized to low-resolution and also has its weights at each layer reduced to powers-of-2, thus replacing the multiplication operations with a simple shift operation, thereby reducing the computations significantly. The proposed CNN architecture, designed to be used in an always-on inference engine, has been demonstrated for SVHN and CIFAR-10 datasets and achieves an accuracy of 83.23% and 87.02%, respectively, while using 3-bit quantized images. The proposed architecture, therefore, achieves a performance comparable to inference engines that used 8-bit quantized images while achieving a frame-rate of 380 FPS by consuming 0.789 W of dynamic power, a very competitive energy efficiency of 892.45 GOPs/J. The system requires ≈1 Mb of memory for storing weights, making it suitable for memory constrained edge devices and ASIC implementations.
更多查看译文
关键词
CNN,CIFAR-10,SVHN,low-resolution images
AI 理解论文
溯源树
样例
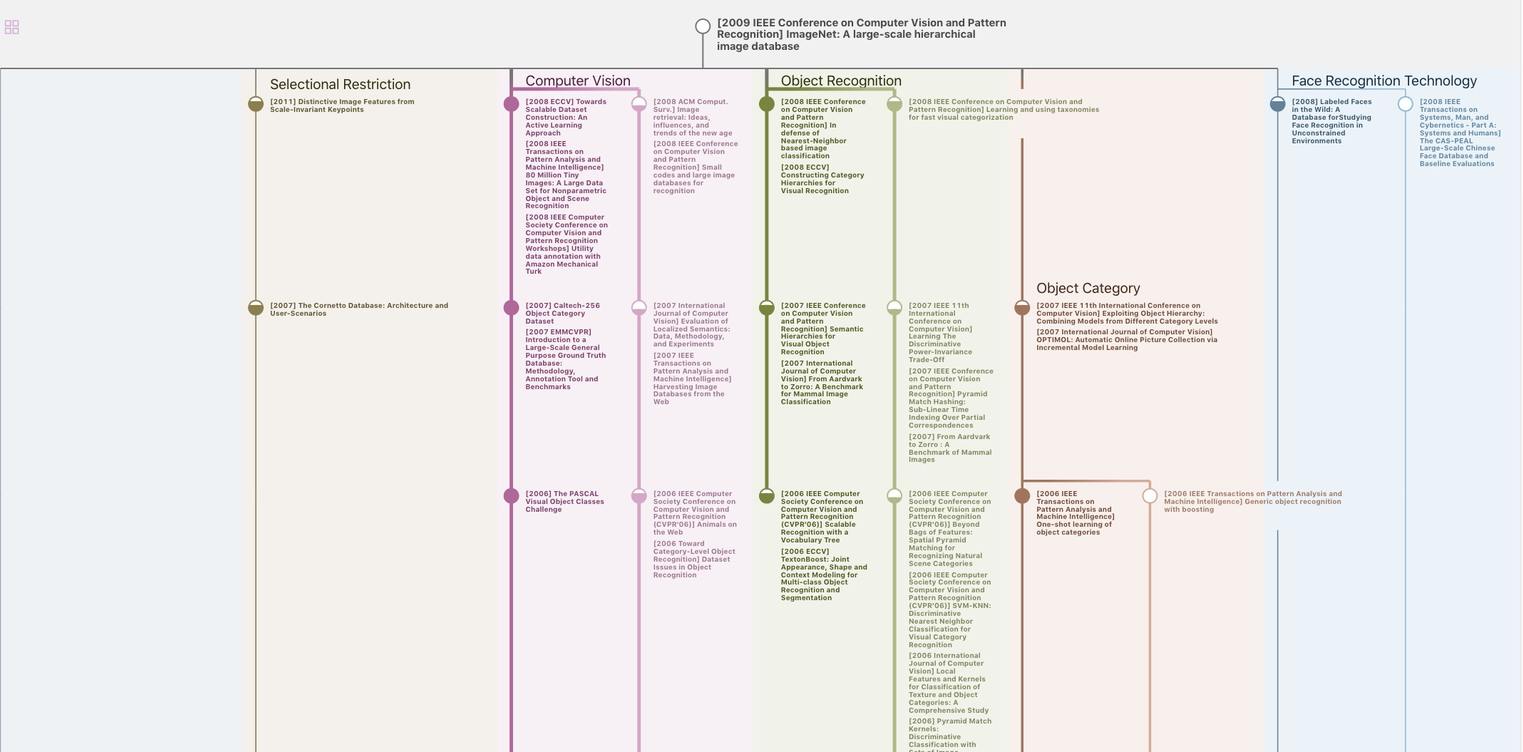
生成溯源树,研究论文发展脉络
Chat Paper
正在生成论文摘要