Towards Symmetry-Aware Pneumonia Detection on Chest X-Rays
2022 IEEE Symposium Series on Computational Intelligence (SSCI)(2022)
摘要
Chest X-rays show elements of bilateral symmetry of the lung field, which can be disturbed by various lung diseases. These bilateral differences are taken into account by physicians during routine radiology examinations and form the basis for diagnosing various lung diseases. While for other medical computer vision tasks such as pelvic fracture detection the bilateral symmetry of the domain is already considered, this has not yet been sufficiently explored in the evaluation of chest X-rays to aid in the diagnosis of lung diseases. To this end, we developed a symmetry-aware deep learning architecture for the classification of bacterial and viral pneumonia, demonstrating the effectiveness of symmetry-aware models on lung conditions. Our work builds upon the idea of Siamese networks, which independently process the left and right lung and fuse the two learned representations in downstream layers for classification. Two different feature map fusion methods are implemented, by integrating a difference merging layer, and by concatenating the feature maps. It is shown that the performance of the network can be improved by symmetry-motivated adaptation of the architecture in terms of AUROC and F1 score by up to 0.8% and 2.0%, respectively, without the introduction of extended loss functions. In addition, our analysis of the activation maps illustrates that the focus of the network improves compared to the baseline model. Our proposed architecture focuses on the lung lobes without a region of interest crop, pinpointing the effectiveness of symmetry incorporation. By incorporating the prior medical knowledge of the bilateral symmetry of the lung field, a more data-efficient algorithm can be developed, leading to comparable performances with fewer data samples.
更多查看译文
关键词
Siamese Network,Lung Abnormalities,Lung Region Symmetry
AI 理解论文
溯源树
样例
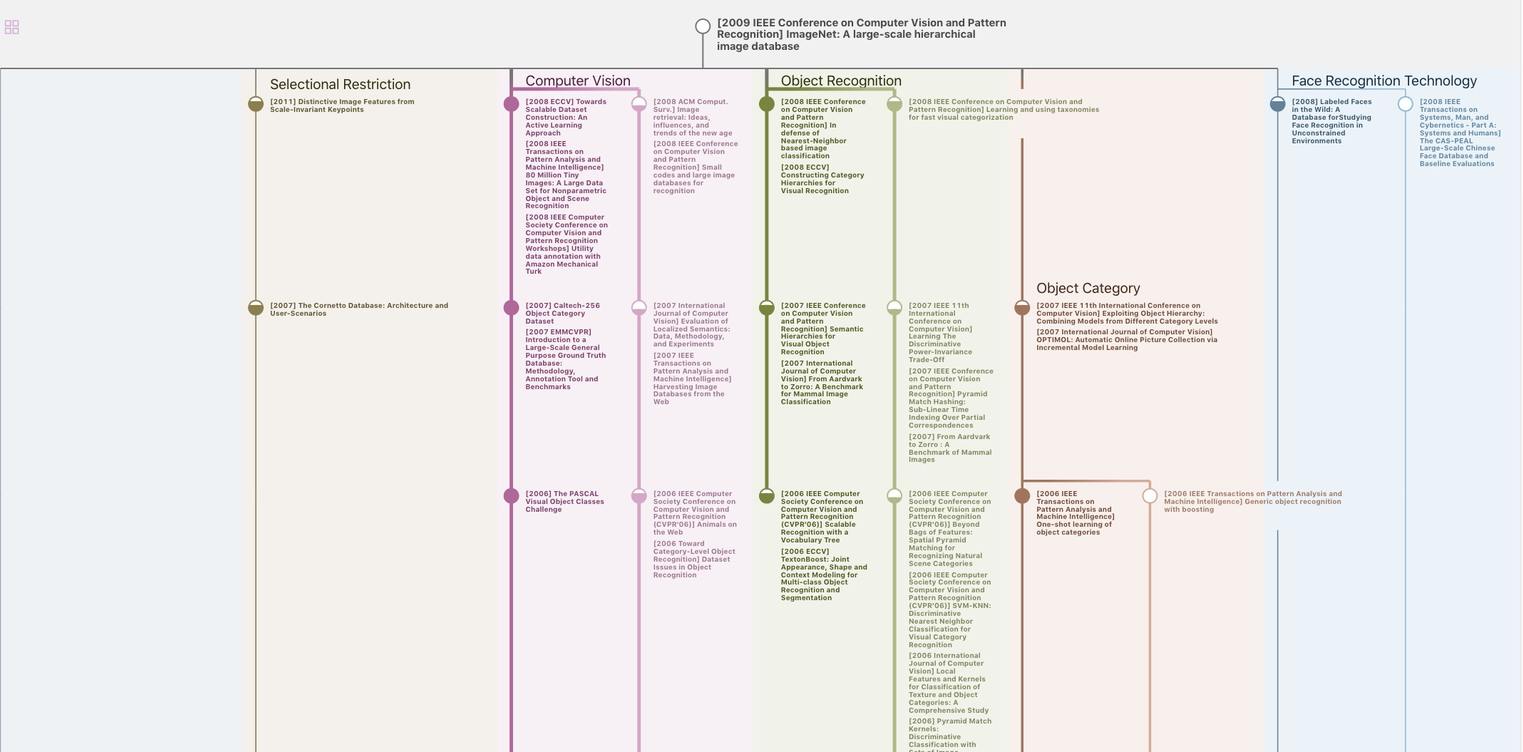
生成溯源树,研究论文发展脉络
Chat Paper
正在生成论文摘要