A Quasi oppositional smell agent optimization and its levy flight variant: A PV/Wind/battery system optimization application
APPLIED SOFT COMPUTING(2023)
摘要
In this study, two novel algorithms are developed: the quasi-oppositional smell agent optimization (QOBL-SAO) and its levy flight variation (LFQOBL-SAO), and their performance is compared to that of the conventional smell agent optimization (SAO). Two investigations were carried out. First, the capabilities of the novel algorithms were tested in solving ten benchmarked functions and five CEC2020 real-world optimization problems. Second, they are applied to optimize the hybrid photovoltaic (PV)/wind/battery, PV/battery, and wind/battery power system for a healthcare center in a Nigerian village. Load demand, PV and wind profiles of the aforementioned location were used to develop the hybrid system. All simulations were carried out in MATLAB software. The results show that the novel algorithms can solve benchmarked functions and the CEC2020 real-world constrained optimization competition. In particular, the performance of the QOBL and the LF-QOBL are as good as the top performing functions like the IUDE, epsilon MAgES and the iLSHAD epsilon algorithms. However, in terms of convergence time, lowest cost of energy (LCE), and total annualized cost (TAC), the novel algorithms outperformed the SAO for the PV/wind/battery optimization application. The hybrid PV/wind/battery system is the most cost-effective when using LFQOBL-SAO and QOBL-SAO, with a TAC value of $15100. Furthermore, the results demonstrate that the LFQOBL-SAO method is accurate and outperforms the QOBL-SAO and SAO algorithms.(c) 2023 Elsevier B.V. All rights reserved.
更多查看译文
关键词
Quasi oppositional smell agent,optimization,Levy flight quasi oppositional smell agent,Smell agent optimization,Photovoltaic,Wind,Renewable energy sources,Lowest cost of energy,Net present cost and hybrid system
AI 理解论文
溯源树
样例
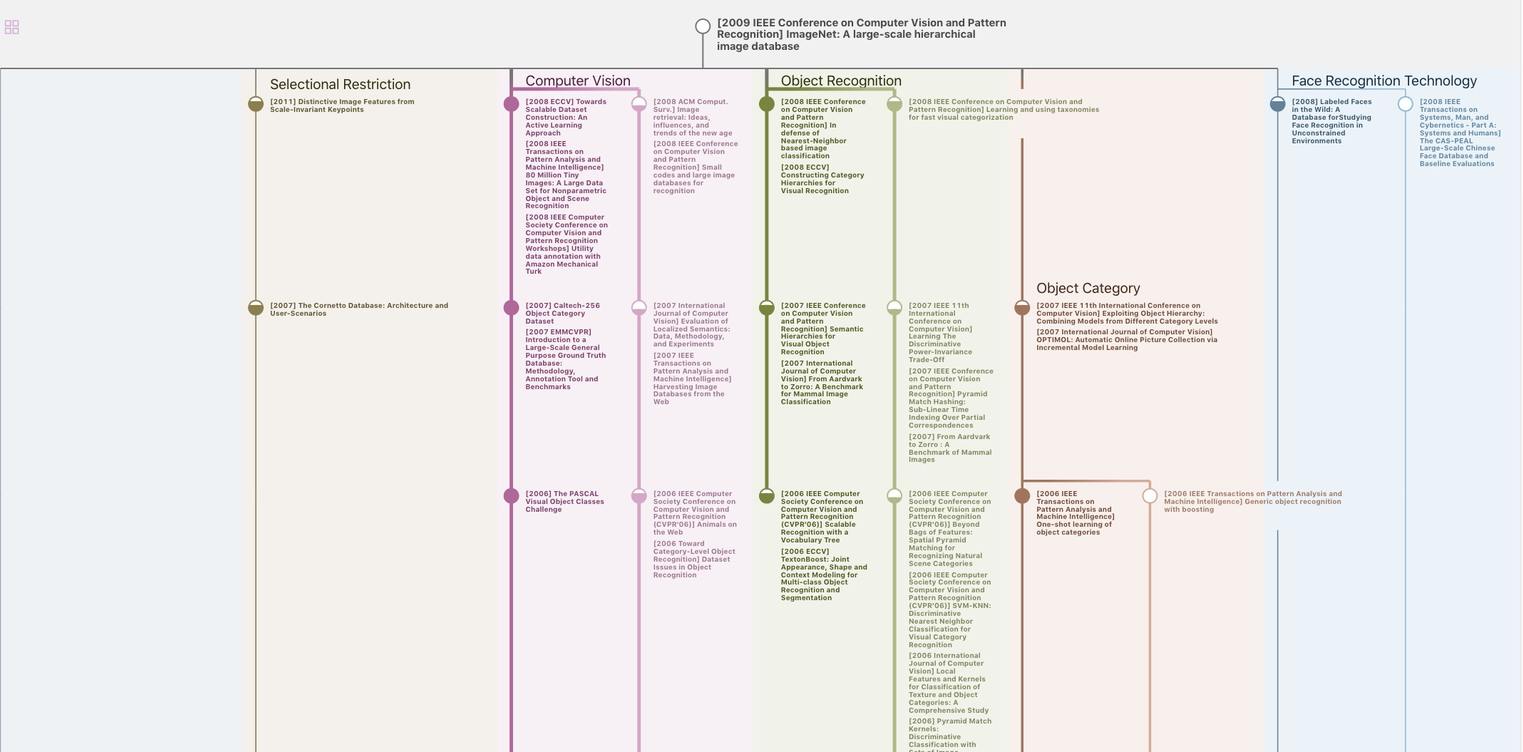
生成溯源树,研究论文发展脉络
Chat Paper
正在生成论文摘要