Learning by reusing previous advice: a memory-based teacher–student framework
Autonomous Agents and Multi-Agent Systems(2022)
摘要
Reinforcement Learning (RL) has been widely used to solve sequential decision-making problems. However, it often suffers from slow learning speed in complex scenarios. Teacher–student frameworks address this issue by enabling agents to ask for and give advice so that a student agent can leverage the knowledge of a teacher agent to facilitate its learning. In this paper, we consider the effect of reusing previous advice, and propose a novel memory-based teacher–student framework such that student agents can memorize and reuse the previous advice from teacher agents. In particular, we propose two methods to decide whether previous advice should be reused: Q-Change per Step that reuses the advice if it leads to an increase in Q-values, and Decay Reusing Probability that reuses the advice with a decaying probability. The experiments on diverse RL tasks (Mario, Predator–Prey and Half Field Offense) confirm that our proposed framework significantly outperforms the existing frameworks in which previous advice is not reused.
更多查看译文
关键词
Reinforcement learning, Multi-agent learning, Action advising, Teacher–student
AI 理解论文
溯源树
样例
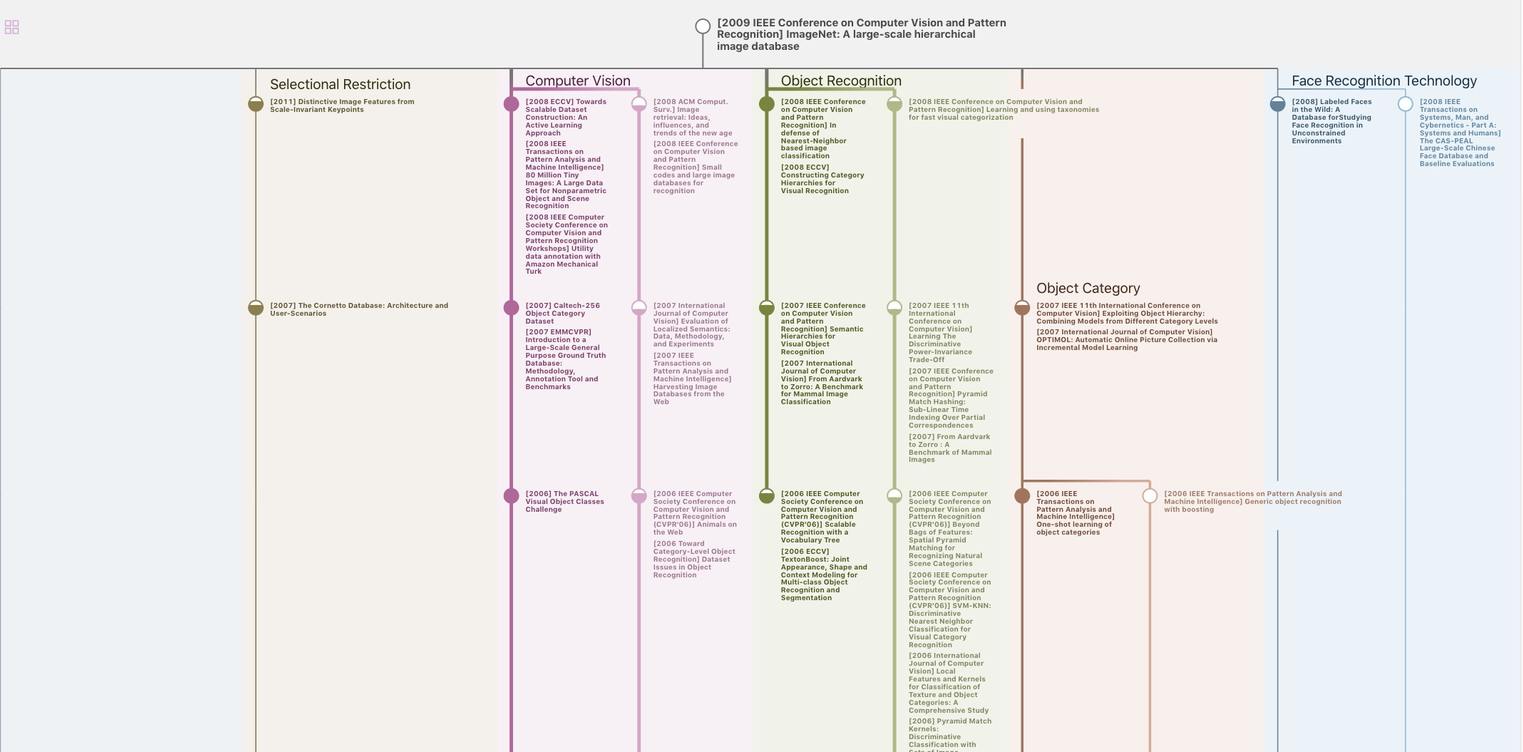
生成溯源树,研究论文发展脉络
Chat Paper
正在生成论文摘要