Omnidirectional 2.5D representation for COVID-19 diagnosis using chest CTs
Journal of Visual Communication and Image Representation(2023)
摘要
The Coronavirus Disease 2019 (COVID-19) has drastically overwhelmed most countries in the last two years, and image-based approaches using computerized tomography (CT) have been used to identify pulmonary infections. Recent methods based on deep learning either require time-consuming per-slice annotations (2D) or are highly data- and hardware-demanding (3D). This work proposes a novel omnidirectional 2.5D representation of volumetric chest CTs that allows exploring efficient 2D deep learning architectures while requiring volume-level annotations only. Our learning approach uses a siamese feature extraction backbone applied to each lung. It combines these features into a classification head that explores a novel combination of Squeeze-and-Excite strategies with Class Activation Maps. We experimented with public and in-house datasets and compared our results with state-of-the-art techniques. Our analyses show that our method provides better or comparable prediction quality and accurately distinguishes COVID-19 infections from other kinds of pneumonia and healthy lungs.
更多查看译文
关键词
2.5D representation,COVID-19 diagnosis,Ground-glass opacity,Omnidirectional imaging
AI 理解论文
溯源树
样例
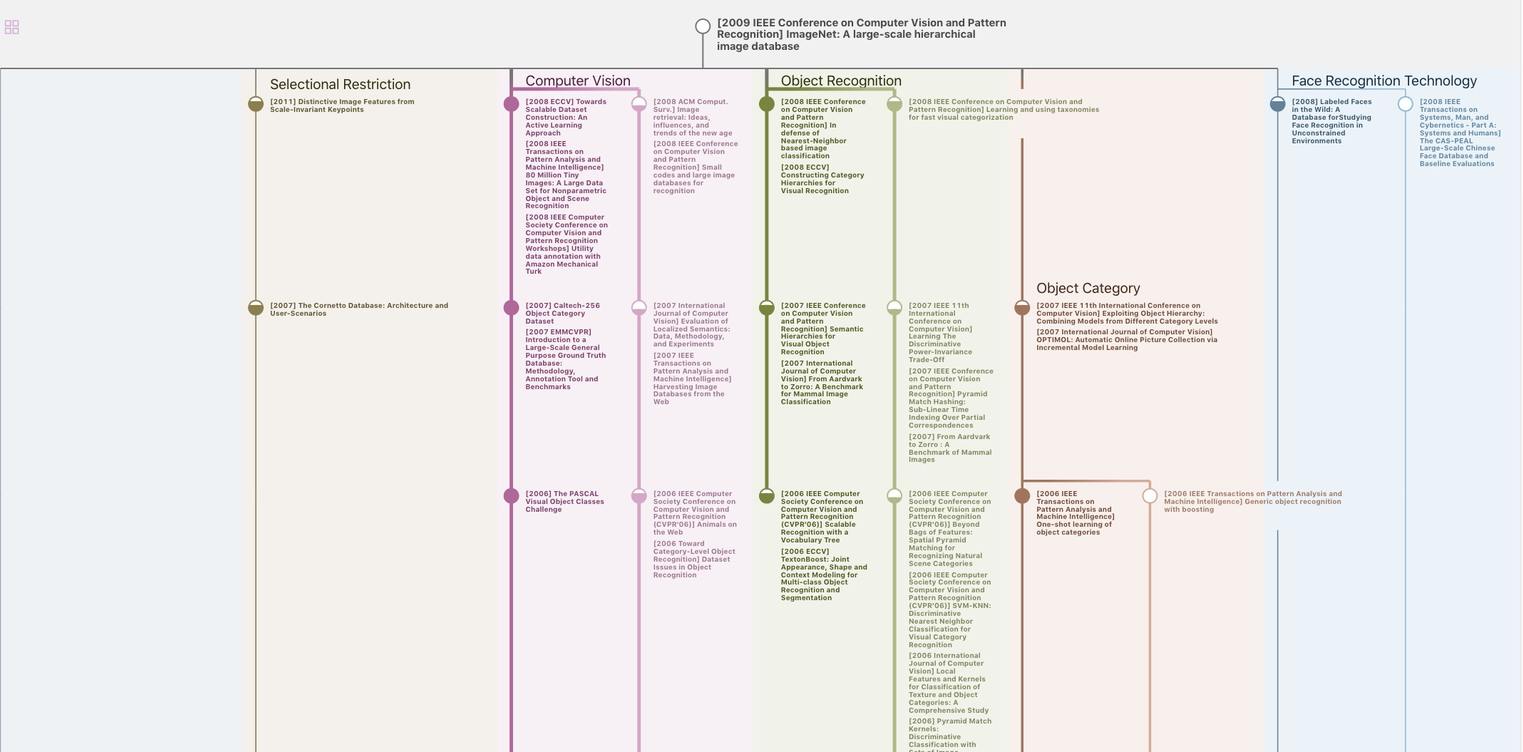
生成溯源树,研究论文发展脉络
Chat Paper
正在生成论文摘要