Intra-class consistency and inter-class discrimination feature learning for automatic skin lesion classification.
Medical image analysis(2023)
摘要
Automated skin lesion classification has been proved to be capable of improving the diagnostic performance for dermoscopic images. Although many successes have been achieved, accurate classification remains challenging due to the significant intra-class variation and inter-class similarity. In this article, a deep learning method is proposed to increase the intra-class consistency as well as the inter-class discrimination of learned features in the automatic skin lesion classification. To enhance the inter-class discriminative feature learning, a CAM-based (class activation mapping) global-lesion localization module is proposed by optimizing the distance of CAMs for the same dermoscopic image generated by different skin lesion tasks. Then, a global features guided intra-class similarity learning module is proposed to generate the class center according to the deep features of all samples in one class and the history feature of one sample during the learning process. In this way, the performance can be improved with the collaboration of CAM-based inter-class feature discriminating and global features guided intra-class feature concentrating. To evaluate the effectiveness of the proposed method, extensive experiments are conducted on the ISIC-2017 and ISIC-2018 datasets. Experimental results with different backbones have demonstrated that the proposed method has good generalizability and can adaptively focus on more discriminative regions of the skin lesion.
更多查看译文
关键词
Class activation mapping,Inter-class feature discrimination,Intra-class feature concentration,Skin lesion classification
AI 理解论文
溯源树
样例
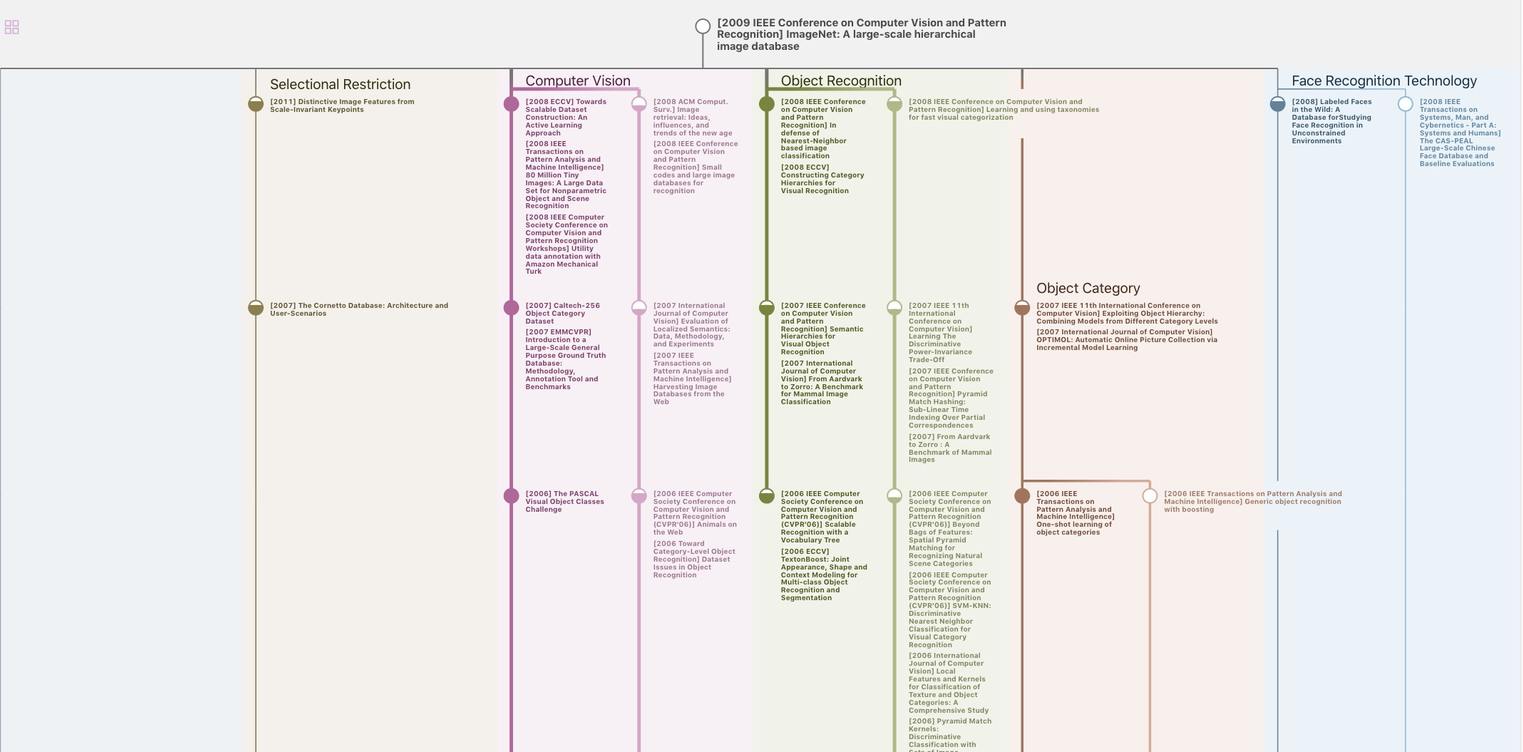
生成溯源树,研究论文发展脉络
Chat Paper
正在生成论文摘要