Towards Practical Secure Neural Network Inference: The Journey So Far and the Road Ahead
ACM COMPUTING SURVEYS(2024)
摘要
Neural networks (NNs) have become one of the most important tools for artificial intelligence. Well-designed and trained NNs can perform inference (e.g., make decisions or predictions) on unseen inputs with high accuracy. Using NNs often involves sensitive data: Depending on the specific use case, the input to the NN and/or the internals of the NN (e.g., the weights and biases) may be sensitive. Thus, there is a need for techniques for performing NN inference securely, ensuring that sensitive data remain secret. In the past few years, several approaches have been proposed for secure neural network inference. These approaches achieve better and better results in terms of efficiency, security, accuracy, and applicability, thus making big progress toward practical secure neural network inference. The proposed approaches make use of many different techniques, such as homomorphic encryption and secure multi-party computation. The aim of this article is to give an overview of the main approaches proposed so far, their different properties, and the techniques used. In addition, remaining challenges toward large-scale deployments are identified.
更多查看译文
关键词
Privacy-preserving machine learning,secure inference,neural networks,deep learning,secure computation,homomorphic encryption,multi-party computation
AI 理解论文
溯源树
样例
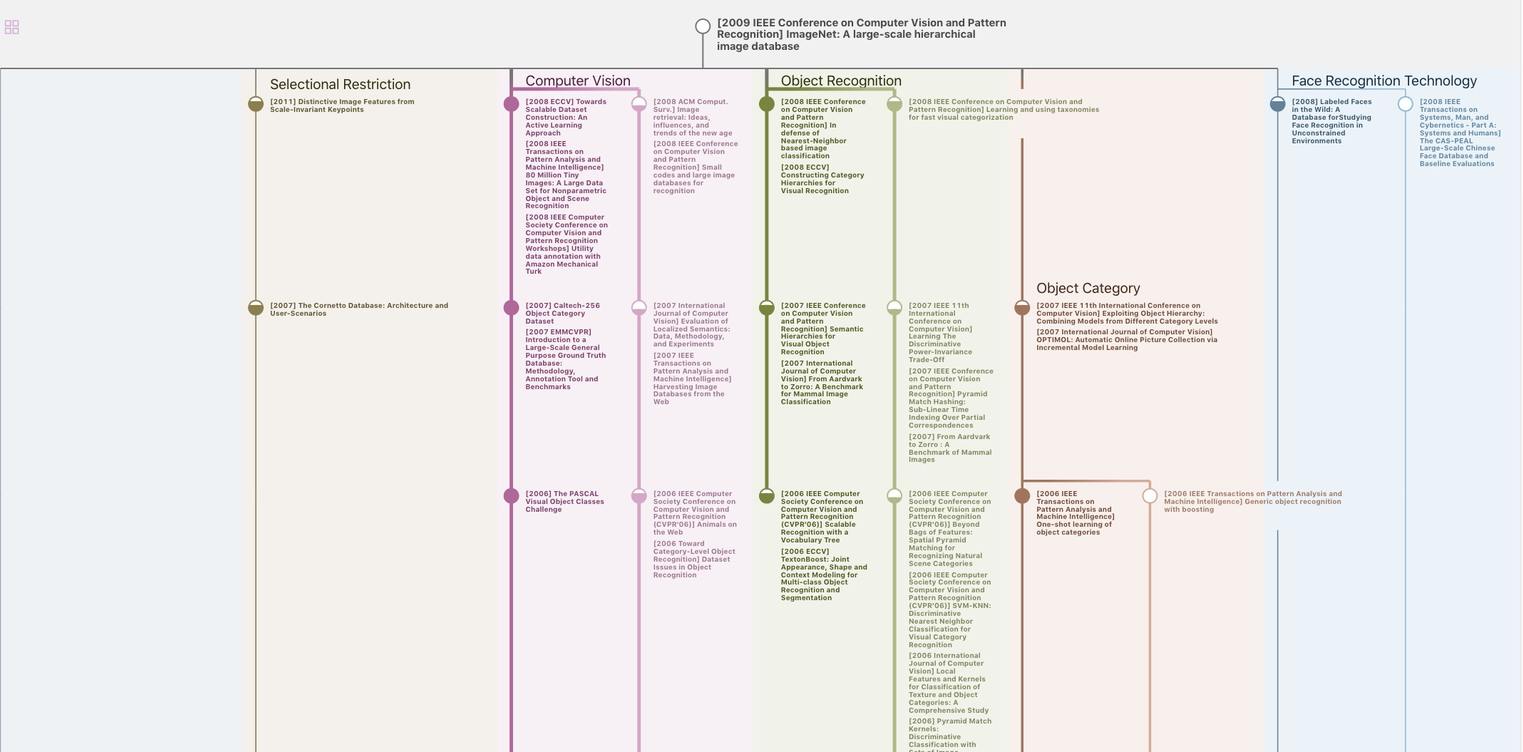
生成溯源树,研究论文发展脉络
Chat Paper
正在生成论文摘要