Multiscale convolutional neural network for no-reference image quality assessment with saliency detection
Multim. Tools Appl.(2022)
摘要
In recent years, Convolutional Neural Network (CNN) has been gradually applied to Image Quality Assessment (IQA). Most CNNs segment the image into patches for training, which lead to increase of data and affect calculation speed of the model. Meanwhile, the parameters of CNN usually reach millions, which is the root cause of overfitting. In this paper, a multiscale CNN for NR-IQA is established to solve these problems. Since IQA simulates the perception of Human Visual System (HVS) on image quality, salient areas are more valuable for reference. Therefore a patch sampling method was designed based on saliency detection. Firstly, patches with salient values between given thresholds are retained as training data. Secondly, the sampled patches are fed into multiscale CNN. The network consists of three branches with multiscale convolutional kernels. Finally, the weighted average of the quality scores from the salient patches is the final score. The CNN was trained on LIVE dataset and cross-validated on CSIQ dataset. The experimental results show that the proposed method can achieve better performance with fewer parameters compared with state-of-the-art NR-IQA algorithms.
更多查看译文
关键词
Convolutional neural network,No-reference image quality assessment,Human visual system,Saliency detection,Multiscale network
AI 理解论文
溯源树
样例
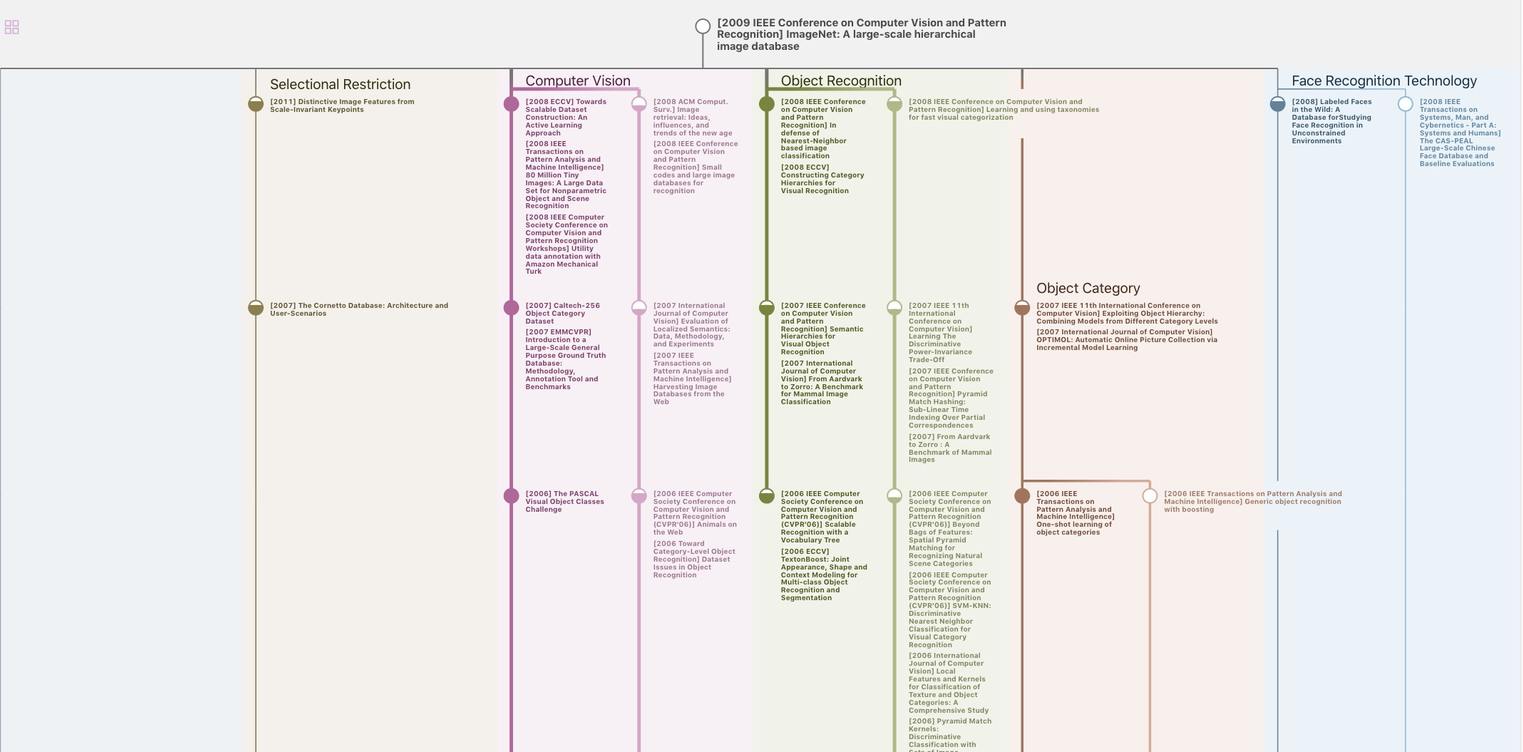
生成溯源树,研究论文发展脉络
Chat Paper
正在生成论文摘要