Dynamic Channel-Aware Subgraph Interactive Networks for Skeleton-Based Action Recognition.
IEEE Signal Process. Lett.(2022)
摘要
Skeleton-based action recognition has obtained remarkable success due to the rapid development of Graph Neural Networks (GNNs). Existing skeleton-based methods primarily focus on the spatial aspect of skeleton graphs, but rarely mine long-range temporal relationships to learn the intrinsic dependencies across different frames. The latter is crucial for extracting discriminative motion patterns. To acquire a more precise spatial-temporal representation for the human skeleton data, we develop a lightweight yet practical method termed Dynamic Channel-Aware Subgraph Interactive Network (DCA-SGIN), using the interactive motion to adaptively capture long-range temporal nuance among the skeleton sequences. Moreover, we unify a novel paradigm to address the inherent structural limitations of GCNs. Specifically, the proposed graph interactive learners utilize the collaborative channel-aware topology of multiple subgraphs to model spatial relations. Unlike previous methods, the global and local features are simultaneously considered in spatial modeling without calculating any adjacency matrix, making it more efficient. Each proposed individual component in DCA-SGIN can be treated as a plug-in module that can be easily applied to other GNNs. Extensive experiments on the three challenging datasets show that the performance of DCA-SGIN outperforms the state-of-the-art methods with fewer FLOPs.
更多查看译文
关键词
Skeleton, Topology, Solid modeling, Adaptation models, Convolution, Computational modeling, Collaboration, Graph neural network, channel-aware, skeleton-based action recognition
AI 理解论文
溯源树
样例
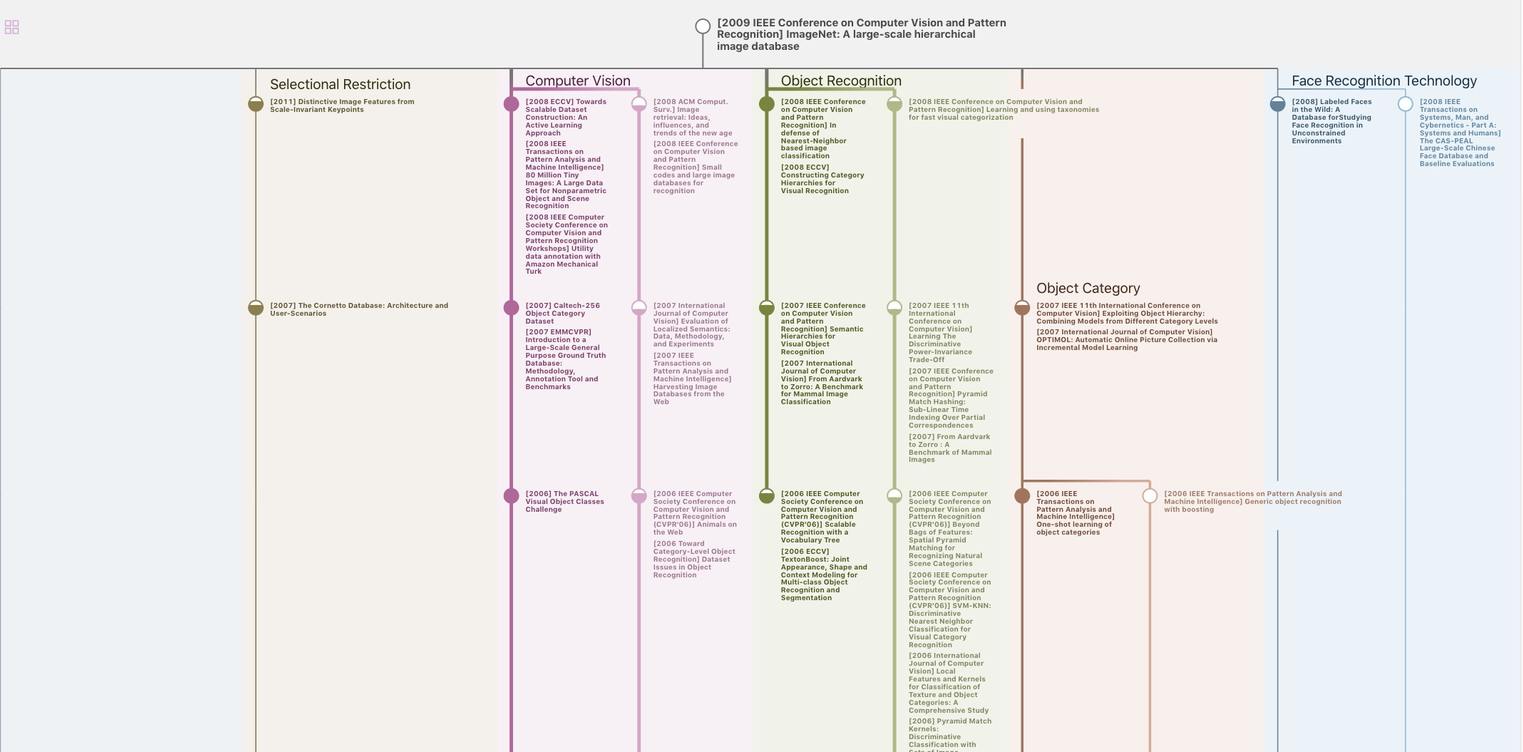
生成溯源树,研究论文发展脉络
Chat Paper
正在生成论文摘要