Efficient Algorithms for Bayesian Inverse Problems with Whittle-Matérn Priors.
SIAM J. Sci. Comput.(2024)
摘要
This paper tackles efficient methods for Bayesian inverse problems with priors based on Whittle–Matérn Gaussian random fields. The Whittle–Matérn prior is characterized by a mean function and a covariance operator that is taken as a negative power of an elliptic differential operator. This approach is flexible in that it can incorporate a wide range of prior information including nonstationary effects, but it is currently computationally advantageous only for integer values of the exponent. In this paper, we derive an efficient method for handling all admissible noninteger values of the exponent. The method first discretizes the covariance operator using finite elements and quadrature, and uses preconditioned Krylov subspace solvers for shifted linear systems to efficiently apply the resulting covariance matrix to a vector. This approach can be used for generating samples from the distribution in two different ways: by solving a stochastic partial differential equation, and by using a truncated Karhunen–Loève expansion. We show how to incorporate this prior representation into the infinite-dimensional Bayesian formulation, and show how to efficiently compute the maximum a posteriori estimate, and approximate the posterior variance. Although the focus of this paper is on Bayesian inverse problems, the techniques developed here are applicable to solving systems with fractional Laplacians and Gaussian random fields. Numerical experiments demonstrate the performance and scalability of the solvers and their applicability to model and real-data inverse problems in tomography and a time-dependent heat equation.
更多查看译文
关键词
bayesian inverse problems,whittle–matérn priors,efficient algorithms
AI 理解论文
溯源树
样例
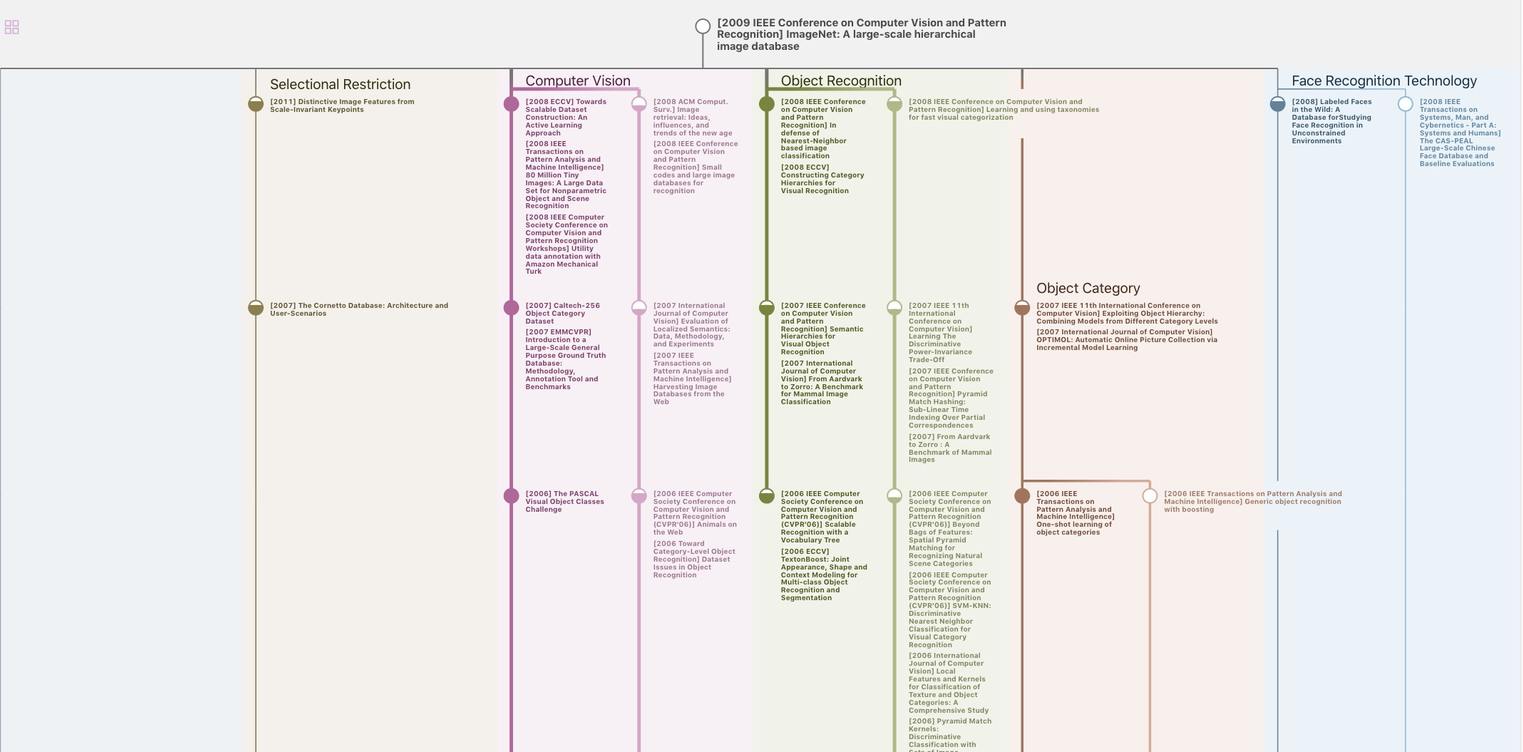
生成溯源树,研究论文发展脉络
Chat Paper
正在生成论文摘要