Graph Attention Network-Based Fault Detection for UAVs With Multivariant Time Series Flight Data.
IEEE Trans. Instrum. Meas.(2022)
摘要
Timely detection and isolation of faults is crucial to the survival of unmanned aerial vehicle (UAV) systems and to avoid threats to humans and the environment. This article focused on automatically and accurately identifying the faults with data-driven methods as traditional algorithms may rely on the knowledge of underlying system dynamics. The analytical redundancies are provided with the proposed masked spatial graph attention network (GAT) with gated recurrent unit (GRU) (masked-SGAT-GRU) model. The model extracts pairwise dependencies between variables from different components of UAVs explicitly, which is of vital importance in improving fault detection (FD) performance. With the finely optimized model, residuals are generated from measurements and predictions, which are smoothed with the exponential weighted moving average (EWMA) method to generate the proper fault thresholds. Real flight data under two flight modes are collected for model training and fault injection. The robustness and effectiveness of the model are demonstrated in FD with four types of faults compared with the commonly used data-driven methods.
更多查看译文
关键词
Exponential weighted moving average (EWMA),fault detection (FD),graph attention network (GAT),multivariant time series prediction,unmanned aerial vehicles (UAVs)
AI 理解论文
溯源树
样例
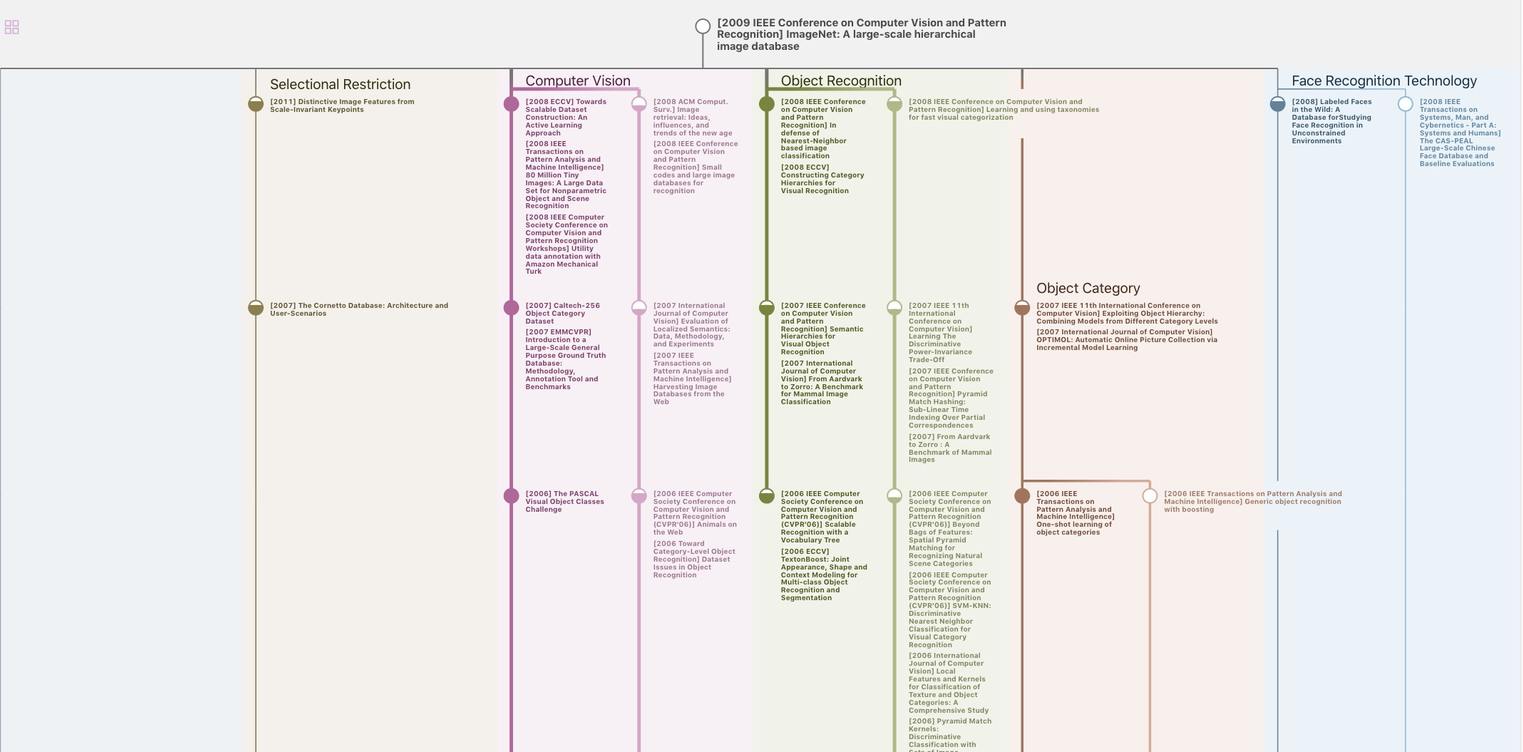
生成溯源树,研究论文发展脉络
Chat Paper
正在生成论文摘要