A spatiotemporal correlation deep learning network for brain penumbra disease
Neurocomputing(2023)
摘要
Brain penumbra is a critical condition that is closely related to stroke. Thus, there is high demand for fast and accurate segmentation of penumbra tissue in magnetic resonance images. However, most convolutional neural networks (CNNs) focus on learning contextual semantic information from two-dimensional imaging slides, ignoring the spatiotemporal correlations among adjacent slides. Here, we propose an encoder-decoder network (ConvLSTM-Net) with a specifically convolutional long short-term memory skip connection to extract the spatiotemporal correlations of features of adjacent slices in a non-linear manner. A mixed loss function is also used to improve the segmentation performance. We test the proposed method on the penumbra segmentation challenge and obtain an average Dice score over 80%, indicating that its performance is superior to or comparable with that of state-of-the-art segmentation methods. A mixed loss function provides positive support for the stability of model training. In addition, we visualize two representative samples to improve the interpretability of the results of ConvLSTM-Net.
更多查看译文
关键词
Brain penumbra,Convolutional neural networks,Adjacent slides,Convolutional long short-term memory
AI 理解论文
溯源树
样例
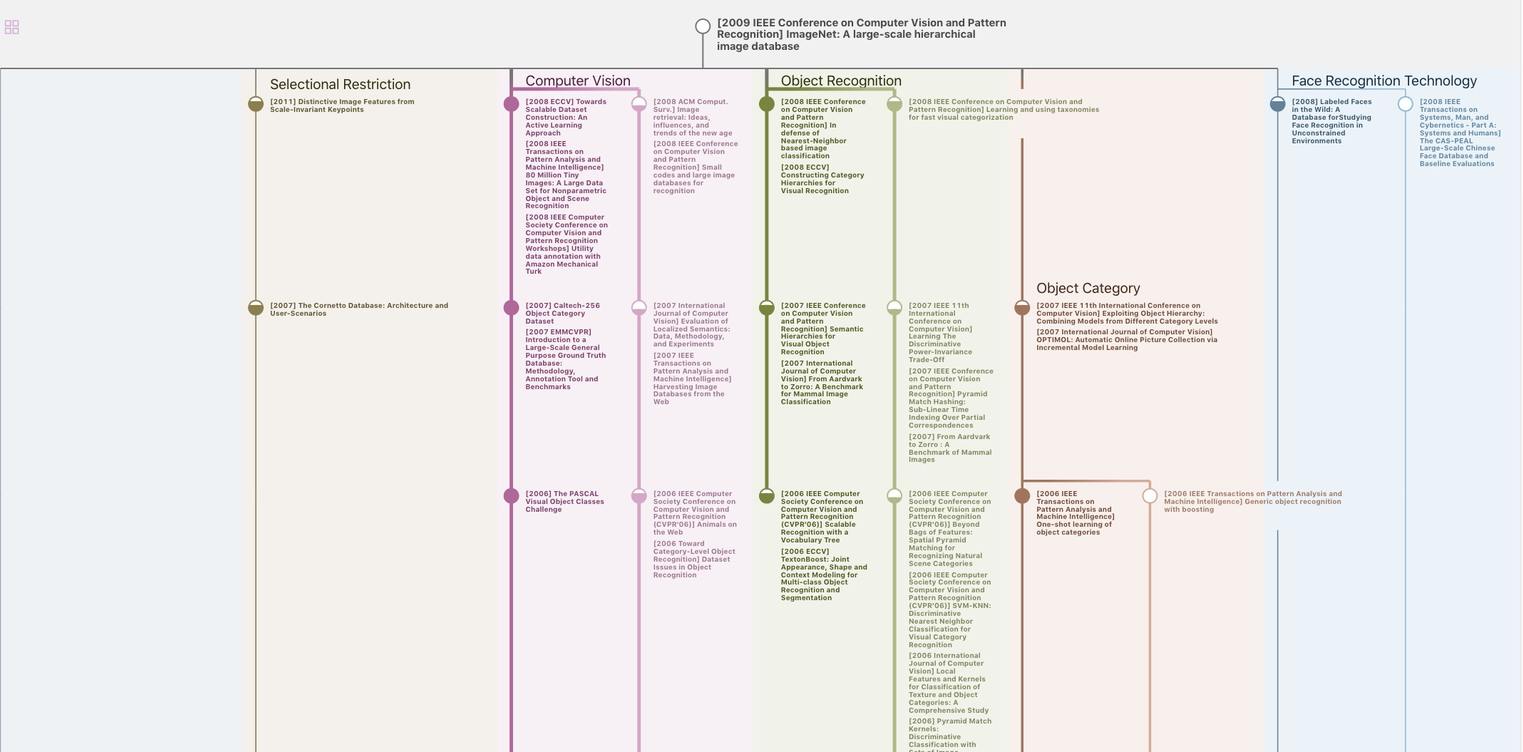
生成溯源树,研究论文发展脉络
Chat Paper
正在生成论文摘要