Considering three elements of aesthetics: Multi-task self-supervised feature learning for image style classification.
Neurocomputing(2022)
摘要
Image style classification is the basis of computational aesthetics, and its role has become increasingly important with the rise of computational aesthetics. Most of the current image style classification methods use supervised learning for model training. These methods require a large number of expensive aesthetic style labels. Unlike existing methods, self-supervised learning can perform feature learning on many current unlabeled style images, thereby alleviating the constraint that current supervised learning methods require a large amount of labeled data. However, the self-supervised learning method also poses the problem that it is difficult to fully characterize the highly subjective aesthetic style characteristics. Therefore, this study proposes a multi-task self-supervised style feature learning algorithm considering the three elements of aesthetics. The three elements of aesthetics include compositional rules, luminance and color. The algorithm designs multiple self-supervised learning tasks from multiple perspectives and proposes a joint learning method for multiple self-supervised learning tasks, so that the model can learn the style features of images more comprehensively than ordinary self-supervised learning methods. The experimental results on three large image style datasets also show that the method proposed in this paper can effectively learn the style features of images and outperform most style feature learning algorithms based on supervised learning in terms of classification results.
更多查看译文
AI 理解论文
溯源树
样例
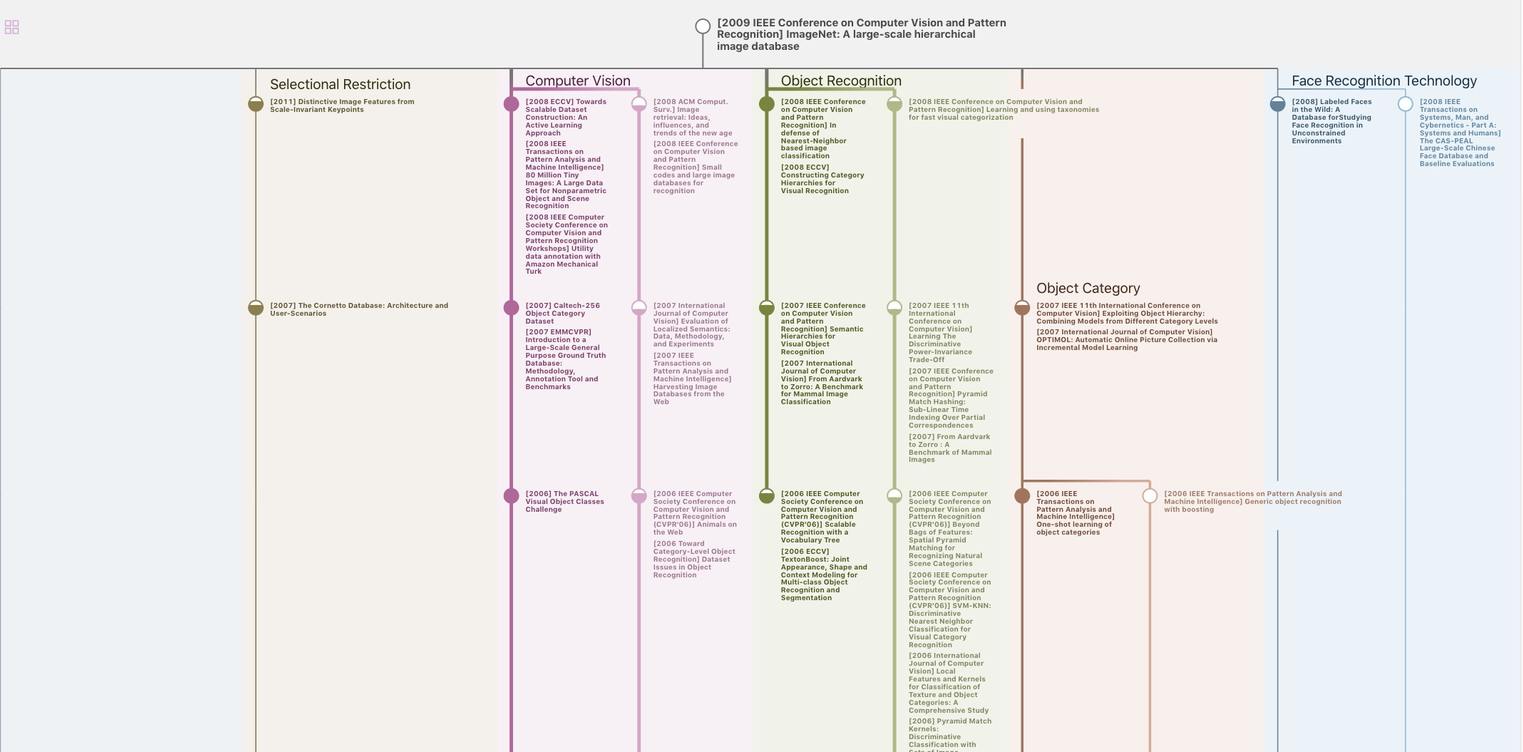
生成溯源树,研究论文发展脉络
Chat Paper
正在生成论文摘要