Field-Testing a Proteomics-Derived Machine-Learning Model for Predicting Coral Bleaching Susceptibility
Applied Sciences(2023)
摘要
Given the widespread decline of coral reefs, temperature-focused models have been generated to predict when and where bleaching events may occur (e.g., Coral Reef Watch). Although such algorithms are adept at forecasting the onset of bleaching in many areas, they suffer from poor predictive capacity in regions featuring corals that have adapted or acclimatized to life in marginal environments, such as reefs of the Florida Keys (USA). In these locales, it may instead be preferred to use physiological data from the corals themselves to make predictions about stress tolerance. Herein proteomic data from both laboratory and field samples were used to train neural networks and other machine-learning models to predict coral bleaching susceptibility in situ, and the models’ accuracies were field-tested with massive corals (Orbicella faveolata) sampled across a 2019 bleaching event. The resulting artificial intelligence was capable of accurately predicting whether or not a coral would bleach in response to high temperatures based on its protein signatures alone, meaning that this approach could consequently be of potential use in delineating O. faveolata climate resilience.
更多查看译文
关键词
artificial intelligence,coral reefs,dinoflagellates,global climate change,machine-learning,molecular biotechnology,proteomics,temperature
AI 理解论文
溯源树
样例
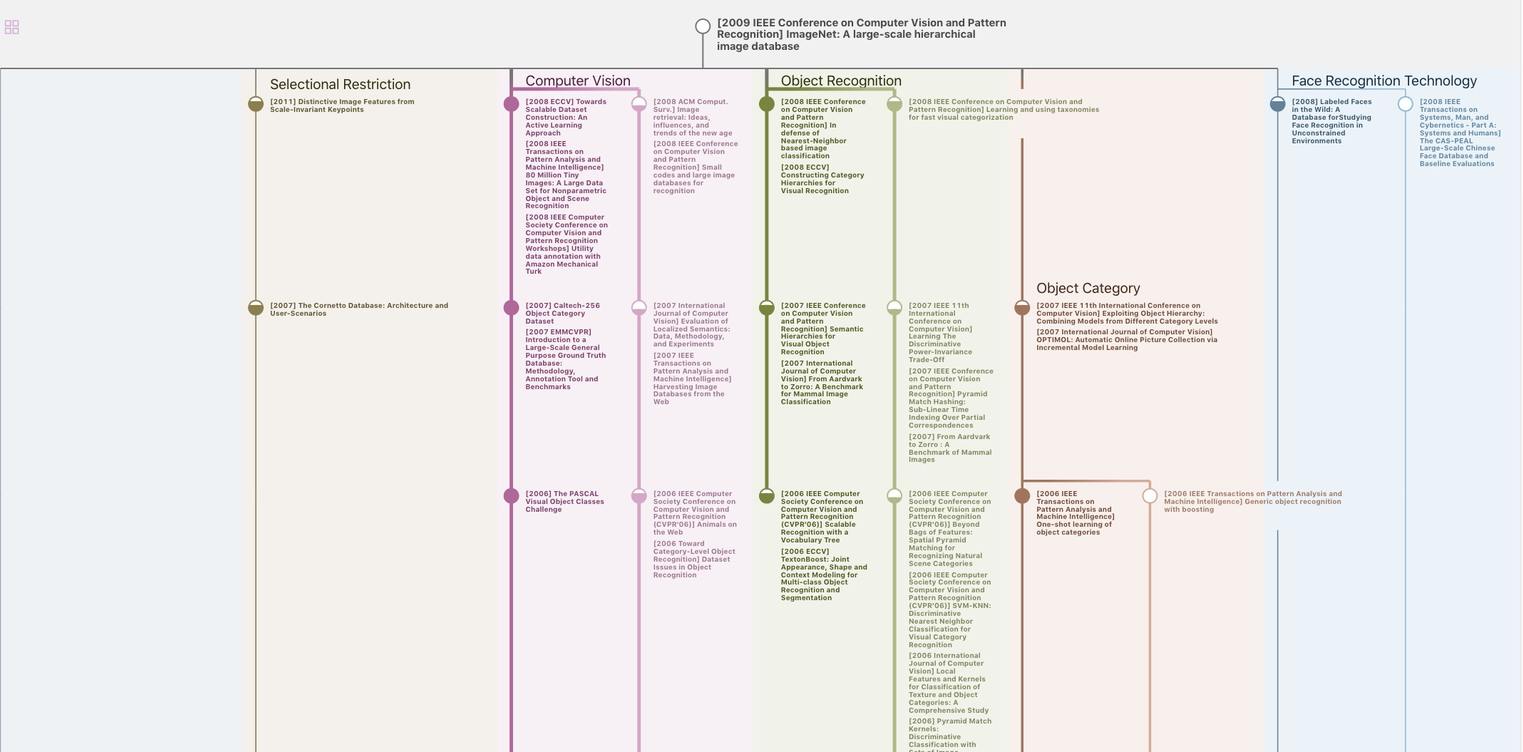
生成溯源树,研究论文发展脉络
Chat Paper
正在生成论文摘要