Trend Feature Consistency Guided Deep Learning Method for Minor Fault Diagnosis.
Entropy (Basel, Switzerland)(2023)
摘要
Deep learning can be applied in the field of fault diagnosis without an accurate mechanism model. However, the accurate diagnosis of minor faults using deep learning is limited by the training sample size. In the case that only a small number of noise-polluted samples is available, it is crucial to design a new learning mechanism for the training of deep neural networks to make it more powerful in feature representation. The new learning mechanism for deep neural networks model is accomplished by designing a new loss function such that accurate feature representation driven by consistency of trend features and accurate fault classification driven by consistency of fault direction both can be secured. In such a way, a more robust and more reliable fault diagnosis model using deep neural networks can be established to effectively discriminate those faults with equal or similar membership values of fault classifiers, which is unavailable for traditional methods. Validation for gearbox fault diagnosis shows that 100 training samples polluted with strong noise are adequate for the proposed method to successfully train deep neural networks to achieve satisfactory fault diagnosis accuracy, while more than 1500 training samples are required for traditional methods to achieve comparative fault diagnosis accuracy.
更多查看译文
关键词
deep learning,fault orientation consistency,minor fault,small sample size,trend feature consistency
AI 理解论文
溯源树
样例
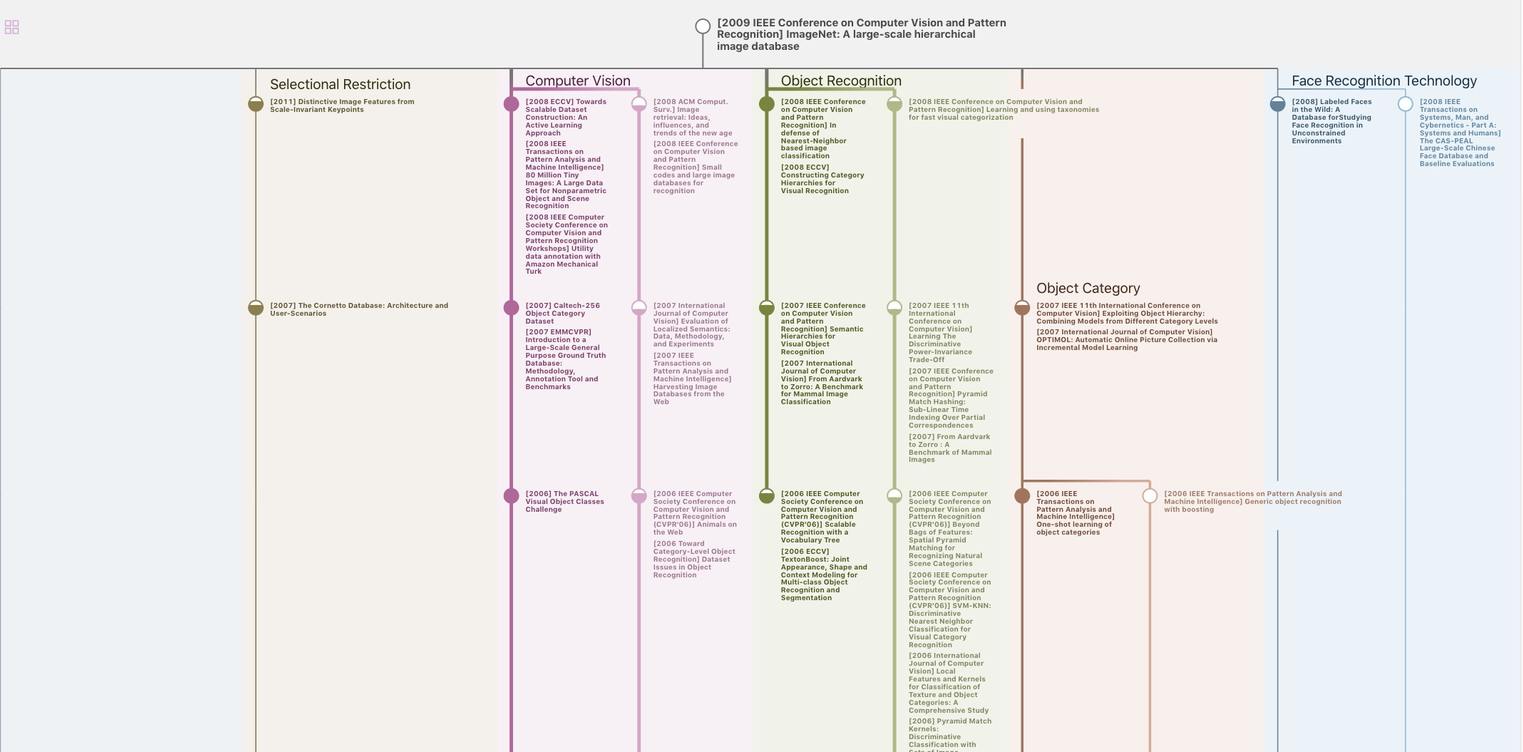
生成溯源树,研究论文发展脉络
Chat Paper
正在生成论文摘要