Rail Defect Detection Using Ultrasonic A-Scan Data and Deep Autoencoder
Transportation Research Record: Journal of the Transportation Research Board(2023)
摘要
Rail defects, especially transverse defects (TDs), can pose risks to safe and efficient railroad operations. Effective rail defect detection is critical for the prevention of broken rail-induced accidents and derailments. In this study, a deep autoencoder (DAE) rail defect detection framework is developed to process ultrasonic A-scan data collected by a roller search unit and to identify the presence of TDs in rail samples. An autoencoder is a semi-supervised learning algorithm that identifies observations in a dataset that significantly deviate from the remaining observations and can be used for rail defect detection. Ultrasonic A-scan signals collected from both pristine and damaged rail segments are analyzed, where the pristine dataset is used to train a DAE model. To improve the accuracy and sensitivity of defect detection, we optimize the architecture and hyperparameters of the DAE model. Moreover, we evaluate the performance of two features extracted from the DAE model through receiver operating characteristic curves and confusion matrix. The DAE features outperformed conventional knowledge-driven features in the accuracy and robustness of defect detection, especially with the presence of noise.
更多查看译文
关键词
rail,rail structures,track,defect,inspection
AI 理解论文
溯源树
样例
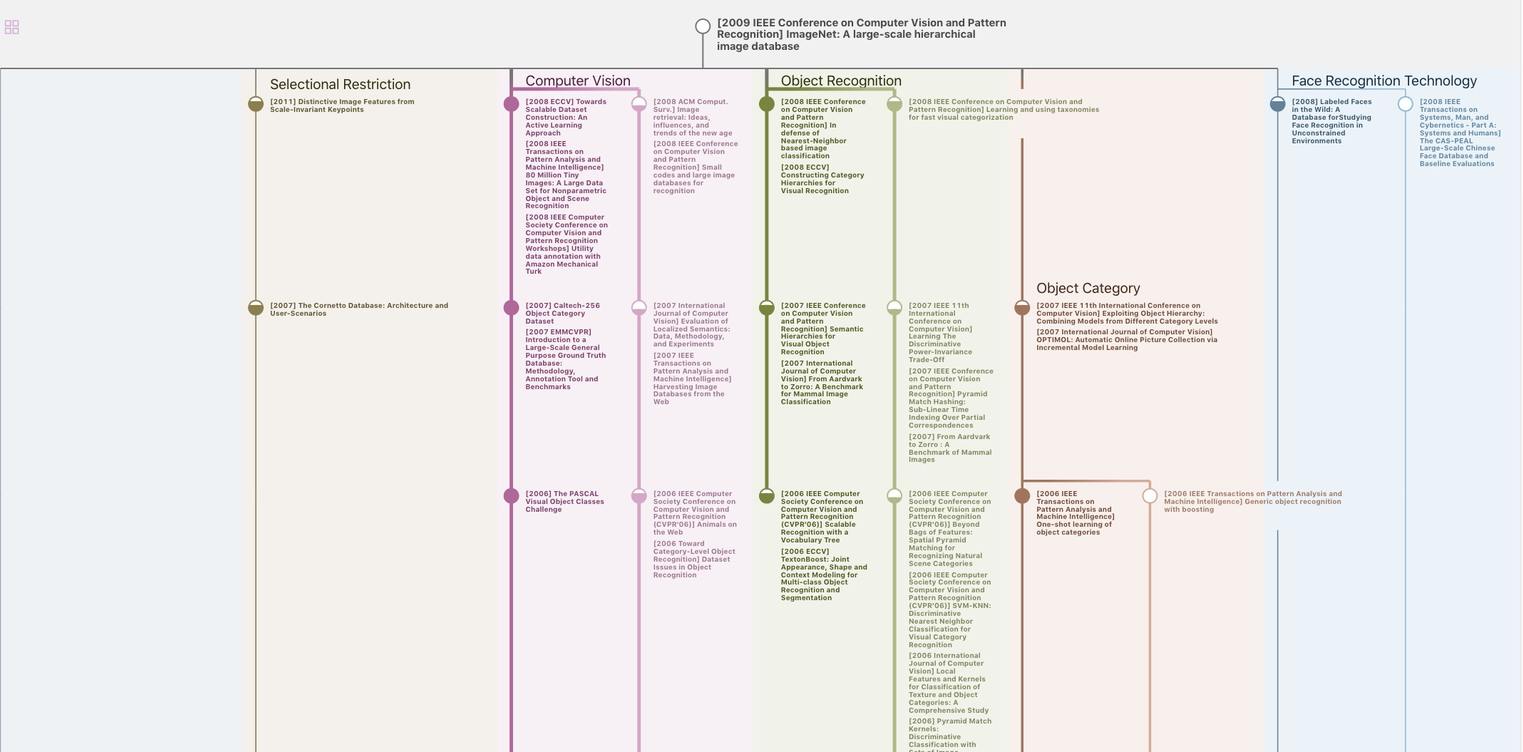
生成溯源树,研究论文发展脉络
Chat Paper
正在生成论文摘要