Cross-Guided Feature Fusion with Intra-Modality Reweighting for Multi-Spectral Pedestrian Detection.
ICPR(2022)
Abstract
Multi-spectral pedestrian detection has gained extensive attention over the past decade. To alleviate the problem of modality imbalance in the multi-spectral tasks, a novel cross-guided feature fusion network based on the auto-encoder framework is proposed using RGB-thermal image pairs as inputs. To obtain the complementary features, a cross-guided loss is designed, so that the output images are balanced with both modalities in an unsupervised manner. An intra-modality reweighting module is implemented to filter the redundant features before the fusion. Finally, YOLOv3 is chosen as the detector fed by the fused features. The proposed method is verified using the public KAIST and VOT-RGBT datasets. Experimental results demonstrate that the proposed method can outperform the state-of-the-art methods, the miss rate of pedestrian detection reaches 48.57% and 4.52% using KAIST and VOT-RGBT datasets, respectively.
MoreTranslated text
Key words
auto-encoder framework,complementary features,cross-guided loss,fused features,intra-modality reweighting module,modality imbalance,multispectral pedestrian detection,multispectral tasks,novel cross-guided feature fusion network,redundant features,RGB-thermal image pairs
AI Read Science
Must-Reading Tree
Example
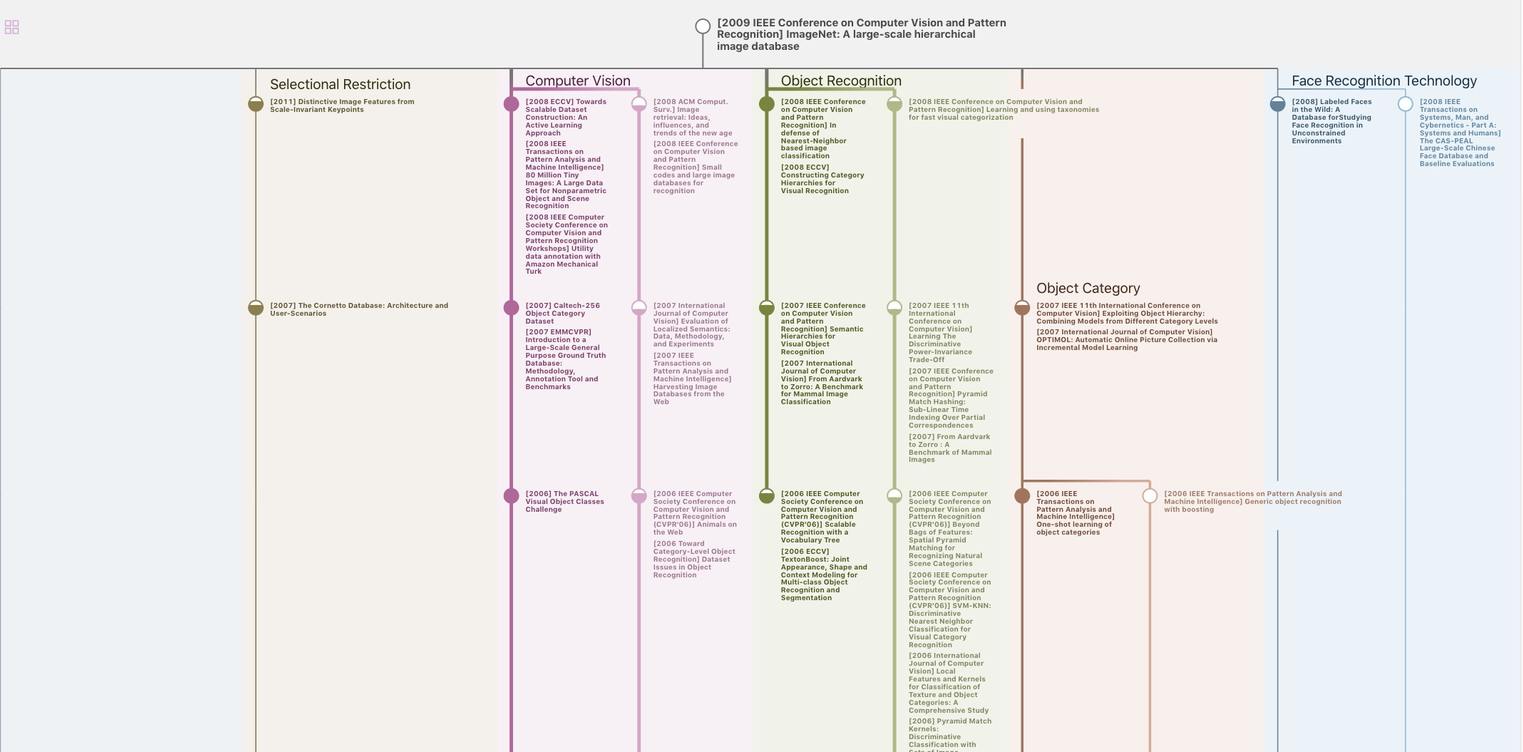
Generate MRT to find the research sequence of this paper
Chat Paper
Summary is being generated by the instructions you defined