Cross-Session Motor Imagery EEG Classification using Self-Supervised Contrastive Learning.
ICPR(2022)
摘要
Among various Brain-Computer Interfaces (BCI) categories, Electroencephalography (EEG) signals have advantages over their other counterparts, such as fNIRS and fMRI, for their ease of acquisition and affordable recording devices. Despite the ease of data recording, the labeling of the data needs expert knowledge, which is expensive. Also, the data collected from the same subjects in different sessions are prone to varying distribution, making the cross-session data classification more challenging. Supervised learning has been widely used for EEG signal analysis, but its performance is highly dependent on a vast amount of annotated data. Self-supervised learning (SSL) has been proven to be a highly effective technique that allows deep learning architectures to learn general features from unlabelled data without needing an extensive amount of labeled data. This paper proposes a self-supervised contrastive learning method of cross-session based EEG data for motor imagery classification. The pretext task of signal transformations has been used to train the network during the training phase to reduce the distance between the similar transformation pairs and maximize the distance between the dissimilar transformation pairs generated from the EEG signals. It helps the network learn generalized features of cross-session EEG signals with the help of representation learning and in the absence of labeled data. The diverse nature of cross-session EEG data leads the network toward learning more generalized features. The performance of the proposed model upon pretraining on the BCI-IV 2a dataset using self-supervised contrastive learning and the fine-tuning on the same dataset using supervised learning gives the average accuracy of 50.81% and performed significantly better than the compared supervised learning-based methods.
更多查看译文
关键词
annotated data,brain-computer interfaces,cross-session based EEG data,cross-session data classification,cross-session EEG signals,cross-session motor imagery EEG classification,data recording,deep learning architectures,EEG signal analysis,electroencephalography signals,generalized features,motor imagery classification,representation learning,self-supervised contrastive learning method,self-supervised learning,signal transformations,similar transformation pairs,supervised learning-based methods,unlabelled data
AI 理解论文
溯源树
样例
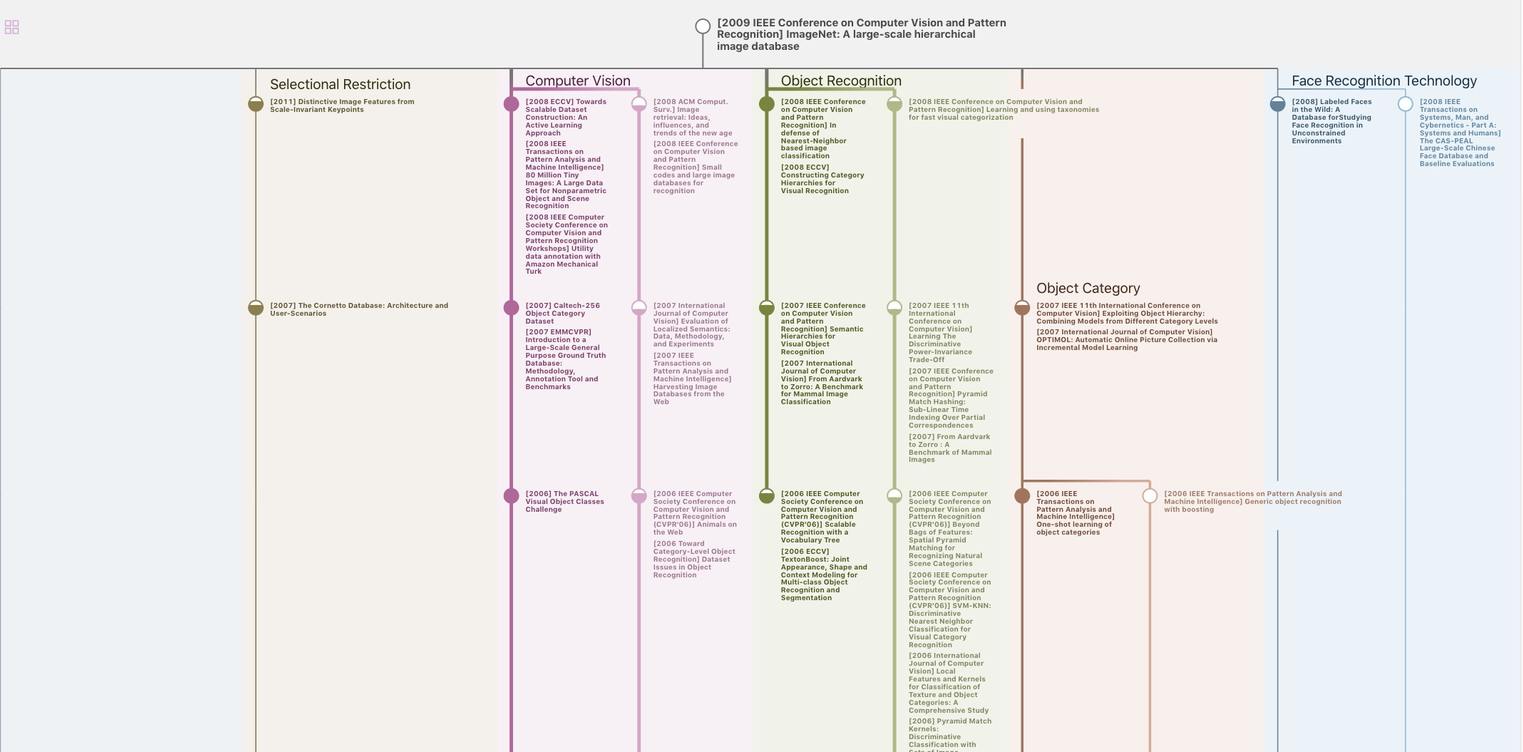
生成溯源树,研究论文发展脉络
Chat Paper
正在生成论文摘要