Online Feature Classification and Clustering for Transformer-based Visual Tracker.
ICPR(2022)
摘要
Compared with the current booming development of siamese tracking network, online optimization methods for tracking models still update parameters or features in pulses, which is non-real-time and on whole image level. In the past year, similarity measurement components derived from Transformer equiped on Siamese networks have obtained excellent performance in visual tracking task. Leveraging its element-wise attention mechanism, we implement a real-time feature update approach on coarse pixel level. We first construct a classification branch for quality control; and to further reduce the feature amount in online update process, we apply an incremental clustering method to minimize the repetitive contribution of similar features. The proposed method is evaluated on multiple datasets including OTB2015, NfS and GOT-10k. It exceeds the baseline methods on all 3 datasets and achieves competitive performance against the state-of-the-art networks.
更多查看译文
关键词
Visual Tracking,Online Updating,Transformer-based Tracker,Feature Clustering
AI 理解论文
溯源树
样例
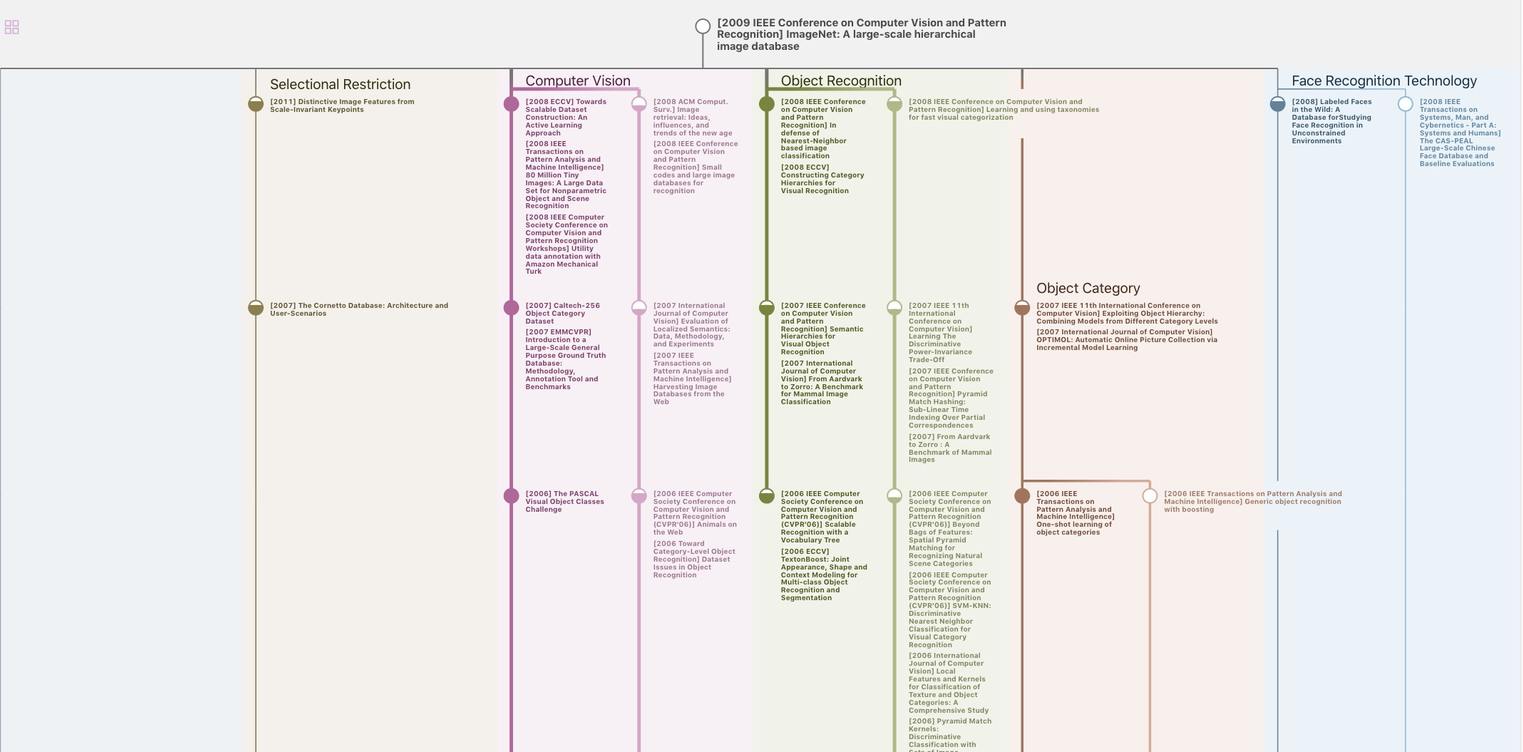
生成溯源树,研究论文发展脉络
Chat Paper
正在生成论文摘要