Kolmogorov-Smirnov and ROC curve metrics for binary classification performance assessment are equivalent
ICPR(2022)
摘要
Binary classification problems are very common in decision making. In decision support systems, classifiers which produce a propensity score for mapping the multidimensional space into a scalar give the human decider the power to control the operating point by just setting a threshold to separate the two classes. These classifiers, usually called rankers, have their discriminating power measured along the continuous range of the score by the Area Under the ROC curve (AUC_ROC) in most application fields. Only the domain of finances uses the single point metric maximum Kolmogorov-Smirnov (KS) distance. This paper presents the uncommon Area Under the KS curve (AUC_KS) for performance assessment and proves that AUC_ROC = 0.5+ AUC_KS. This equivalence allows for a simpler calculation of the AUC_ROC and brings about new perspectives for ROC averaging in ensembles of classifiers or n-fold cross-validation. The equivalence proof is geometrically inspired on rotating the KS curve counter-clockwise via a simple matrix transformation. Having the independent variable (normalized score) on the abscissa of the KS curve simplifies the calculation of the average AUC_KS and its errors in ensembles, thus yielding their ROC counterparts calculations by simple matrix multiplication. This research gives insights on probabilistic interpretations of classifiers assessment and integrates the existing body of knowledge of the information theoretical ROC approach with the proposed statistical approach based on the thoroughly known KS distribution.
更多查看译文
关键词
Binary classification performance metrics,Area Under the ROC curve,Kolmogorov-Smirnov distribution,Space transformation matrix
AI 理解论文
溯源树
样例
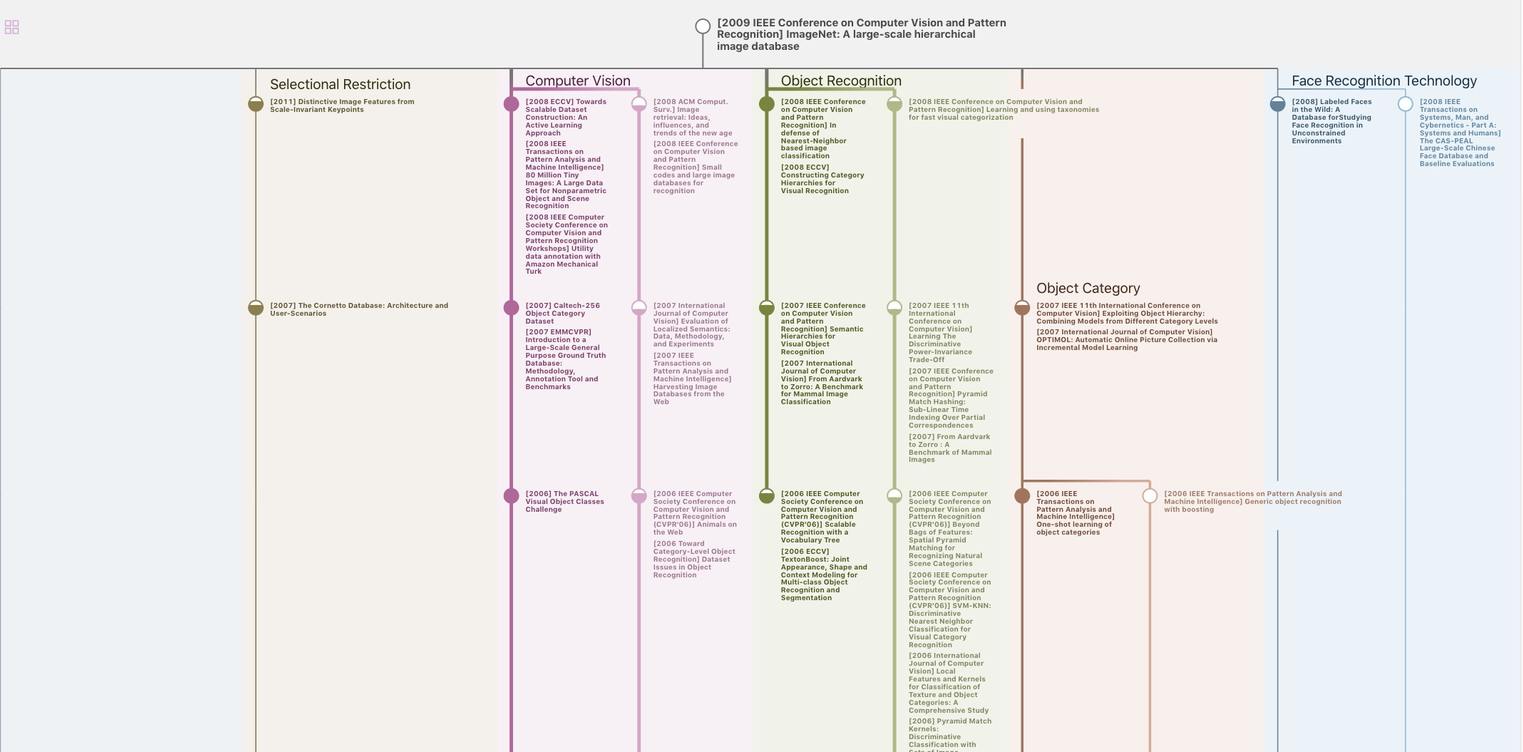
生成溯源树,研究论文发展脉络
Chat Paper
正在生成论文摘要