Adv-Cut Paste: Semantic adversarial class specific data augmentation technique for object detection
ICPR(2022)
摘要
Data augmentation has been a prevalent approach in improving the performance of deep learning models against slight variations in data. Adversarial learning is one such form of data augmentation. In this work, we aim to introduce a framework to generate harder examples for a specific object class and an adversarial attack for the object detection task. We have also presented our study on the effect of training against such generated harder examples and adversarial samples in object detection. We have applied this adversarial learning technique to a YOLOv3 model and due to the nature of the attack, we demonstrated a substantial improvement in average precision (AP) for a single class of the COCO dataset. As per the literature, we are the first to introduce this kind of class-specific data augmentation strategy in object detection. With our approach, we have shown an improvement of 23.34% in AP for Cat class and 3.1% on overall mAP of YOLOv3 model on clean validation data, while 43.5% improvement in AP for the Cat class on the composite images with class-specific adversarial samples.
更多查看译文
关键词
semantic adversarial class,specific data augmentation technique,object detection,adv-cut
AI 理解论文
溯源树
样例
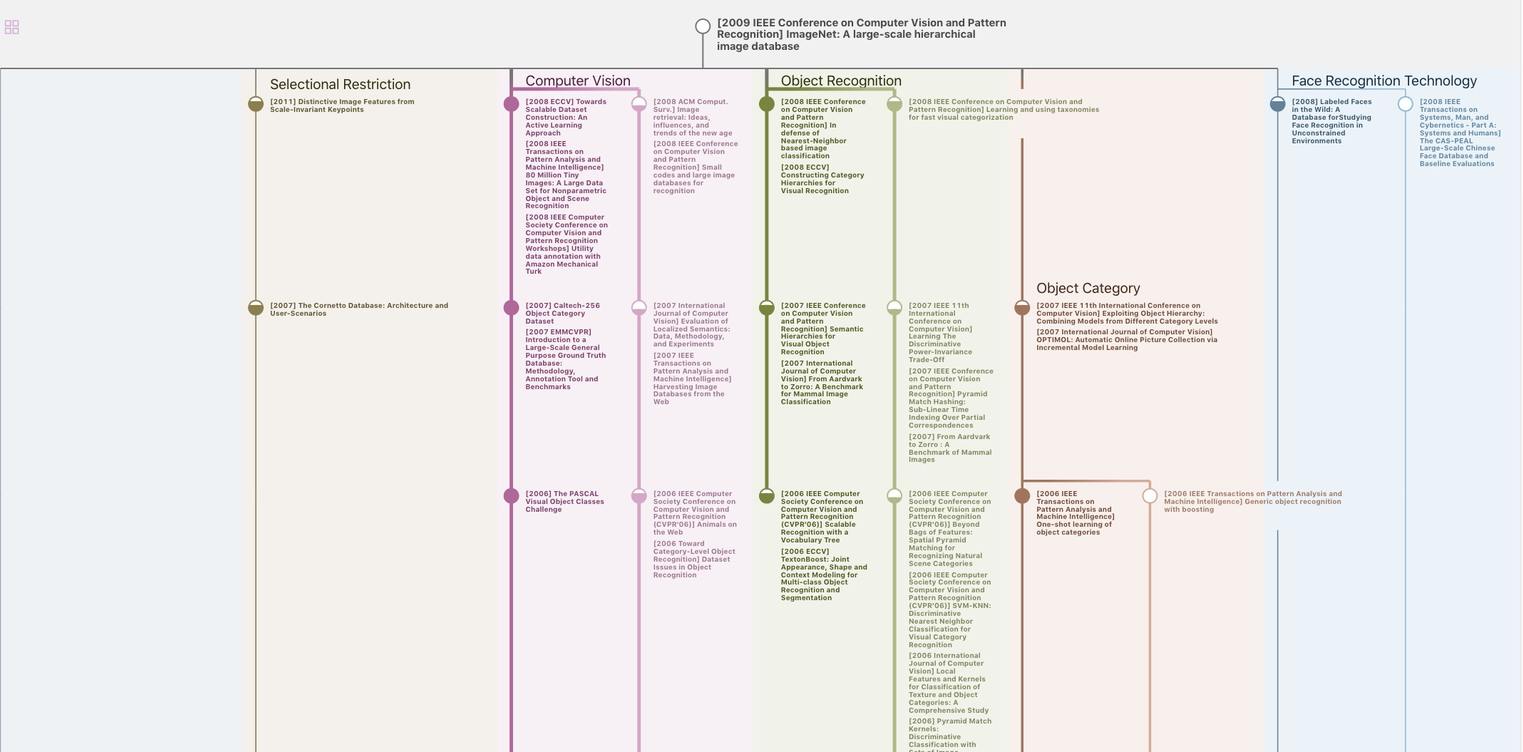
生成溯源树,研究论文发展脉络
Chat Paper
正在生成论文摘要