Lung Nodule Malignancy Subtype Discovery with Semantic Learning.
ICPR(2022)
摘要
Computer-aided diagnosis (CAD) systems have been widely used as second readers in lung cancer diagnosis. However, lung cancer heterogeneity and lack of using human annotated semantic characteristics are significant obstacles to an accurate and explainable CAD outcome. We propose a novel CAD scheme that characterizes lung nodule malignancy subtypes semantically and classifies nodule malignancy through a semantic learning process. We built and evaluated our method on a publicly available dataset, Lung Image Database Consortium (LIDC). We discovered and characterized two malignant nodule subtypes and two benign subtypes. In addition, we achieved a significantly improved malignancy classification result by incorporating the semantic learning process when compared with a result without using the semantic learning. This study suggests that future CAD systems should include disease heterogeneity as a model input to refine the model explainability; our classification result provides case-base evidence that learning image semantic explanations is valuable for improving CAD accuracy. While we tested our approach on one dataset, the proposed approach is applicable to other CAD tasks if semantic annotations are available.
更多查看译文
关键词
lung,discovery,malignancy,learning
AI 理解论文
溯源树
样例
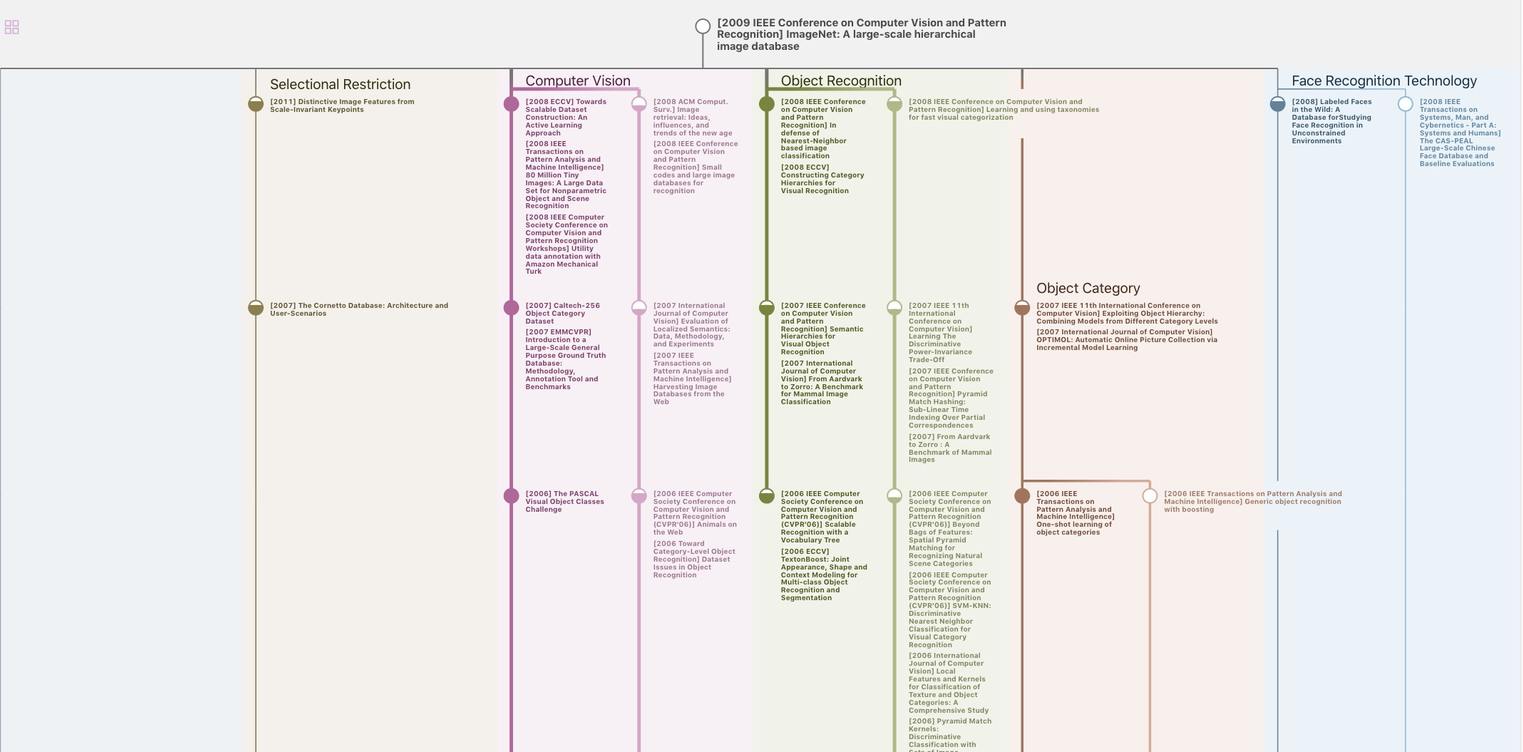
生成溯源树,研究论文发展脉络
Chat Paper
正在生成论文摘要