CycleResume: A Cycle Learning Framework with Hybrid Attention for Fine-Grained Talent-Job Fit.
CICAI (3)(2022)
摘要
Online recruitment platforms are becoming powerful tools for job hunters to look for jobs and for companies to search for outstanding candidates. To liberate the workforce for resume assessment, there is an increasing attention on developing learning-based systems for automatic talent-job fit. Existing approaches suffer from several drawbacks, such as simply ignoring the interactions between talent's skills and experiences and only utilizing the final output representations of resume and job-post for matching. To address these issues, we propose a new deep cycle learning-based framework for talent-job fit. In particular, we first propose a noise-incorporated co-attention mechanism to measure resume consistency in a fine-grained manner. Then a multi-scale CNN is utilized for job representation learning, and the matching score between resume and job is measured via multi-scale attention. To evaluate the effectiveness of the proposed approach, we conduct extensive experiments on a self-collected resume-job dataset. The results show that our method achieves state-of-the-art performance, and we demonstrate that the proposed modules can be utilized as off-the-shelf blocks for similar application tasks.
更多查看译文
关键词
Talent-job fit, Cycle learning, Hybrid attention
AI 理解论文
溯源树
样例
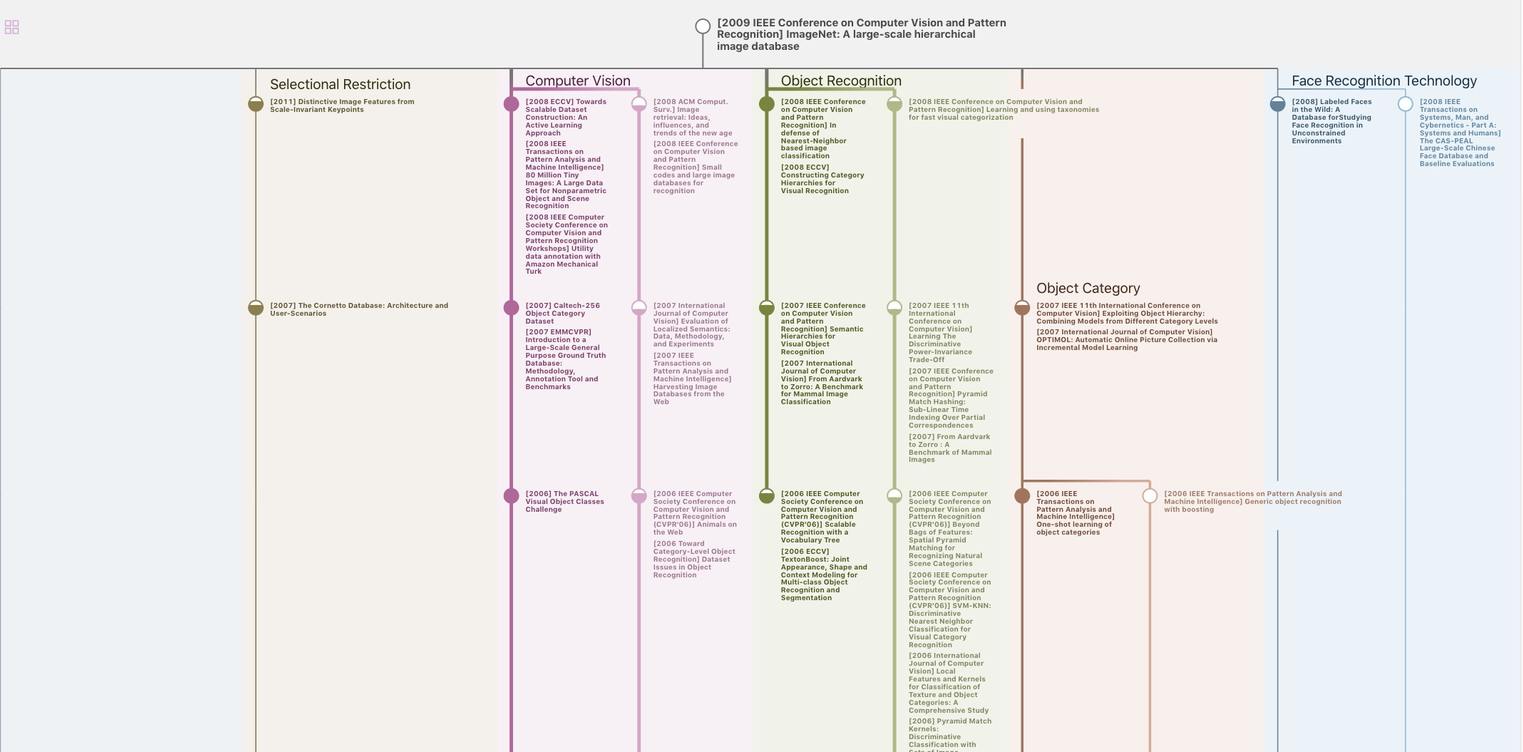
生成溯源树,研究论文发展脉络
Chat Paper
正在生成论文摘要