"Earlier" Warning Systems: Making the Most out of the First Signs of Student Underperformance.
2022 IEEE FRONTIERS IN EDUCATION CONFERENCE, FIE(2022)
关键词
blended learning,early warning systems,learning analytics,learning management systems,machine learning
AI 理解论文
溯源树
样例
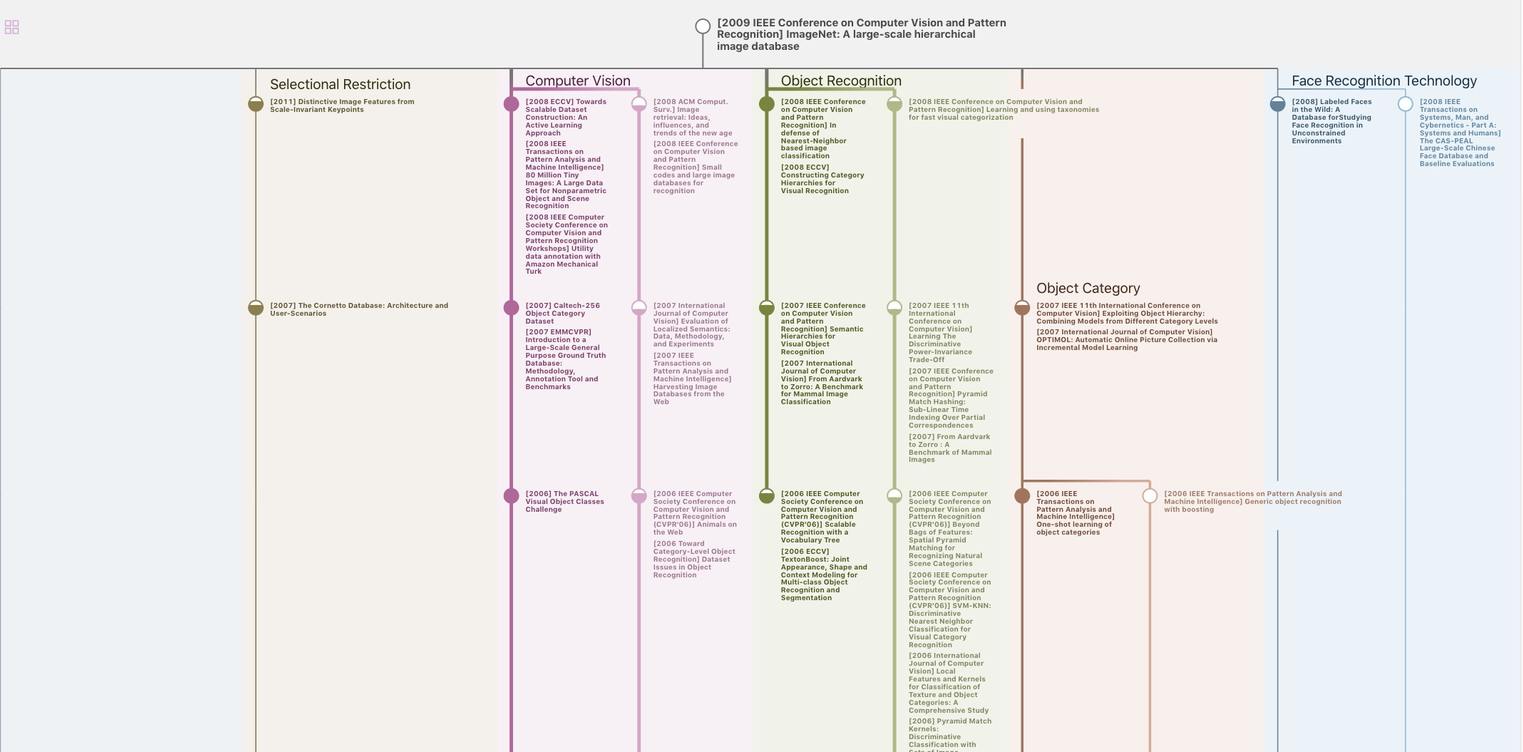
生成溯源树,研究论文发展脉络
Chat Paper
正在生成论文摘要