Feature Extraction for a Genetic Programming-Based Brain-Computer Interface.
BRACIS (1)(2022)
摘要
Brain-Computer Interfaces (BCI) open a two-way communication channel between a computer and the brain: while the brain can control the computer, the computer can induce changes in the brain through feedback. This mechanism is used in post-stroke motor rehabilitation, in which a BCI provides feedback by classifying signals collected from a patient's brain. Single Feature Genetic Programming (SFGP) can create classifiers for these signals. However, the Genetic Programming (GP) step in SFGP requires a set of extracted features to generate its model. To the best of our knowledge, the LogPower function is the only initial feature extraction function used in SFGP. Nevertheless, other functions can improve the quality of the generated classifiers. Thus, we analyze new initial feature extraction functions for GP in SFGP. We test the Common Spatial Patterns, Nonlinear Energy, Average Power Spectral Density, and Curve Length methods on two datasets suitable for post-stroke rehabilitation training. The results obtained show that the analyzed functions outperform LogPower in all our experiments, with a kappa value up to 25.20% better. We further test the proposed methods on a third dataset, created with low-cost equipment. In this case, we show that the Average Power Spectral Density function outperforms LogPower by 11.39% when three electrodes are used. Thus, we demonstrate that the new approach can be used with low-cost equipment and a small number of electrodes, reducing the financial costs of treatment and improving patients' comfort.
更多查看译文
关键词
Brain-machine interface, Common spatial pattern, Stroke rehabilitation, Power spectral density
AI 理解论文
溯源树
样例
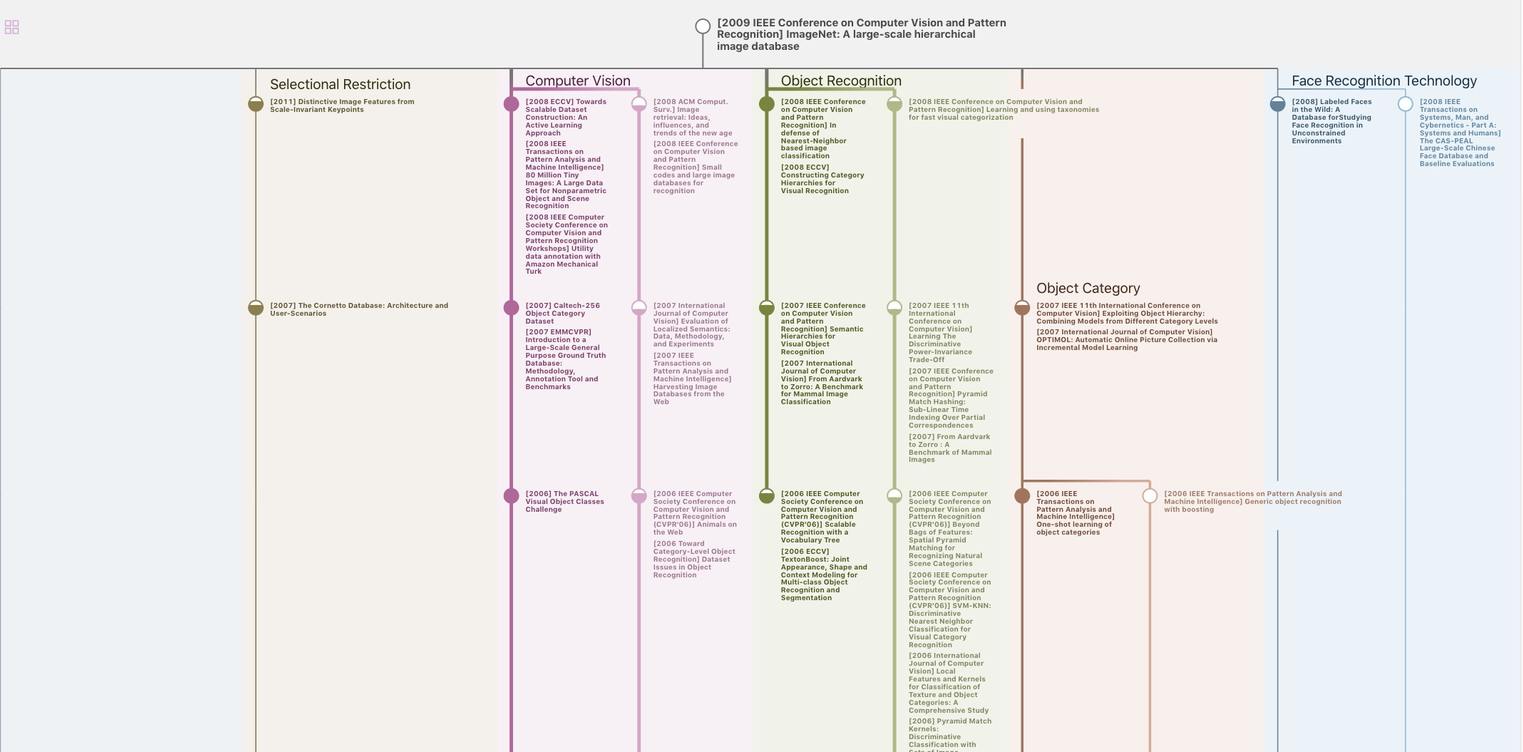
生成溯源树,研究论文发展脉络
Chat Paper
正在生成论文摘要