Discretization-Agnostic Deep Self-Supervised 3D Surface Parameterization
SIGGRAPH ASIA 2022 TECHNICAL COMMUNICATIONS PROCEEDINGS, SIGGRAPH 2022(2022)
Abstract
We present a novel self-supervised framework for learning the discretization-agnostic surface parameterization of arbitrary 3D objects with both bounded and unbounded surfaces. Our framework leverages diffusion-enabled global-to-local shape context for each vertex first to partition the unbounded surface into multiple patches using the proposed self-supervised PatchNet and subsequently perform independent UV parameterization of these patches by learning forward and backward UV mapping for individual patches. Thus, our framework enables learning a discretization-agnostic parameterization at a lower resolution and then directly inferring the parameterization for a higher-resolution mesh without retraining. We evaluate our framework on multiple 3D objects from the publicly available SHREC [Lian et al. 2011] dataset and report superior/faster UV parameterization over conventional methods.
MoreTranslated text
Key words
UV parameterization,texture mapping,neural network,self-supervised learning,surface parameterization
AI Read Science
Must-Reading Tree
Example
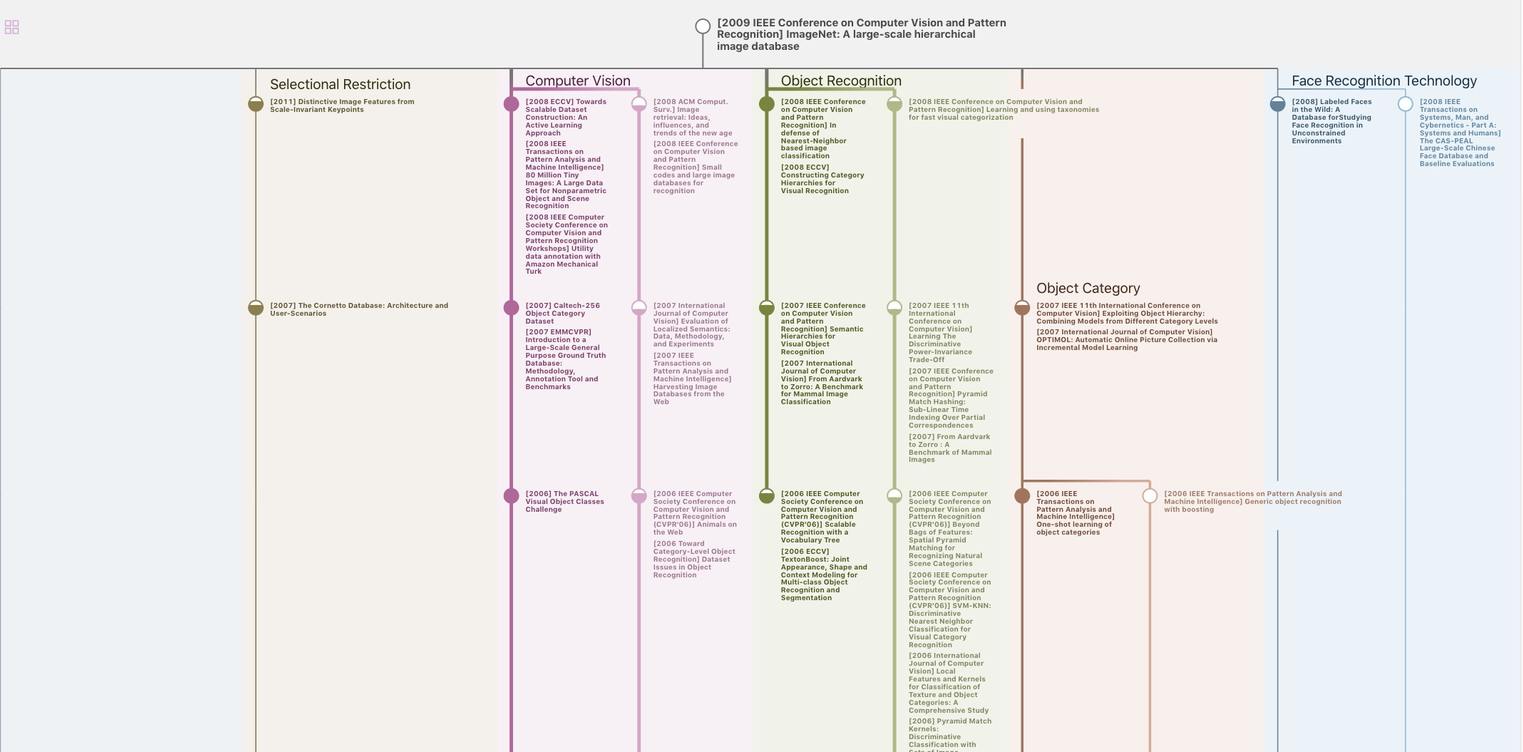
Generate MRT to find the research sequence of this paper
Chat Paper
Summary is being generated by the instructions you defined