Multi-objective Optimization Based Feature Selection Using Correlation.
ADMA (2)(2022)
摘要
The optimal feature selection (FS) problem is widely targeted in the field of machine learning (ML). There are several ways to select the best features when the dataset dimension is small. However, when the dataset and number of features tend to increase, the solution becomes unrealistic as we need to evaluate every subset performance with the model. Various existing heuristics are partially useful as they portray premature convergence and exponential or high computational complexity. To solve this issue, evolutionary approaches-based FS has been extensively used in obtaining the optimal subset of features while maintaining the accuracy of the model. This paper proposes an efficient evolutionary-based multi-objective feature selection approach with a correlation coefficient filter method called Multi-Objective Optimization based Feature Selection (MOOFS). We introduce a two-stage process to select the best optimal features. In the first stage, a subset of features is randomly selected, and then a novel mutual correlation coefficient technique is used to get the important and relevant subset of features. The proposed MOOFS is experimented on several datasets and compared with the classical approach to demonstrate its efficiency.
更多查看译文
关键词
selection,optimization,feature,correlation,multi-objective
AI 理解论文
溯源树
样例
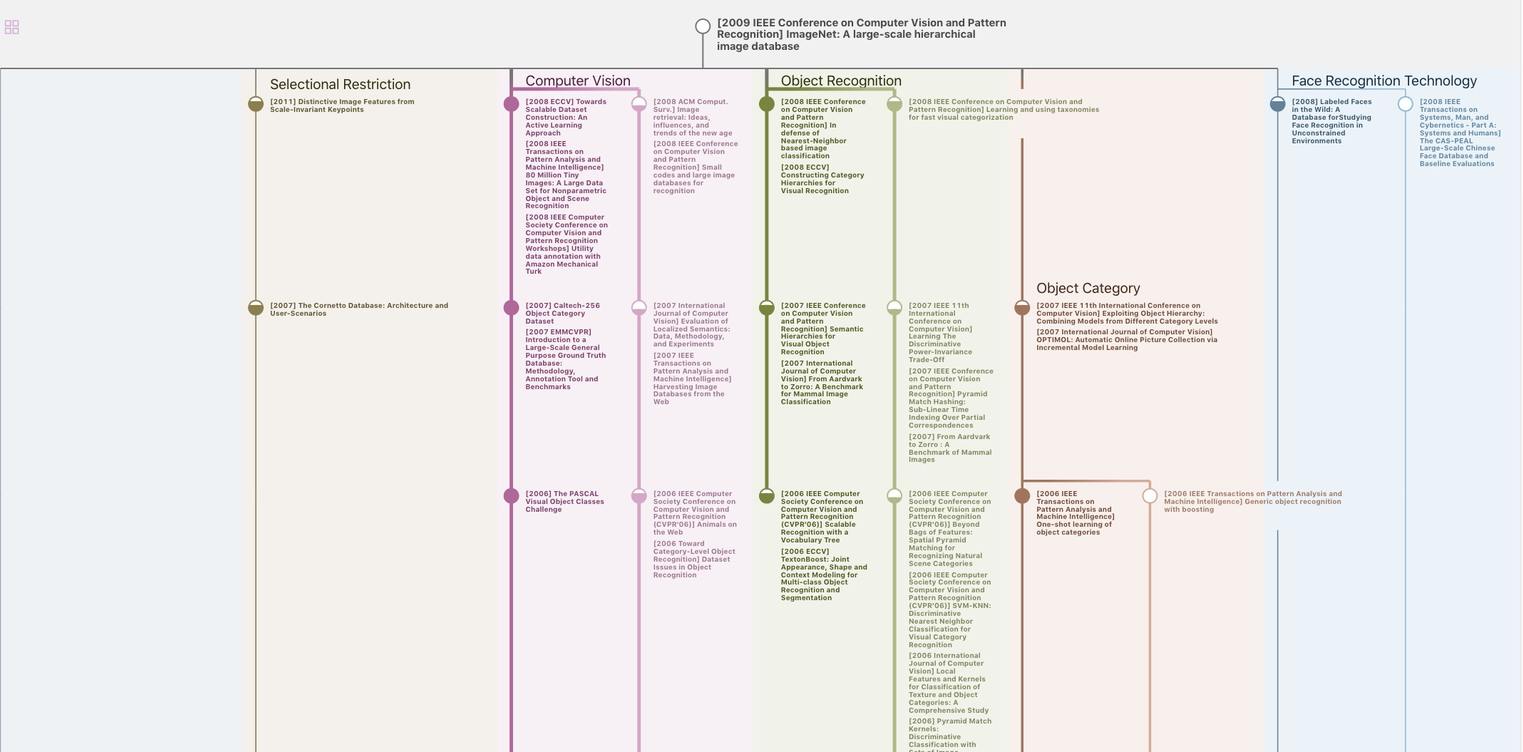
生成溯源树,研究论文发展脉络
Chat Paper
正在生成论文摘要