PR-MVI: Efficient Missing Value Imputation over Data Streams by Distance Likelihood.
iiWAS(2022)
摘要
Predicting missing attribute values in data streams is useful in boosting the accuracies of analytical results in many applications. Many algorithms (i.e., Distance Likelihood Maximization (DLM)) have been proposed for permanently-stored data. They can be used to handle data streams, but the performance is bad when the data streams have a different distribution from the training data. Some works (i.e., Autoregressive Integrated Moving Average (ARIMA)) can deal with data streams, but they cannot handle categorical data. This paper proposes Past and Recent neighboring approaches for Missed attribute Value Imputation by distance likelihood, called "PR-MVI", over data streams. PR-MVI learns from both past training data and the set of the most recent complete records. It can handle both numerical and categorical data streams that have similar and/or different distribution from that of the past training data. Extensive experiments have shown that PR-MVI can better predict the missed attribute values over data streams than other existing approaches.
更多查看译文
关键词
Data cleansing, Data streams, Missed attribute values
AI 理解论文
溯源树
样例
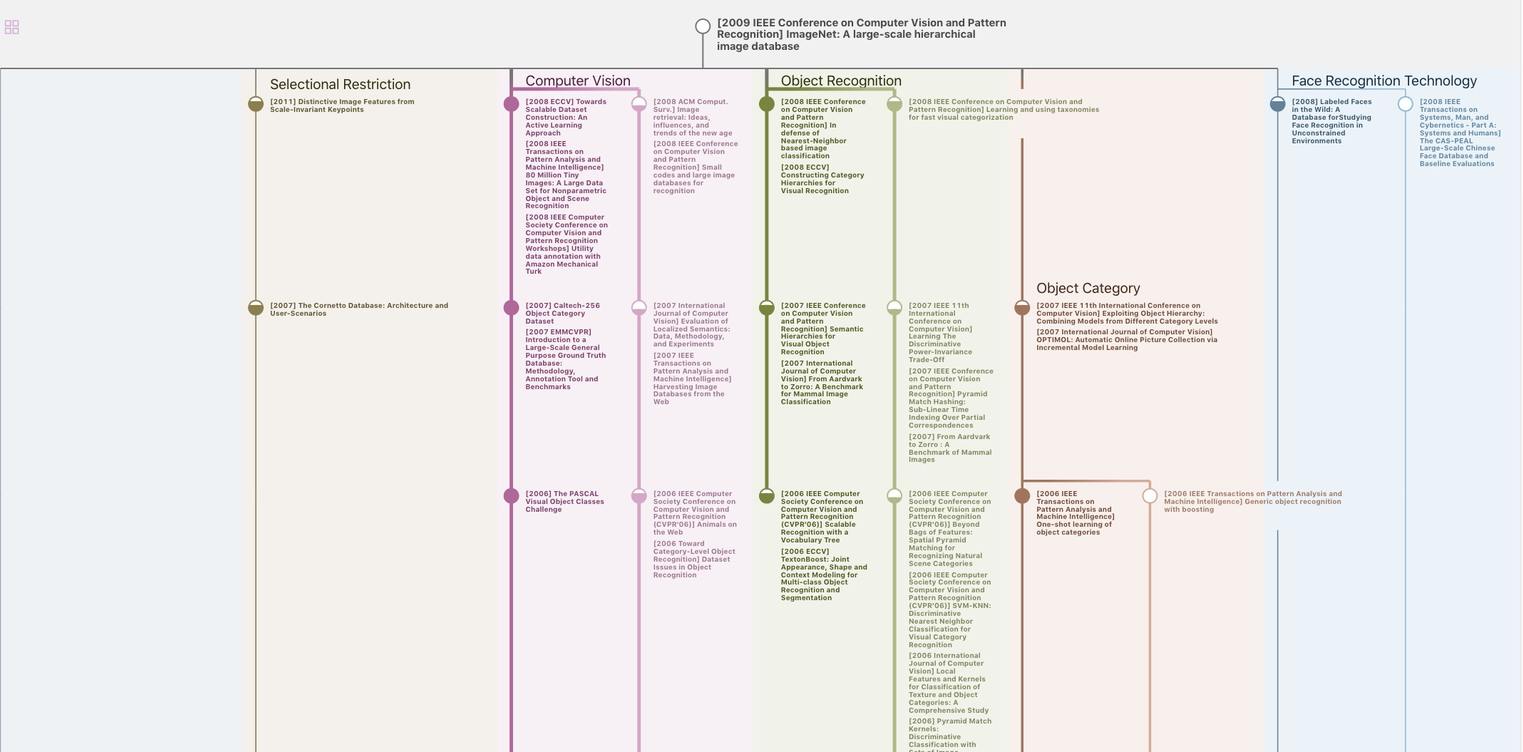
生成溯源树,研究论文发展脉络
Chat Paper
正在生成论文摘要